Improving Writing Feedback: GPT-4’s Role in Assessing Young Students’ Revisions
Researchers from the University of Pittsburgh and RAND Corporation explored GPT-4’s ability to evaluate young students’ essay revisions, finding it moderately effective but challenged by complex tasks and lacking developmental context. The study highlights the need for tailored AI scoring models and hybrid systems combining AI with human expertise for better educational support.
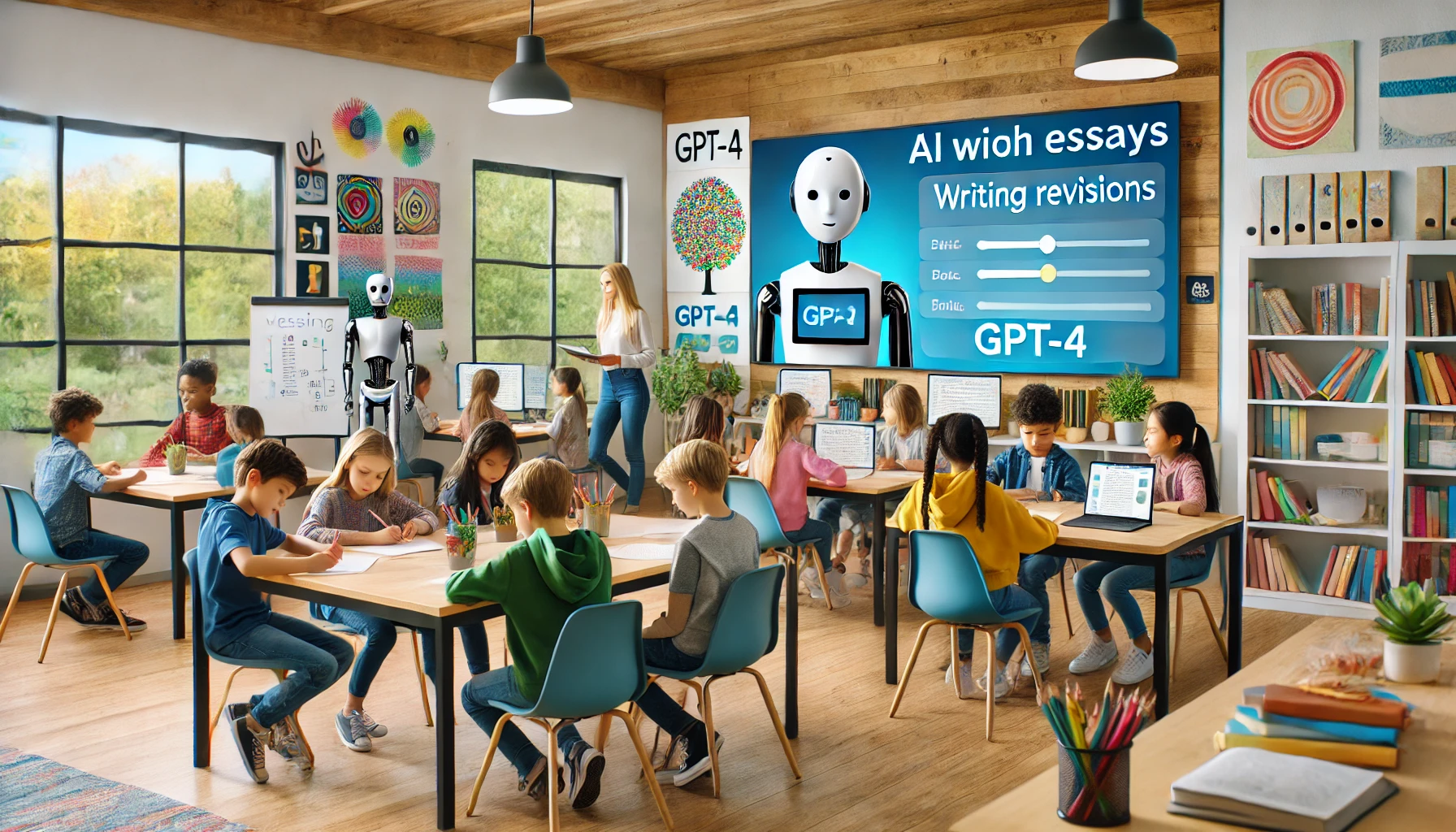
Researchers from the University of Pittsburgh and the RAND Corporation have explored the application of GPT-4 to assess young students’ essay revisions, addressing a critical gap in Automated Writing Evaluation (AWE) systems. These systems traditionally focus on final essay quality, overlooking the revision process a vital aspect of developing strong writing skills. Revision is known to be challenging for students, particularly younger ones, as they often struggle to implement feedback effectively. Studies indicate that many students fail to align their revisions with the feedback provided, leading to missed opportunities for meaningful improvement. This research emphasizes the importance of evaluating revision quality to offer targeted insights that help both students and educators refine their approaches to writing instruction.
