Unlocking AI’s potential: Why better environments matter
Despite its potential, designing environments for AI agents comes with challenges. The vast design space and high computational cost of evaluating each intervention require efficient optimization methods.
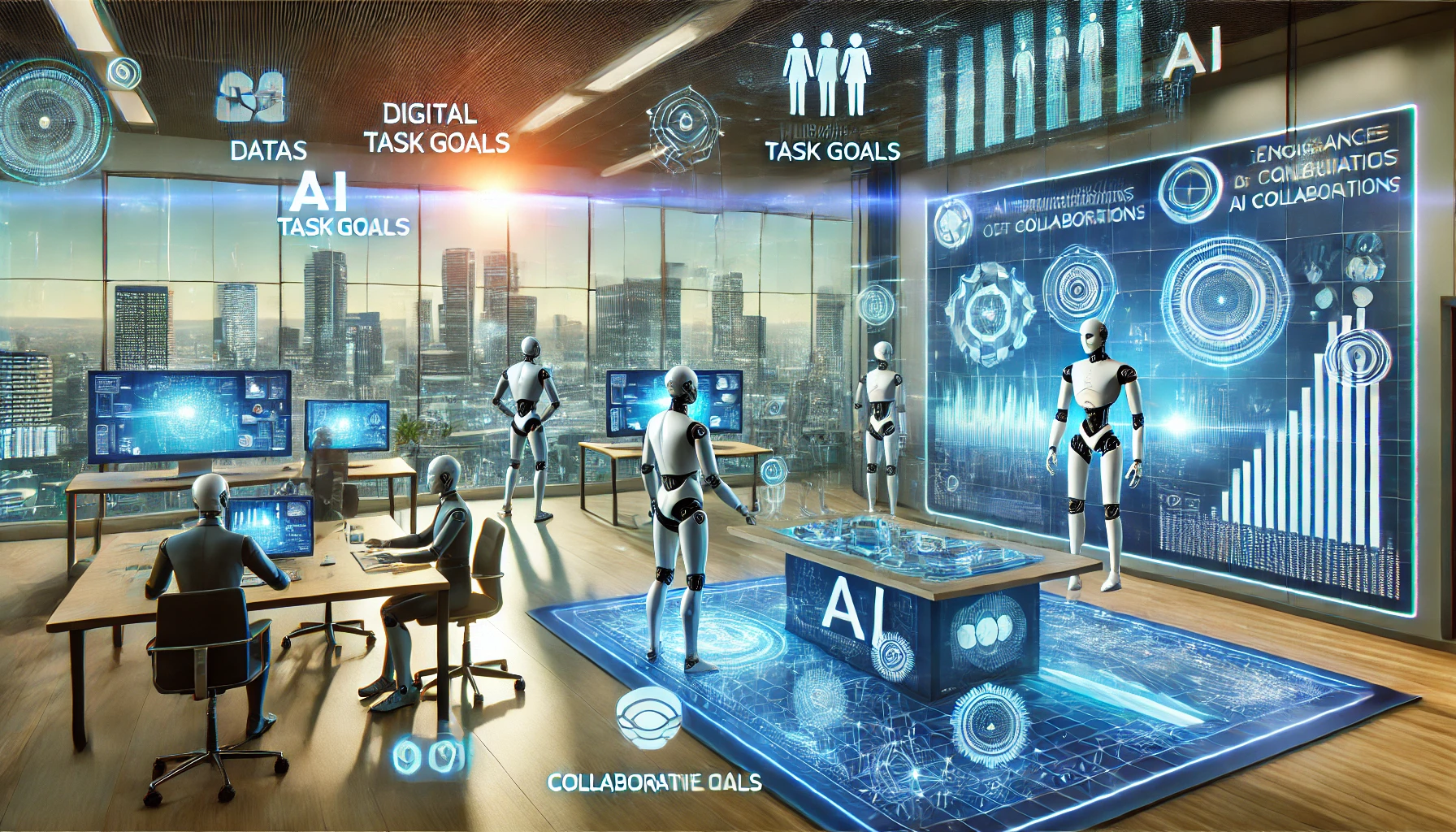
Artificial intelligence has redefined the boundaries of innovation, enabling machines to perform tasks once thought to require human intelligence. Yet, as AI systems advance, one fundamental question emerges: Are we missing an opportunity to amplify their potential by focusing solely on improving the agents themselves? In her groundbreaking study, "Better Environments for Better AI," Sarah Keren from the Technion from the Israel Institute of Technology presents a bold new perspective.
