A new era in cloud security: Leveraging AI for real-time defense
The dynamic and interconnected nature of cloud environments makes them particularly susceptible to a wide range of cyber threats. Traditional security systems rely heavily on predefined rules and manual interventions, which often fail to address the rapidly evolving tactics of attackers. These systems struggle with the complexity of modern cloud infrastructures, which include distributed hardware, APIs, virtual machines, and dynamic networks.
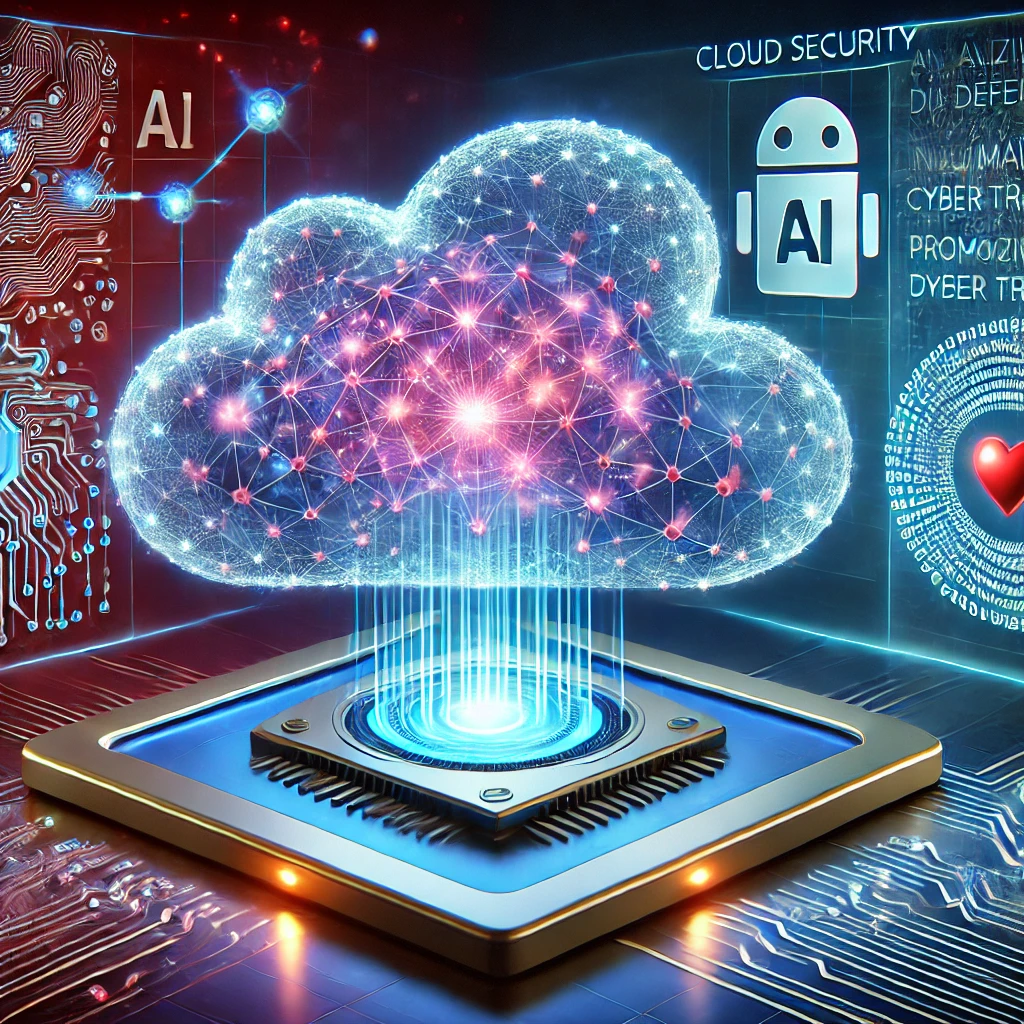
Cloud computing has become the backbone of modern digital infrastructure, powering applications across industries and enabling seamless global connectivity. However, the growing complexity of cloud environments has also made them increasingly vulnerable to sophisticated cyberattacks. Addressing these challenges requires a shift from traditional reactive defense mechanisms to proactive systems capable of anticipating and mitigating threats in real-time. A recent study, "Toward Intelligent and Secure Cloud: Large Language Model Empowered Proactive Defense," authored by Yuyang Zhou, Guang Cheng, Kang Du, and Zihan Chen and published in December 2024, introduces LLM-PD, a cutting-edge architecture leveraging Large Language Models (LLMs) to transform cloud security. This research presents a paradigm shift in the way cloud-based threats are detected, assessed, and mitigated.
