Unmasking hidden threats: A revolutionary approach to backdoor attacks in AI
Backdoor attacks are a subset of poisoning attacks, in which an adversary manipulates the training data to introduce vulnerabilities. By embedding a specific pattern, known as a backdoor trigger, into a small portion of the training dataset and altering their labels, attackers compromise the model’s integrity. When inputs containing the same trigger are provided during testing, the model misclassifies them as the attacker’s target label.
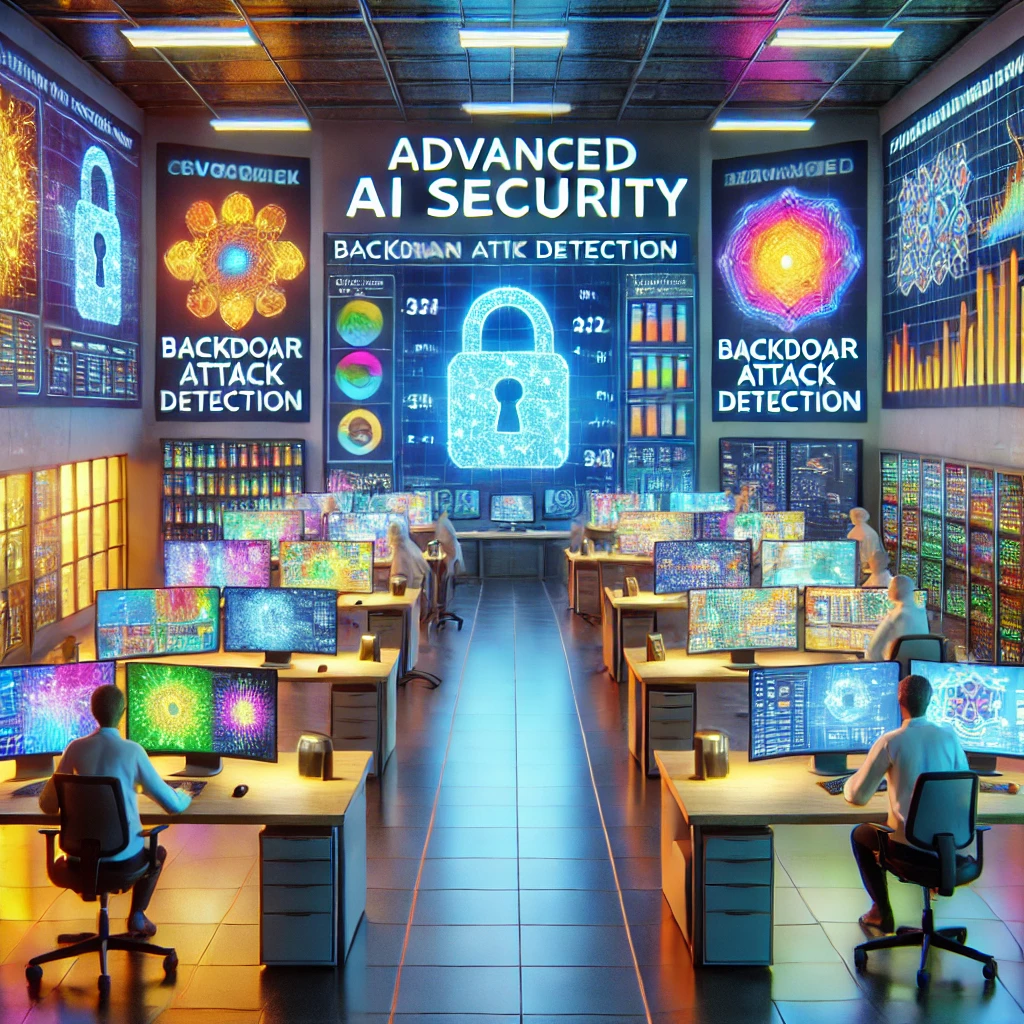
As deep learning (DL) systems become increasingly integral to fields like healthcare, autonomous vehicles, and national defense, the security of these systems has never been more critical. Backdoor attacks, a sophisticated form of adversarial attack, threaten the integrity and reliability of DL models by embedding malicious triggers during training. These triggers cause models to misclassify inputs in specific, attacker-defined ways while maintaining normal behavior for other inputs, making them difficult to detect.
