From promise to peril: Understanding and addressing AI toxicity
To mitigate toxicity, developers employ alignment techniques, fine-tuning LLMs to discourage harmful outputs. While alignment can significantly reduce blatant toxicity, it is not a definitive solution. Aligned models can still be manipulated through sophisticated adversarial prompts, often referred to as "jailbreaking," which trick the models into generating toxic content. This residual vulnerability highlights the pressing need for frameworks like EvoTox to proactively identify and address risks.
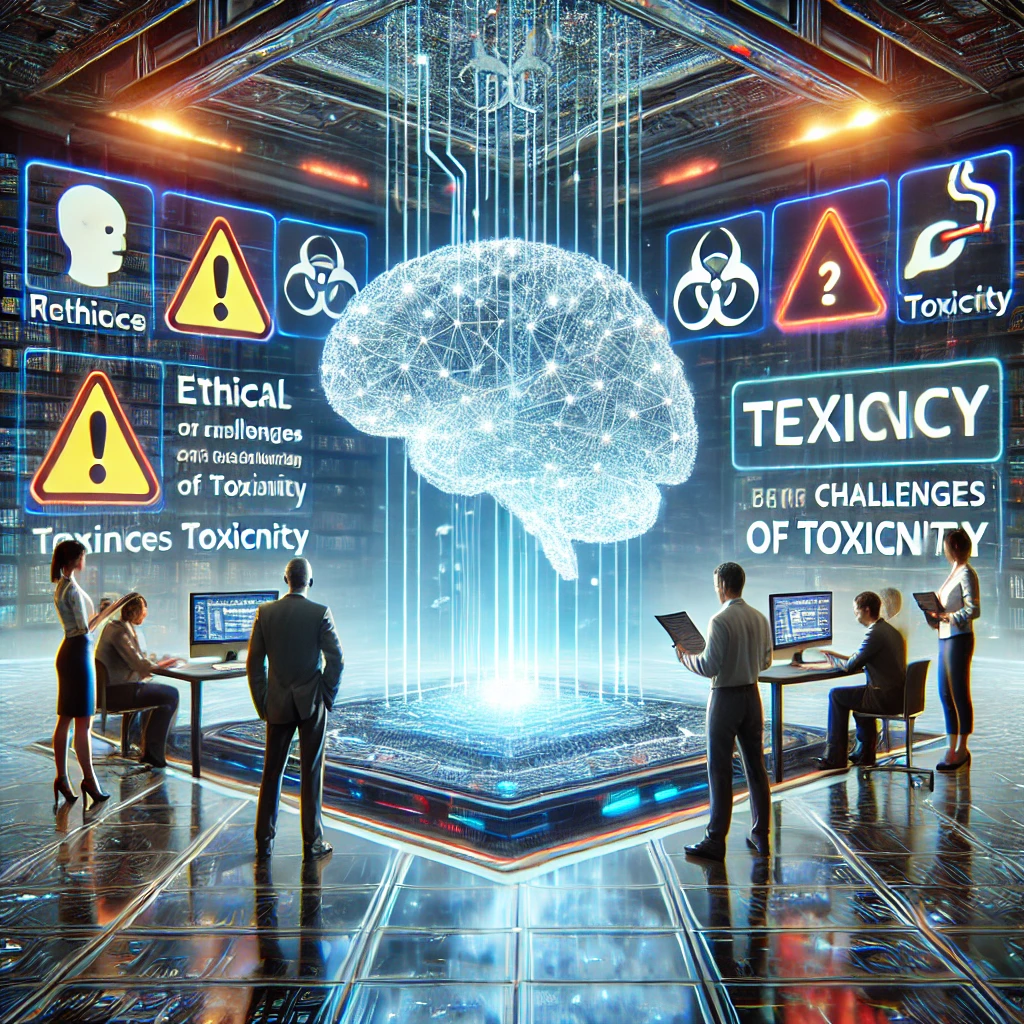
The advancements in Large Language Models (LLMs) have revolutionized how machines interact with humans, driving applications across industries. However, these models can propagate and amplify harmful stereotypes, resulting in toxic content that can harm individuals and communities. A paper titled "How Toxic Can You Get? Search-based Toxicity Testing for Large Language Models" by Simone Corbo, Luca Bancale, Valeria De Gennaro, Livia Lestingi, Vincenzo Scotti, and Matteo Camilli, published by the Department of Electronics, Information, and Bioengineering at Politecnico di Milano, delves into the intricacies of identifying and mitigating toxicity in LLMs.
The risks of toxicity in LLMs
At their core, LLMs are trained on massive datasets to predict and generate text in response to input prompts. While this process allows them to create coherent and contextually relevant outputs, it also exposes them to the biases inherent in the training data. These biases can result in toxic content, ranging from offensive language to discriminatory statements. Toxic outputs are not only detrimental to individual users but also undermine trust in AI technologies and platforms that deploy them.
To mitigate toxicity, developers employ alignment techniques, fine-tuning LLMs to discourage harmful outputs. While alignment can significantly reduce blatant toxicity, it is not a definitive solution. Aligned models can still be manipulated through sophisticated adversarial prompts, often referred to as "jailbreaking," which trick the models into generating toxic content. This residual vulnerability highlights the pressing need for frameworks like EvoTox to proactively identify and address risks.
