AI mirrors human reasoning - but with a surprising twist
Understanding how content affects reasoning in both humans and AI systems offers crucial insights into the cognitive mechanisms that underlie logical thought. It also provides a framework for evaluating whether AI can truly replicate human decision-making processes or simply reproduce patterns observed in its training data.
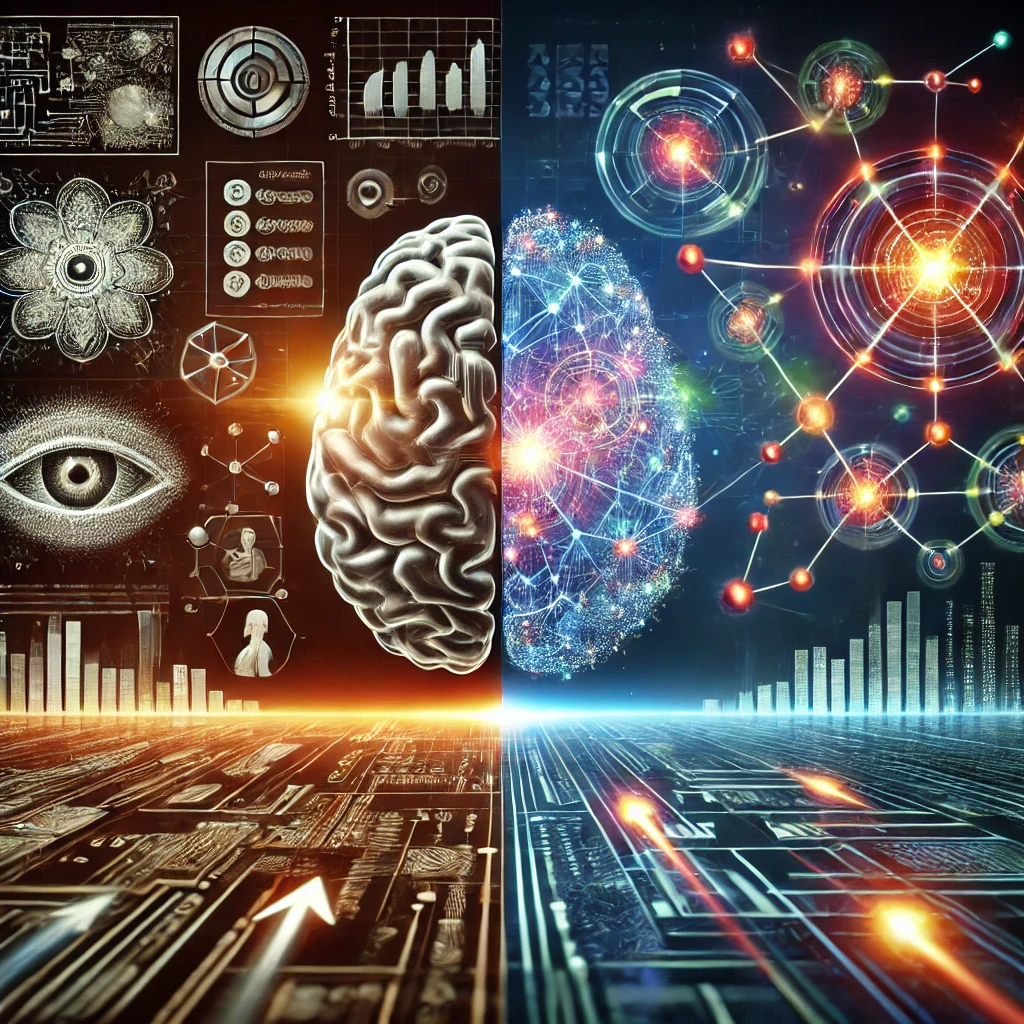
In the age of rapidly advancing artificial intelligence, the question of whether machines can think like humans has captured the imagination of researchers and the public alike. Large language models (LLMs) such as GPT-3.5 have demonstrated extraordinary abilities in understanding and generating text, raising new questions about their cognitive capacities. Do these models merely mimic human-like reasoning, or do they truly exhibit patterns of thought akin to human cognition?
