AI's Uneven Impact: How Automation is Reshaping Regional Employment in the U.S
The study examines how AI adoption across U.S. regions from 2010 to 2021 led to significant declines in employment, particularly in manufacturing, low-skill services, and among middle-skill workers, younger and older individuals, and men. The findings underscore the uneven labor market impact of AI and highlight the need for policies to mitigate its adverse effects.
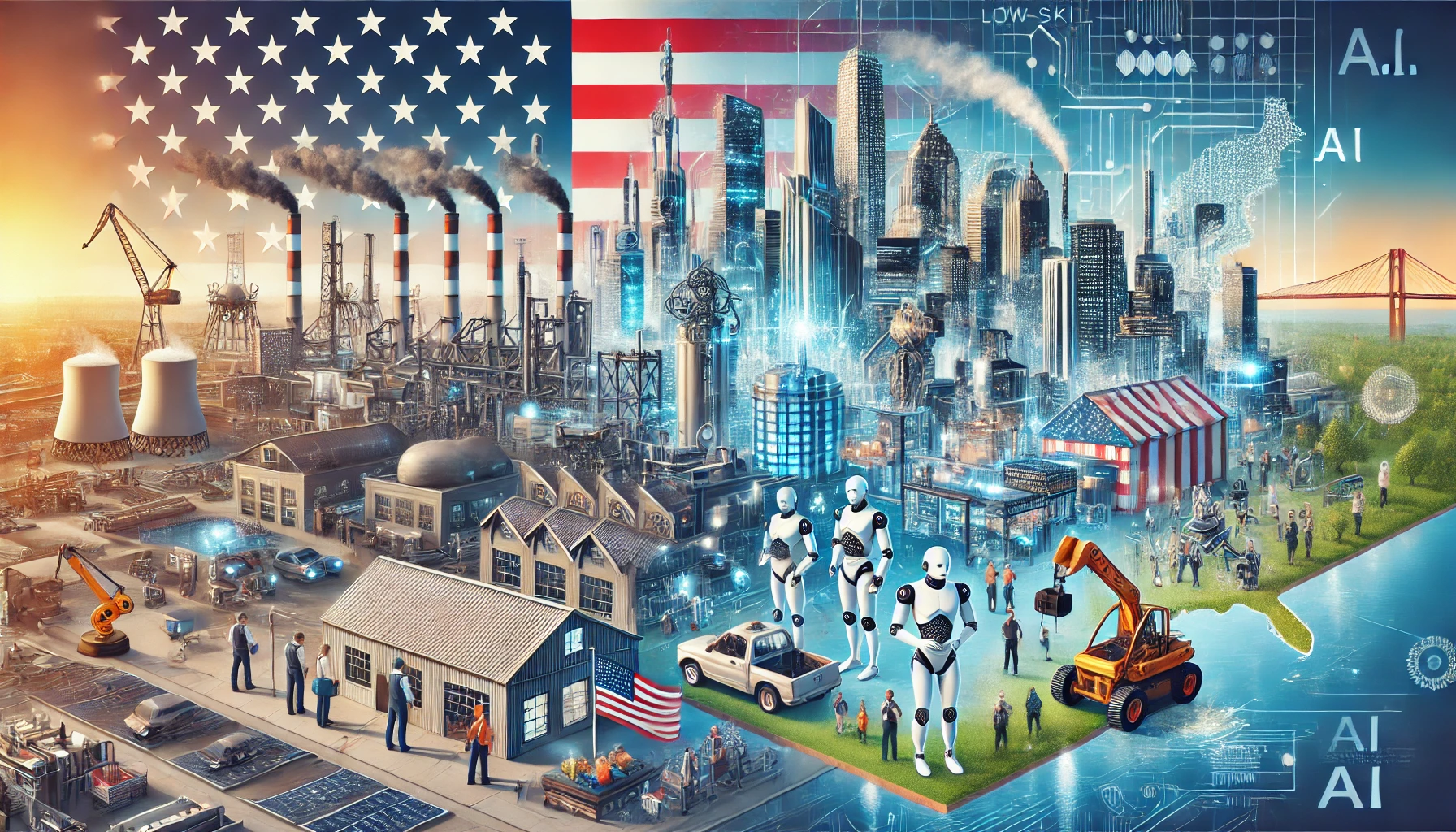
The paper "The Labor Market Impact of Artificial Intelligence: Evidence from US Regions" by Yueling Huang, published by the International Monetary Fund (IMF) Research Department, investigates how artificial intelligence (AI) has influenced employment in different regions across the United States from 2010 to 2021. This research leverages a shift-share approach, which analyzes variations in AI adoption in U.S. commuting zones to study the effects of AI on the employment-to-population ratio. The findings reveal a significant decline in employment in areas with high AI adoption, particularly in the manufacturing and low-skill service sectors. This negative impact was felt primarily by middle-skill workers, individuals in non-STEM occupations, and those at the extremes of the age distribution, particularly younger and older workers. Additionally, the study found that men were more adversely affected by AI-induced job losses than women, underscoring the uneven labor market impact across different demographic groups.
