Safeguarding IoT networks: The role of advanced Intrusion Detection Systems
As IoT continues to expand across smart cities, healthcare, agriculture, and industrial automation, the scale and complexity of cyber threats are growing. The interconnected nature of IoT makes it vulnerable to diverse attack vectors, such as unauthorized access, data theft, distributed denial-of-service (DDoS) attacks, and malicious intrusions. Traditional security mechanisms are often insufficient for IoT environments due to the resource-constrained nature of devices and the dynamic nature of threats. This underscores the critical need for IDS that are both lightweight and adaptive.
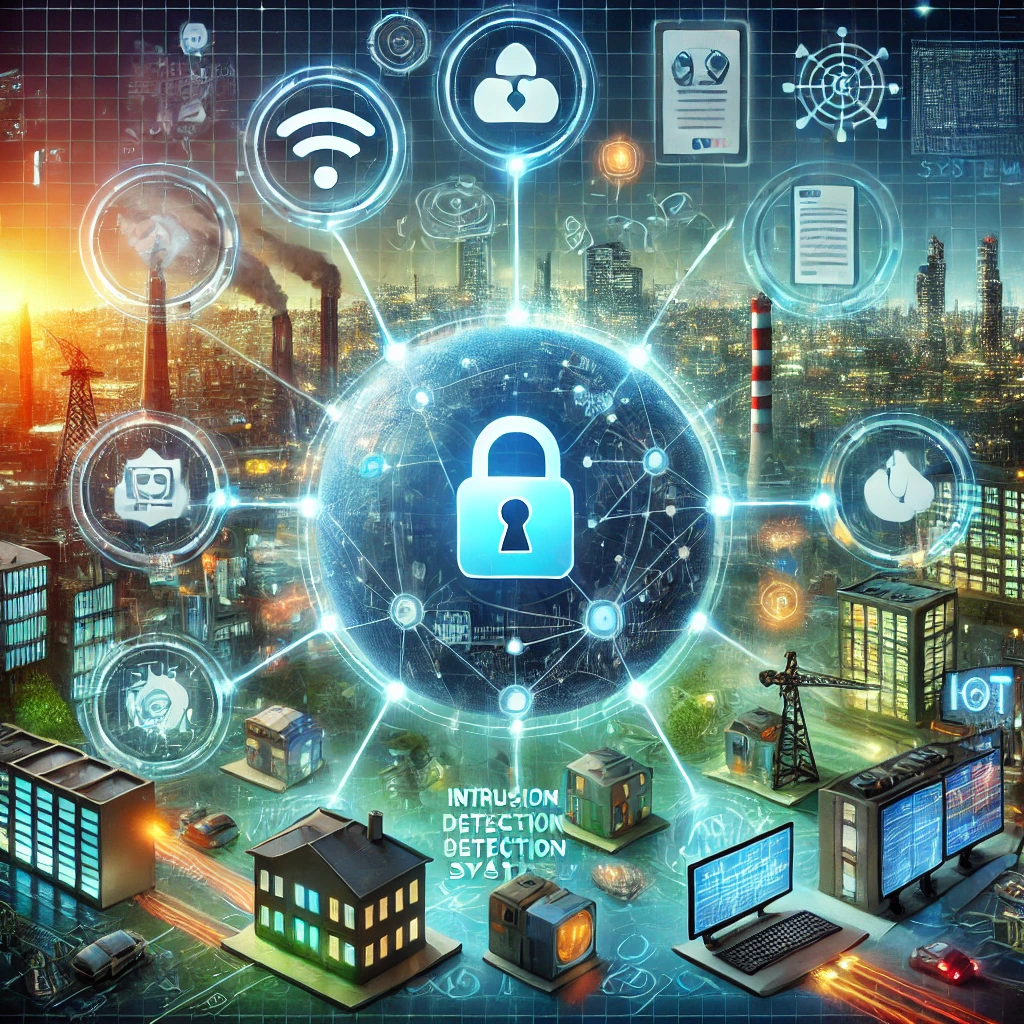
The rapid expansion of the Internet of Things (IoT) has transformed industries and daily life, enabling the seamless connection of billions of devices. However, this interconnectivity has brought significant security challenges, making IoT networks increasingly vulnerable to malicious activities. Intrusion Detection Systems (IDS) play a pivotal role in safeguarding these networks, and their evolution is critical in the face of emerging threats.
