Can machine unlearning make AI safer for everyone?
Balancing safety and utility remains a critical goal, as unlearning must remove harmful capabilities while preserving the system's effectiveness for benign applications. Integrating human oversight into unlearning processes can further enhance reliability, particularly for complex and safety-critical systems. These steps, combined with collaborative efforts across research domains, can help establish unlearning as a valuable component of AI safety strategies.
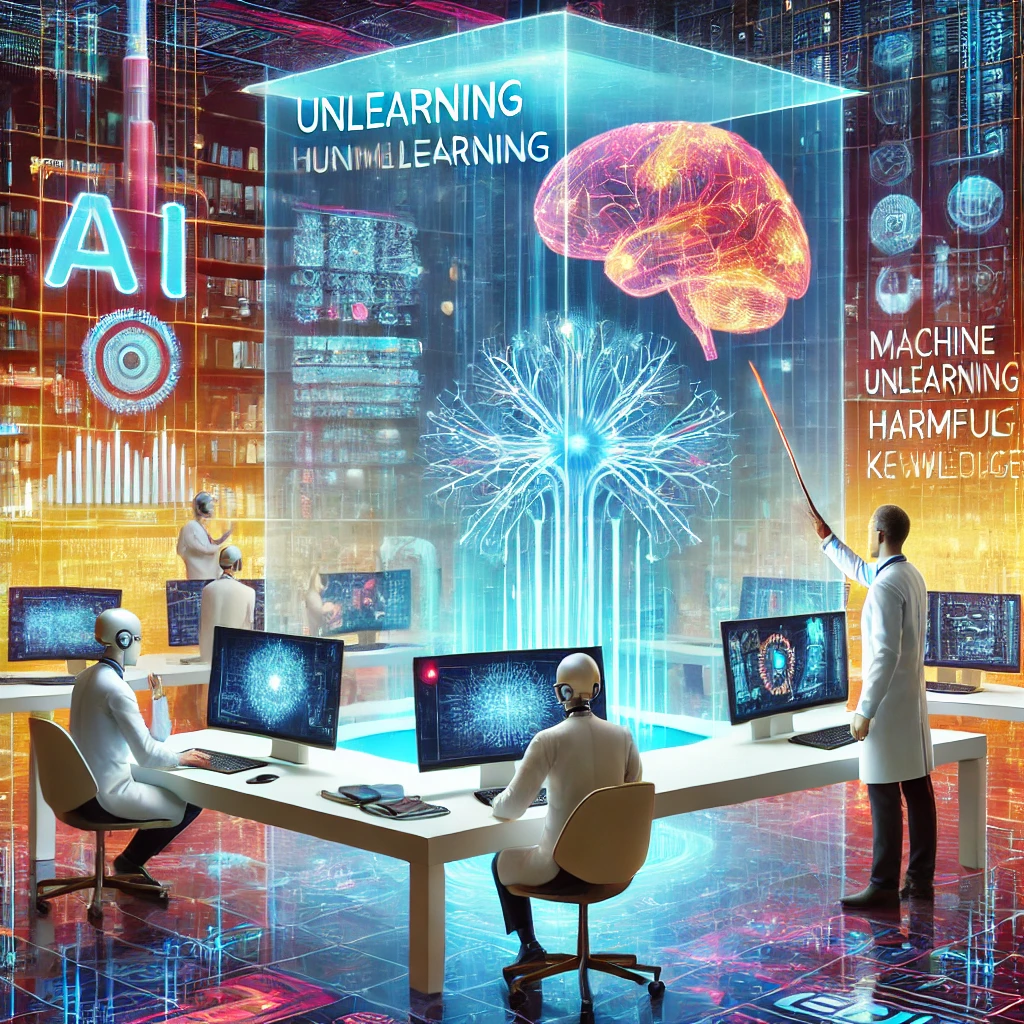
Artificial Intelligence (AI) is becoming increasingly entrenched in critical domains such as cybersecurity, healthcare, and biological research. While these systems offer significant advancements, they also pose risks, particularly through the misuse of AI capabilities for harmful purposes. The study “Open Problems in Machine Unlearning for AI Safety”, authored by Fazl Barez et al., published as a preprint on arXiv, explores the emerging field of machine unlearning. This approach, initially developed to address privacy and compliance concerns, now offers potential applications in AI safety. The research highlights unlearning as a way to remove or suppress harmful behaviors in AI models, emphasizing its role in mitigating dual-use risks, while critically analyzing its inherent challenges and limitations.