Tackling the Challenges of AI Data and Endogeneity
One of the main challenges Huang faced was the lack of direct data on AI adoption at the local level, as well as issues related to the endogeneity of AI exposure. In response, Huang constructed a measure of AI exposure for U.S. commuting zones by combining data on local employment shares with national industry-level AI adoption data sourced from the Annual Business Survey. To address concerns of endogeneity such as AI adoption being influenced by local demand shocks or previous technological waves Huang used an instrumental variable approach. Specifically, the study employed local employment data from 1990 combined with industry-level AI adoption data from the European Union. This method aimed to account for global technological advances while isolating U.S.-specific factors, providing a more accurate picture of how AI affected local labor markets.
Regional Employment Declines in AI-Exposed Areas
The analysis showed that commuting zones with higher AI exposure saw a more pronounced decline in employment between 2010 and 2021. A one standard deviation increase in AI exposure led to a nearly 1% reduction in the employment-to-population ratio. Furthermore, regions at the 75th percentile of AI adoption experienced a 1.25 percentage point greater decline in employment compared to those at the 25th percentile. These effects were not evenly distributed across the workforce. The most substantial employment declines were concentrated in sectors such as manufacturing and low-skill services, where tasks are more likely to be automated by AI technologies like machine learning, machine vision, and automated-guided vehicles. Workers in middle-skill jobs—those that typically require some level of routine tasks—were hit harder, continuing the trend of job polarization seen in previous decades due to automation and offshoring. Middle-skill jobs, which were already vulnerable to technological disruptions, are increasingly being replaced by AI, leaving many workers without viable alternatives.
Impact Across Age and Gender Groups
In terms of demographic impact, the study revealed that individuals at both ends of the age spectrum—particularly those aged 16-25 and over 46 were disproportionately affected by AI-driven job losses. Younger workers, many of whom are less educated or in the process of acquiring skills, were more likely to be displaced from entry-level, lower-skill jobs, while older workers struggled to adapt to the rapid pace of technological change. Older workers also face higher opportunity costs when switching careers due to the specific skills they have accumulated over their working lives, making them less mobile in the labor market. Men, in particular, bore the brunt of these job losses, reflecting the gendered nature of AI’s impact on labor. Although women are more likely to be employed in occupations exposed to AI, these jobs tend to be more complementary to AI technologies, providing some level of insulation from the negative employment effects that men experienced.
Policy Implications for Managing AI's Uneven Impact
Huang’s findings highlight the complex and uneven nature of AI’s impact on employment, suggesting that AI adoption does not benefit all sectors or demographic groups equally. These results echo past labor market disruptions caused by automation, robotization, and trade liberalization, where certain workers and industries were disproportionately affected. For policymakers, the study emphasizes the importance of addressing the unequal distributional consequences of AI adoption. Social safety nets, job retraining programs, and policies aimed at enhancing labor mobility will be essential to mitigate the adverse effects on the most vulnerable workers, particularly those in declining industries or at risk of technological displacement.
Future Research and the Broader Implications of AI
The paper concludes by calling for further research into the long-term effects of AI on wages, housing prices, and broader societal outcomes. While the study focused on the employment-to-population ratio, AI’s influence on the economy extends beyond employment figures, potentially reshaping industries, communities, and even political landscapes. Additionally, the rapid pace of AI innovation, especially following the advent of generative AI technologies like ChatGPT, presents new uncertainties for researchers. As AI continues to evolve, understanding its broader implications will require more extensive data collection and analysis, particularly at the micro-level. Despite these challenges, Huang’s research provides valuable insights into the transformative effects of AI on local labor markets in the United States, laying the groundwork for future policy discussions aimed at managing AI’s disruptive potential.
- FIRST PUBLISHED IN:
- Devdiscourse