In a paper titled "A Survey on Intrusion Detection System in IoT Networks," authored by Md. Mahbubur Rahman, Shaharia Al Shakil, and Mizanur Rahman Mustakim, and published in the journal Cyber Security and Applications (2025), researchers present a detailed examination of IDS methodologies, challenges, and future directions for IoT networks.
The need for advanced intrusion detection systems in IoT
As IoT continues to expand across smart cities, healthcare, agriculture, and industrial automation, the scale and complexity of cyber threats are growing. The interconnected nature of IoT makes it vulnerable to diverse attack vectors, such as unauthorized access, data theft, distributed denial-of-service (DDoS) attacks, and malicious intrusions. Traditional security mechanisms are often insufficient for IoT environments due to the resource-constrained nature of devices and the dynamic nature of threats. This underscores the critical need for IDS that are both lightweight and adaptive.
IDS are designed to monitor network traffic, detect anomalies, and respond to malicious activities. They can be categorized into two primary approaches: signature-based methods, which rely on predefined patterns of known threats, and anomaly-based techniques, which utilize statistical or machine learning models to identify deviations from normal behavior. While signature-based systems are efficient for known threats, anomaly-based systems excel at detecting novel attacks but face challenges like high false-positive rates and computational complexity.
Key contributions
This comprehensive survey provides an in-depth analysis of state-of-the-art intrusion detection techniques, offering valuable insights into their performance, limitations, and future research directions. It evaluates various IDS models, including traditional machine learning (ML), deep learning (DL), and hybrid approaches.
Techniques such as Random Forest, Support Vector Machines, and ensemble-based models demonstrate high accuracy in detecting specific attack types, while advanced neural networks like Convolutional Neural Networks (CNNs) and Long Short-Term Memory (LSTM) networks offer sophisticated capabilities for analyzing complex datasets and temporal patterns, achieving near-perfect accuracy in several scenarios. The study also reviews widely used datasets for IoT IDS, including NSL-KDD, UNSW-NB15, and BoT-IoT, highlighting challenges such as class imbalances and outdated attack scenarios, which underscore the need for more comprehensive and representative data.
Standardized performance metrics, such as accuracy, precision, recall, and F1 scores, are emphasized as critical for evaluating IDS effectiveness, alongside computational efficiency, particularly for resource-constrained IoT devices. The authors identify emerging trends and research gaps, including high false-positive rates, computational overhead, and the need for real-world testing. They advocate for the development of lightweight models that balance performance with resource efficiency and highlight the potential of explainable AI (XAI) to build trust in IDS systems by improving transparency and interpretability.
Applications across IoT domains
The findings of this study have significant implications for securing IoT networks across various sectors. In smart cities, Intrusion Detection Systems (IDS) play a critical role in protecting essential infrastructure, such as power grids, transportation systems, and public utilities, from potential cyberattacks. In the healthcare sector, IDS are crucial for ensuring the security of IoT devices used in telemedicine, patient monitoring, and medical data exchange, safeguarding sensitive information and maintaining patient trust. Within industrial automation, IDS enhance the resilience of industrial control systems against sophisticated cyber threats, ensuring uninterrupted operations and protecting critical manufacturing and supply chain processes. These applications underscore the versatility and necessity of IDS in safeguarding IoT networks across diverse and critical domains.
Challenges and future directions
The study identifies several critical challenges in developing effective Intrusion Detection Systems (IDS) for IoT networks. A primary concern is computational complexity, as many IoT devices have limited processing power and energy resources, necessitating IDS models that are both efficient and effective.
Additionally, data imbalance in existing datasets, where certain attack types are underrepresented, can lead to biased model performance, highlighting the need for advanced data preprocessing techniques like oversampling to ensure equitable training. The challenge of adaptability is equally significant, as IDS must evolve continuously to address new and unforeseen attack patterns, making flexibility a key focus for future research.
To address these challenges, the authors propose innovative directions, including the integration of federated learning for decentralized intrusion detection, leveraging generative adversarial networks (GANs) to simulate diverse attack scenarios for robust model training, and developing compact, lightweight models tailored specifically for the resource-constrained nature of IoT devices. These approaches pave the way for more adaptive, efficient, and scalable IDS solutions in the rapidly evolving IoT landscape.
- FIRST PUBLISHED IN:
- Devdiscourse