A groundbreaking study titled “Language Models, Like Humans, Show Content Effects on Reasoning Tasks”, authored by Andrew K. Lampinen, Ishita Dasgupta, and colleagues, and published in PNAS Nexus, Volume 3, Issue 7, sheds light on this intriguing topic. The study examines how both humans and LLMs process logical reasoning when tasks are framed with varying semantic content, revealing surprising parallels between machine learning and human cognition.
Role of content in logical reasoning
Logical reasoning is often considered an abstract skill, ideally untainted by the specific content being reasoned about. However, in practice, humans frequently exhibit “content effects” - a tendency to perform better on reasoning tasks when they involve familiar or realistic content. For instance, people are more likely to solve logic puzzles correctly if they involve scenarios that align with everyday experiences. The study explores whether LLMs display similar content effects, suggesting that their training on human-generated data might imbue them with human-like reasoning biases.
Understanding how content affects reasoning in both humans and AI systems offers crucial insights into the cognitive mechanisms that underlie logical thought. It also provides a framework for evaluating whether AI can truly replicate human decision-making processes or simply reproduce patterns observed in its training data.
Testing reasoning across humans and LLMs
The researchers designed three reasoning tasks to assess the impact of content on logical reasoning in humans and LLMs. In the Natural Language Inference (NLI) task, participants had to evaluate the logical relationship between a premise and a hypothesis. By presenting belief-consistent, belief-violating, or nonsensical content, the researchers assessed how semantic framing influenced performance.
The syllogism task required participants to evaluate deductive arguments for validity. Here, belief alignment played a key role in determining how humans and LLMs approached logical conclusions.
Lastly, the Wason Selection Task, a well-known logic puzzle, challenged participants to falsify a conditional rule under realistic, arbitrary, or nonsensical conditions. This range of tasks provided a comprehensive view of how content affects reasoning across different contexts.
To ensure robustness, the study incorporated novel datasets specifically curated to minimize contamination from LLM training data. Human participants were recruited through online platforms and were tested under controlled conditions to evaluate their responses alongside the AI models.
Parallels and divergences between humans and LLMs
The study revealed striking similarities in how humans and LLMs approach reasoning tasks. Both groups demonstrated better performance when tasks involved realistic or belief-consistent content. For example, logical puzzles framed in familiar contexts were more likely to elicit correct responses. This parallel suggests that LLMs, like humans, are influenced by the semantic framing of reasoning tasks.
However, notable differences emerged in specific contexts. While humans struggled more with abstract or nonsensical content in complex tasks like the Wason Selection Task, LLMs often performed better, particularly in recognizing logical structures devoid of semantic cues. This divergence underscores the differences in how humans and machines process logic under challenging conditions, with LLMs leveraging their computational capacities to overcome content biases.
Implications for AI and cognitive science
The findings have significant implications for both AI development and our understanding of human cognition. The presence of content effects in LLMs highlights the extent to which these models internalize patterns from human-generated data. This raises important questions about the origins of cognitive biases and whether such biases are intrinsic to human reasoning or learned through exposure to specific content.
For AI researchers, these insights offer opportunities to refine LLMs for better reasoning performance. By addressing content sensitivity, developers can create models that excel in abstract reasoning while maintaining the contextual adaptability needed for real-world applications. For cognitive scientists, LLMs serve as valuable tools for modeling human thought processes, enabling new explorations into the mechanisms of reasoning and decision-making.
Towards improved AI reasoning
Despite their impressive capabilities, LLMs remain influenced by the content framing of tasks. This sensitivity presents both challenges and opportunities. On the one hand, content effects can limit an LLM’s ability to generalize across diverse scenarios. On the other hand, leveraging these effects can enhance performance in applications requiring context-aware decision-making. Strategies such as chain-of-thought prompting and fine-tuning on abstract reasoning datasets could help mitigate content biases and improve logical consistency.
Moreover, the study emphasizes the importance of balancing logical precision with practical utility. In many real-world applications, content sensitivity can enhance user experiences by providing contextually relevant responses. The challenge lies in optimizing AI systems to navigate both abstract reasoning and context-rich problem-solving effectively.
- FIRST PUBLISHED IN:
- Devdiscourse