In their study, "Reliable Backdoor Attack Detection for Various Sizes of Backdoor Triggers," Yeongrok Rah and Youngho Cho from the Korea National Defense University introduce a novel method to overcome the limitations of existing detection techniques. Published in the IAES International Journal of Artificial Intelligence (IJ-AI), this research offers a groundbreaking solution for safeguarding DL models against backdoor threats, irrespective of trigger size.
The growing threat of backdoor attacks
Backdoor attacks are a subset of poisoning attacks, in which an adversary manipulates the training data to introduce vulnerabilities. By embedding a specific pattern, known as a backdoor trigger, into a small portion of the training dataset and altering their labels, attackers compromise the model’s integrity. When inputs containing the same trigger are provided during testing, the model misclassifies them as the attacker’s target label.
These attacks are particularly dangerous because they can achieve a high success rate with minimal poisoning of the dataset. For instance, a trigger might be as small as a few pixels or a subtle watermark, making it undetectable during routine evaluations. Moreover, traditional defenses, like Neural Cleanse (NC), struggle to identify attacks when triggers exceed a certain size, typically 8×8 pixels, leaving a significant gap in the current security landscape.
A novel approach to detection
Recognizing the limitations of NC, Rah and Cho proposed a method that focuses on the inherent characteristics of backdoor triggers, irrespective of their size. Their approach relies on the observation that backdoor images carry features of both the ground-truth label and the attacker’s target label. This dual nature provides a unique opportunity for detection.
The method introduces perturbations to backdoor images to reclassify them into various labels, including the ground-truth label. The researchers hypothesized that the amount of perturbation required to revert a backdoor image to its ground-truth label would be abnormally small compared to the perturbation needed for other labels. This anomaly serves as the foundation for detecting backdoor triggers. Unlike NC, which measures perturbations on benign images, this method directly analyzes backdoor images, making it more robust and effective for a wide range of trigger sizes.
The researchers conducted extensive experiments using a convolutional neural network (CNN) trained on the MNIST dataset, a widely used benchmark for image classification. They tested backdoor triggers ranging in size from 1×1 to 16×16 pixels, simulating increasingly sophisticated attacks.
- Performance against large triggers: The proposed method successfully detected backdoor attacks for all trigger sizes, including large triggers (8×8 and 16×16 pixels) that NC failed to identify.
- Detection accuracy: The method achieved an average backdoor detection accuracy (BDA) of 96.3%, significantly outperforming NC, which averaged only 64.3%. For large triggers, the BDA reached 98% for 8×8 pixels and 94% for 16×16 pixels, compared to 0% for NC.
- Robustness: The method maintained consistent performance across various experimental setups, demonstrating its reliability in real-world scenarios.
These results highlight the method’s ability to address the critical limitations of NC, providing a scalable solution for detecting even the most sophisticated backdoor attacks.
Implications, challenges and future directions
The implications of this research extend far beyond its experimental success, representing a significant advancement in deep learning (DL) security by addressing a critical vulnerability in existing defenses. The proposed method fortifies DL systems against a broader spectrum of adversarial threats, ensuring the reliability and robustness of AI applications in sensitive domains such as defense, healthcare, and finance. Its scalability is another standout feature, as the method’s effectiveness across various backdoor trigger sizes makes it adaptable to diverse DL models and datasets, providing a versatile tool for AI practitioners. Furthermore, the method’s seamless integration into existing DL pipelines enhances security without disrupting established workflows, making it a practical and efficient solution for safeguarding AI systems.
While the proposed method demonstrates remarkable promise, it is not without challenges. The researchers emphasized the need for further refinement to enhance the precision, scalability, and adaptability of their approach. Future efforts could focus on extending the method to dynamic, real-time scenarios where adversarial triggers evolve over time, ensuring its effectiveness in constantly changing environments.
Additionally, developing techniques to neutralize detected backdoors without the need to retrain the entire model would significantly improve practicality. Testing the method on more complex datasets and real-world applications, such as autonomous systems and medical imaging, is also crucial to validate its generalizability across domains. Lastly, automating and streamlining the detection process to minimize computational overhead would facilitate faster deployment, making the method more suitable for time-sensitive and resource-constrained settings.
- READ MORE ON:
- backdoor attacks in AI
- AI security
- AI risks
- hidden threats in AI
- safe AI
- FIRST PUBLISHED IN:
- Devdiscourse