Understanding machine unlearning
Machine unlearning refers to the process by which AI systems are designed to forget specific knowledge or behaviors, effectively suppressing their ability to perform certain tasks. Originally aimed at addressing privacy issues, such as compliance with GDPR's right to be forgotten, the concept is being explored as a tool for enhancing AI safety.
By modifying the behavior of AI systems, unlearning offers potential applications in mitigating risks associated with dual-use knowledge—information or capabilities that can be exploited for both beneficial and harmful purposes. However, the paper highlights that unlearning alone is insufficient to ensure complete safety, as the interplay of retained knowledge often reconstructs harmful capabilities inadvertently.
Applications in AI safety
The research identifies key applications for unlearning in the context of AI safety, such as managing dual-use knowledge, mitigating jailbreak vulnerabilities, and correcting value misalignments. In managing dual-use knowledge, unlearning aims to remove harmful capabilities, such as synthesizing harmful chemicals, while preserving the system's utility for benign tasks.
However, this task is fraught with complexity, as seemingly innocuous knowledge interactions can inadvertently recreate suppressed capabilities. Similarly, unlearning can be applied to mitigate jailbreaks, where adversarial inputs bypass safety measures in AI systems. While this holds promise, the dynamic and evolving nature of adversarial methods presents an ongoing challenge.
Furthermore, unlearning can address value misalignments—instances where AI behaviors deviate from human intentions—though the emergent nature of alignment complicates precise modifications. Privacy-related tasks, such as removing specific data points, remain the most straightforward and successful application of unlearning, yet its broader safety applications require further exploration.
Challenges and limitations
The study underscores several challenges in implementing unlearning effectively for AI safety. A key concern is dual-use knowledge, where harmful capabilities may be reconstructed through the interaction of retained knowledge. This limits the reliability of unlearning as a means to completely suppress dangerous behaviors. Additionally, models that undergo unlearning are often vulnerable to relearning harmful knowledge through fine-tuning or adversarial inputs, raising concerns about its long-term efficacy.
Evaluating the success of unlearning is another critical issue, as existing metrics often fail to capture unintended consequences or long-term impacts on the model’s behavior. Unlearning interventions may also degrade unrelated capabilities, affecting the system's overall performance. Finally, emergent behaviors, where the interaction of safety mechanisms results in new vulnerabilities, pose additional challenges to the development and implementation of unlearning techniques.
Future directions
To address these challenges, the authors propose several avenues for future research, emphasizing the need for robust, reliable, and context-sensitive unlearning methodologies. Developing advanced unlearning techniques that prevent the reconstruction of harmful knowledge is essential, alongside creating comprehensive evaluation frameworks that assess both immediate and long-term impacts of unlearning.
Balancing safety and utility remains a critical goal, as unlearning must remove harmful capabilities while preserving the system's effectiveness for benign applications. Integrating human oversight into unlearning processes can further enhance reliability, particularly for complex and safety-critical systems. These steps, combined with collaborative efforts across research domains, can help establish unlearning as a valuable component of AI safety strategies.
In a nutshell, the study offers valuable insights into the potential and limitations of unlearning, emphasizing the need for further research and integration into broader safety frameworks. By advancing these efforts, the field can progress toward the development of safe and trustworthy AI systems.
- FIRST PUBLISHED IN:
- Devdiscourse