Leveraging GPT-4 for Holistic Revision Scoring
The researchers employed GPT-4 to evaluate student essay revisions using a detailed rubric that classified revisions into four levels: no attempt, attempted but not improved, slightly improved, and substantively improved. The dataset comprised 600 pairs of essays from fifth- and sixth-grade students who participated in a study using the eRevise system. This system provides tailored feedback to students based on their initial essay drafts, focusing on completeness and specificity of evidence, elaboration, and connections to arguments. The study sought to determine how accurately GPT-4 could assess these revisions compared to human raters and whether its performance varied based on students' writing proficiency levels.
Three prompting strategies were tested: zero-shot prompting, one-shot Chain-of-Thought (CoT) prompting with human rationale examples, and one-shot CoT prompting with intermediate reasoning steps. The baseline zero-shot model achieved a moderate agreement with human raters, with an exact match rate of 52% and a Quadratic Weighted Kappa (QWK) score of 0.60. The one-shot CoT approach, which included examples and rationales, slightly improved the exact agreement to 54.5%, though the QWK remained constant. However, introducing intermediate reasoning steps in the one-shot CoT prompt led to a significant decline in performance, with agreement dropping to 36.33% and the QWK score to 0.46. These results suggest that while GPT-4 performs well when using clear and detailed rubrics, its effectiveness decreases when tasked with more complex reasoning.
Challenges in Evaluating Complex Writing Tasks
GPT-4’s accuracy varied significantly depending on the complexity of the revisions it was evaluating. The model excelled in simpler tasks, such as assessing whether students added evidence to their essays, achieving its highest agreement rates with human raters for essays at the lowest proficiency level. These tasks required straightforward feedback and revisions, such as adding more evidence, resulting in agreement rates of up to 65.93% and a QWK of 0.77. However, for mid-level essays requiring more nuanced revisions like elaboration and explanation of evidence, GPT-4’s performance declined. This highlights a limitation in the model’s ability to handle multifaceted writing tasks that involve synthesizing complex feedback.
An interesting trend emerged where GPT-4 consistently assigned lower scores to revisions compared to human raters, particularly for those rated as high-quality by human evaluators. Human raters often accounted for developmental appropriateness, acknowledging incremental improvements as significant within the context of the students’ age and writing abilities. GPT-4, lacking this developmental perspective, applied stricter standards for what constituted substantive improvement, often underestimating students’ efforts. This discrepancy highlights the need for AI systems to integrate contextual understanding and adapt scoring thresholds to align with students’ developmental stages.
Insights for Improving Automated Scoring Models
The study underscores the potential of GPT-4 and similar large language models for advancing formative assessment in education, but it also reveals important areas for enhancement. While detailed rubrics helped the model achieve moderate alignment with human evaluations, the marginal gains from CoT prompting indicate that more sophisticated strategies may be required. The researchers propose exploring alternative prompting methods, such as Tree-of-Thoughts, which could enable GPT-4 to better emulate human reasoning by systematically evaluating multiple aspects of revisions. Additionally, tailoring AI models to consider developmental appropriateness and recognizing students’ efforts could improve scoring accuracy and fairness.
Further, the study emphasizes the importance of combining AI capabilities with human expertise. While GPT-4 showed promise in assessing specific types of revisions, human raters provided a nuanced understanding of the context, effort, and age-appropriate expectations. This suggests that hybrid systems integrating AI-driven scalability with human judgment could offer the most effective solutions for educational applications.
Implications for Writing Instruction and Technology
This research highlights the value of focusing on the revision process, an often-overlooked aspect of writing instruction, to foster meaningful improvements in student writing. By identifying patterns and providing detailed feedback on revisions, educators can better support students in developing the skills needed for effective writing. The findings also underline the importance of designing AWE systems that move beyond surface-level assessments to address the complexities of revision.
Although GPT-4 demonstrated potential as a tool for evaluating revision quality, its limitations call for ongoing refinements in both AI technology and educational practice. Developing AI systems attuned to students' developmental needs and capable of handling complex writing tasks will be essential for creating equitable and impactful educational technologies. This study contributes to the broader conversation about the role of AI in education, offering actionable insights into how technology can complement human expertise to improve learning outcomes. Emphasizing the importance of revisions points to a path for enhancing teaching practices and the tools supporting them.
- FIRST PUBLISHED IN:
- Devdiscourse