Human-like seamless communication in robots: Conversational AI that adapt to interruptions
The ability to manage interruptions in real-time marks a significant step forward for conversational AI. This system not only enhances the fluidity of human-robot interaction but also addresses critical gaps in existing dialogue systems.
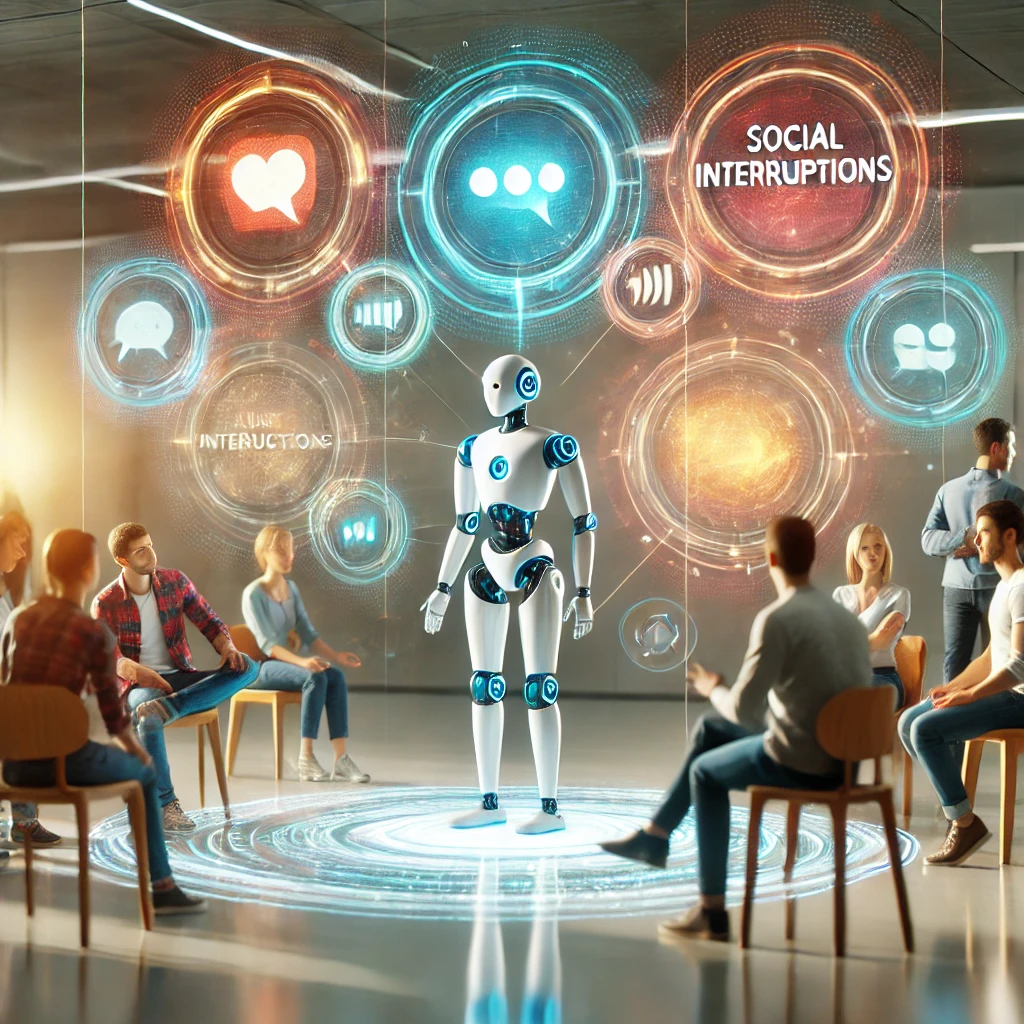
As conversational robots become an integral part of daily life, the challenge of managing interruptions effectively is increasingly critical. Interruptions, while a natural part of human communication, can disrupt conversation flow or enhance interaction dynamics depending on how they are managed. A new study titled "Interruption Handling for Conversational Robots" by Shiye Cao, Jiwon Moon, Amama Mahmood, Victor Nikhil Antony, Ziang Xiao, Anqi Liu, and Chien-Ming Huang, published by Johns Hopkins University, introduces a sophisticated system to classify and manage user-initiated interruptions in real-time. Submitted on the arXiv platform, the groundbreaking work paves the way for more intuitive and human-like interactions with robots.
Understanding interruptions in human communication
Interruptions are a common aspect of human dialogue, occurring when a listener takes the conversational floor before the speaker finishes. These interruptions serve different purposes: they can express agreement, provide assistance, seek clarification, or disrupt the speaker to introduce a new perspective. While humans are adept at navigating these situations, conversational robots have historically struggled to manage interruptions effectively. Previous systems often ignored overlapping speech or yielded the floor indiscriminately, lacking the nuance required for natural interaction.
To bridge this gap, the researchers analyzed patterns of interruption management in human conversations. They categorized interruptions into four types: cooperative agreement, cooperative assistance, cooperative clarification, and disruptive interruptions, laying the foundation for designing a robot capable of responding dynamically to varying user intentions.
A novel interruption-handling system
The system developed by the researchers leverages advanced natural language understanding to detect, classify, and manage interruptions in real-time, ensuring more seamless and intuitive interactions between humans and conversational robots. It comprises three key modules. The first is Interruption Detection, which monitors overlapping speech to identify interruptions while distinguishing them from natural conversational backchannels like "uh-huh" to prevent unnecessary disruptions.
The second module, Intent Classification, employs prompt-engineered large language models to categorize interruptions into four predefined types: cooperative agreement, cooperative assistance, cooperative clarification, and disruptive interruptions. For instance, short overlaps may indicate cooperative agreement, whereas more extensive interruptions are classified based on their context and intent.
Finally, the Interruption Handling module implements tailored strategies for responding to interruptions. Cooperative interruptions are acknowledged and addressed before the robot resumes its planned discourse, whereas disruptive interruptions may prompt the robot to either defend its conversational turn or yield the floor, depending on the specific context and interaction goals. This integrated approach allows conversational robots to mimic human-like interruption management, significantly enhancing their communicative effectiveness.
Real-world validation
To evaluate the system’s effectiveness, the researchers integrated it into a social robot and conducted user studies with 21 participants. These participants engaged in tasks specifically designed to encourage interruptions, including a timed decision-making scenario and a contentious discussion.
The results demonstrated the system’s robust performance across key metrics. The intent classification module achieved high accuracy, correctly classifying 88.78% of interruptions, showcasing its capability to distinguish between various types of interruptions. Additionally, the system successfully managed 93.69% of the 111 interruptions recorded during the study, significantly enhancing the conversational flow. This effective handling of interruptions positively impacted user experience, as participants reported feeling more included and satisfied with the discussions. These findings underscore the critical role of effective interruption management in improving user interaction and engagement with conversational robots.
Implications for conversational AI
The ability to manage interruptions in real-time marks a significant step forward for conversational AI. This system not only enhances the fluidity of human-robot interaction but also addresses critical gaps in existing dialogue systems. By tailoring responses to the intent behind interruptions, the system mimics human conversational dynamics, fostering more natural and engaging interactions. However, the study also highlights challenges, such as errors in speech recognition and the difficulty of integrating non-verbal cues like body language into the interruption-handling process. Addressing these limitations in future work could further refine the system’s performance.
Overall, this research underscores the importance of designing conversational robots that align with human expectations and social norms. By bridging the gap between human and machine communication, this innovation ensures that robots can operate as intuitive and effective partners across diverse settings, paving the way for broader acceptance and integration of conversational AI into daily life.
- FIRST PUBLISHED IN:
- Devdiscourse