Evolving threat landscape in cloud security
The dynamic and interconnected nature of cloud environments makes them particularly susceptible to a wide range of cyber threats. Traditional security systems rely heavily on predefined rules and manual interventions, which often fail to address the rapidly evolving tactics of attackers. These systems struggle with the complexity of modern cloud infrastructures, which include distributed hardware, APIs, virtual machines, and dynamic networks. Zero-day vulnerabilities, Distributed Denial of Service (DDoS) attacks, and insider threats further complicate the task of securing cloud ecosystems. The study emphasizes the limitations of reactive measures and underscores the urgent need for intelligent, adaptive, and proactive defense mechanisms to protect critical cloud assets.
LLM-PD: A proactive defense architecture
At the heart of the study is LLM-PD, an architecture designed to leverage the cognitive capabilities of LLMs to safeguard cloud environments. Unlike traditional security solutions, which respond to threats only after they have been detected, LLM-PD operates proactively by continuously monitoring, analyzing, and defending against potential vulnerabilities. The architecture consists of five key components that work in synergy:
The first stage involves collecting and standardizing data from diverse sources within the cloud ecosystem, including system logs, network traffic, and performance metrics. This data provides a comprehensive view of the cloud environment's security posture. The second stage performs risk assessment, analyzing collected data to identify vulnerabilities across hardware, software, and network layers. This is followed by task inference and decision-making, where the system uses LLMs to determine the appropriate defensive actions. Tasks are decomposed into manageable steps to ensure efficient resource allocation and precise execution. Once a threat is identified, the defense deployment stage enables the system to either invoke existing security solutions or generate custom scripts to neutralize the attack. Finally, the effectiveness analysis and feedback loop ensures that deployed measures are evaluated and refined, allowing LLM-PD to learn from each interaction and improve its defense strategies over time.
Case studies and experimental results
The study evaluates LLM-PD's capabilities through rigorous testing against advanced threats, including various forms of Denial of Service (DoS) attacks. In scenarios such as SYN flooding, SlowHTTP, and Memory DoS, LLM-PD demonstrated exceptional resilience, achieving survival rates of over 90% even under high-attack conditions. This represents a significant improvement over traditional defense mechanisms. Moreover, the architecture's adaptability was evident as it refined its strategies with each interaction, significantly reducing response times and enhancing its ability to counter complex, multi-vector attacks. Compared to existing solutions like Deep Q-Networks (DQN) and Proximal Policy Optimization (PPO), LLM-PD consistently outperformed in terms of accuracy, efficiency, and real-time decision-making.
Challenges and opportunities
While LLM-PD offers groundbreaking advancements in cloud security, the study acknowledges key challenges that must be addressed for widespread adoption. A significant hurdle is the explainability of LLMs. As these models increasingly take on critical security roles, stakeholders must understand how decisions are made to ensure trust, transparency, and accountability. The dynamic nature of cloud environments also presents a challenge, requiring continuous updates to the architecture to keep pace with emerging threats without overburdening computational resources. Additionally, integrating LLM capabilities directly into cloud infrastructure, rather than as an auxiliary layer, could further enhance responsiveness and resilience against sophisticated attacks.
The study also highlights opportunities for advancing the field of cybersecurity. By investing in privacy-preserving AI technologies, such as federated learning and homomorphic encryption, developers can ensure that LLMs process data securely without compromising user privacy. Furthermore, fostering collaboration between cloud service providers, researchers, and policymakers can lead to standardized practices and regulations, ensuring that proactive defense systems align with global security standards.
As cloud computing continues to expand, proactive systems like LLM-PD will be crucial in ensuring resilience and trust in an increasingly interconnected world.
- FIRST PUBLISHED IN:
- Devdiscourse