Published in the AI Magazine, Keren’s work highlights a transformative concept: intelligent systems perform better when the environments they operate in are specifically designed to enhance their capabilities. Rather than simply optimizing algorithms or hardware, Keren emphasizes designing spaces - physical, digital, or structural - that foster collaboration, communication, and efficiency among agents. This innovative approach has wide-reaching implications for fields such as robotics, smart cities, and multi-agent systems, offering a roadmap to reshape the future of AI integration.
Environment-centric AI design
Most advancements in AI have concentrated on improving the agent itself - its ability to learn, process data, and make decisions. Keren’s research challenges this conventional approach by emphasizing the critical role of the environment in determining an agent’s effectiveness. Her methods aim to identify the inherent limitations and capabilities of AI agents and use that understanding to design or modify the environments they interact with. These environments are not limited to physical spaces but include all elements agents consider while acting, such as information channels, communication protocols, and collaborative frameworks.
For instance, rather than focusing solely on the software guiding autonomous vehicles, Keren’s approach could involve strategically placing sensors on roads to minimize collisions. Similarly, in underwater exploration, designing a communication protocol for limited bandwidth between vehicles and surface vessels could dramatically enhance mission success rates. By treating the environment as a co-determinant of success, this research opens up new avenues for AI applications across domains.
Goal Recognition Design (GRD): Enhancing predictive capabilities
One of the cornerstone methodologies introduced in Keren’s research is Goal Recognition Design (GRD), which revolutionizes how agents interpret others' intentions. GRD addresses the challenge of enabling an agent to recognize another’s goals by observing behavior. Traditionally, improving goal recognition has involved enhancing agents’ processing capabilities. However, GRD takes a proactive approach by modifying the environment to make goals more distinguishable.
In an airport security context, for example, GRD could involve rearranging terminal layouts to ensure passengers’ destinations become evident earlier in their journey. If agents need to identify whether travelers are heading to domestic or international gates, placing a physical divider early in the route can significantly improve goal recognition efficiency. This reduces the time required to identify intentions while preserving operational comfort for users.
The application of GRD extends beyond security. It holds potential in areas like intrusion detection, human-robot collaboration, and even gaming, where understanding player objectives is crucial for adaptive responses. By tailoring environments, GRD not only enhances performance but also contributes to privacy preservation, as agents can achieve their goals with minimal exposure of unnecessary information.
Promoting multi-agent collaboration
The research also focuses on promoting collaboration in multi-agent AI systems, particularly in scenarios with limited data and communication constraints. Effective teamwork in these settings requires well-designed environments that optimize information flow and task distribution.
For example, in a robotics laboratory setting, agents tasked with collaborative grasping or navigation often face challenges due to limited sensing capabilities or communication bandwidth. Keren’s approach involves designing interaction protocols and modifying the physical setup to enable seamless collaboration. A strategically placed object or a carefully defined protocol can enhance performance by enabling agents to share skills or request assistance effectively.
One notable project involves multi-agent energy networks, where decentralized electricity production from renewable sources creates challenges in resource management. By modeling energy grids as multi-agent reinforcement learning systems, Keren’s team designs optimal interaction mechanisms for energy production, consumption, and storage. These solutions maximize efficiency while addressing dynamic changes in the grid.
Applications, future directions and challenges
The implications of this research extend far beyond robotics and energy networks. In healthcare, environment design can optimize the placement of medical devices for collaborative use in operating rooms. In smart cities, modifying sensor placement can improve traffic management systems, reducing congestion and enhancing safety. Even in education, tailored digital environments can enhance collaborative learning by enabling students and AI tutors to interact more effectively.
Despite its potential, designing environments for AI agents comes with challenges. The vast design space and high computational cost of evaluating each intervention require efficient optimization methods. Keren’s work addresses these issues by developing theoretical frameworks that ensure optimal solutions while minimizing unnecessary exploration. Her use of game theory, multi-agent reinforcement learning, and model-based reasoning underscores the interdisciplinary nature of this research.
Looking forward, Keren envisions a future where environment design is integral to AI development, bridging the gap between theoretical advancements and practical applications. Her work emphasizes that designing for collaboration, interpretability, and efficiency is key to realizing the full potential of AI systems.
- FIRST PUBLISHED IN:
- Devdiscourse