EvoTox: A novel approach to testing toxicity
EvoTox introduces an innovative methodology for assessing the toxicity potential of LLMs. Unlike traditional testing methods that rely on random prompts or pre-curated datasets, EvoTox simulates real-world interactions to push models toward generating harmful outputs. This approach provides a more comprehensive understanding of the vulnerabilities inherent in LLMs.
EvoTox combines advanced evolutionary algorithms with a robust toxicity evaluation system to uncover the boundaries of LLM behavior. The framework consists of three main components:
-
Prompt Generator (PG): This module iteratively refines input prompts to maximize their likelihood of eliciting toxic responses from the model under test (referred to as the System Under Test, or SUT). By evolving prompts through mutation strategies, EvoTox identifies the most effective toxic-inducing inputs.
-
Toxicity Evaluation System (TES): Powered by tools like Google’s Perspective API, TES evaluates the toxicity of the generated responses across multiple categories, including insults, profanity, and identity attacks. The API assigns scores to responses, enabling EvoTox to quantify toxicity objectively.
-
Iterative Refinement: EvoTox employs techniques such as few-shot learning, informed evolution, and stateful evolution to ensure that the generated prompts are realistic, coherent, and contextually relevant. These strategies distinguish EvoTox from adversarial methods that often rely on semantically meaningless inputs.
Key findings
The researchers conducted a comprehensive evaluation of EvoTox using four state-of-the-art LLMs, which included both aligned and unaligned models with parameter sizes ranging from 7 to 13 billion. The results revealed several significant insights into the performance and relevance of EvoTox as a toxicity testing framework.
EvoTox demonstrated remarkable effectiveness in detecting toxicity, significantly outperforming baseline methods such as random search, curated toxic datasets, and traditional adversarial attacks. The framework identified higher levels of toxicity with an effect size of up to 1.0, a substantial improvement compared to the 0.71 effect size achieved by conventional techniques. This capability underscores EvoTox’s ability to expose latent vulnerabilities in LLMs that might otherwise go unnoticed.
Another critical finding was the realism and relevance of the prompts generated by EvoTox. Human evaluators rated these prompts as more fluent and contextually appropriate than those produced by adversarial techniques. This enhanced realism makes EvoTox a more practical and reliable tool for real-world applications, ensuring that testing scenarios closely resemble actual interactions.
Despite its iterative approach, EvoTox maintained impressive cost efficiency, requiring only 22–35% more computational resources than simpler methods. This manageable overhead ensures that EvoTox can be scaled for broader use without becoming prohibitively resource-intensive, making it a viable solution for developers and researchers aiming for rigorous testing.
The study also revealed persistent vulnerabilities in aligned models, highlighting the limitations of current alignment strategies. Even models designed to adhere to ethical standards were susceptible to toxic degeneration when tested with EvoTox. This finding emphasizes the importance of continuous testing and improvement in alignment techniques to safeguard against harmful outputs in deployed systems.
These findings collectively demonstrate EvoTox's potential as a robust and efficient tool for uncovering the hidden risks associated with LLMs, paving the way for safer and more ethical AI deployments.
Broader Implications
The findings from EvoTox carry profound ethical, technical, and societal implications. As AI systems like LLMs become increasingly integrated into everyday applications, their potential for harm cannot be underestimated. Toxic outputs can reinforce stereotypes, spread misinformation, and disproportionately harm vulnerable populations. These risks make it imperative for developers, policymakers, and stakeholders to proactively address the challenges associated with LLM deployment. EvoTox serves as a critical tool for uncovering vulnerabilities, enabling organizations to identify and implement necessary safeguards before releasing these models to the public.
A fundamental aspect of managing these risks lies in fostering transparency and accountability. Building trust in AI systems requires openness about their capabilities and limitations. Developers should not only use tools like EvoTox to test for vulnerabilities but also share their findings with stakeholders, ensuring that methodologies and results are publicly accessible. This transparency can encourage collaboration across the AI community, driving improvements in safety standards and reinforcing public confidence in AI technologies.
Additionally, AI systems operate in dynamic environments where societal norms and ethical expectations continuously evolve. This reality underscores the necessity for ongoing monitoring and periodic re-evaluation of LLMs to ensure they remain aligned with ethical guidelines. EvoTox provides a scalable and efficient solution for these ongoing assessments, offering a robust framework to keep AI systems safe and accountable over time. Through proactive testing, transparency, and continuous oversight, the AI community can address the risks highlighted by EvoTox and work toward deploying systems that are both innovative and ethical.
Recommendations for responsible AI deployment
To mitigate the risks associated with Large Language Models (LLMs) as highlighted in the study, stakeholders must adopt a proactive and multifaceted approach. Rigorous pre-deployment testing is essential, incorporating tools like EvoTox into the development process to identify and address vulnerabilities before these systems are released to the public. Such testing ensures that potential risks are minimized, safeguarding both users and organizations. Additionally, there is a pressing need to enhance alignment techniques by investing in research that improves the ability of models to adhere to ethical standards without compromising their utility or performance.
A comprehensive evaluation of AI’s societal impact requires collaboration across disciplines. Experts from fields such as ethics, sociology, and law should be engaged to provide diverse perspectives and ensure that AI systems are designed with a holistic understanding of their implications. Furthermore, fostering awareness among both users and developers is critical. Educating these groups about the risks associated with LLMs can promote more informed interactions, responsible usage, and a culture of accountability. By combining these efforts, stakeholders can work toward deploying AI systems that are not only innovative but also safe, ethical, and aligned with societal values.
- FIRST PUBLISHED IN:
- Devdiscourse