From bench to code: How AI is reshaping legal practice
AI has created unparalleled opportunities for the legal sector. Traditionally time-consuming tasks like contract drafting, legal research, and document review can now be automated, saving resources and improving efficiency. However, this potential is tempered by significant challenges, particularly the phenomenon of "hallucinations." This occurs when large language models (LLMs) generate text that is coherent but factually inaccurate or misleading. Such errors, if unchecked, can lead to dire legal consequences, undermining trust in AI-driven systems.
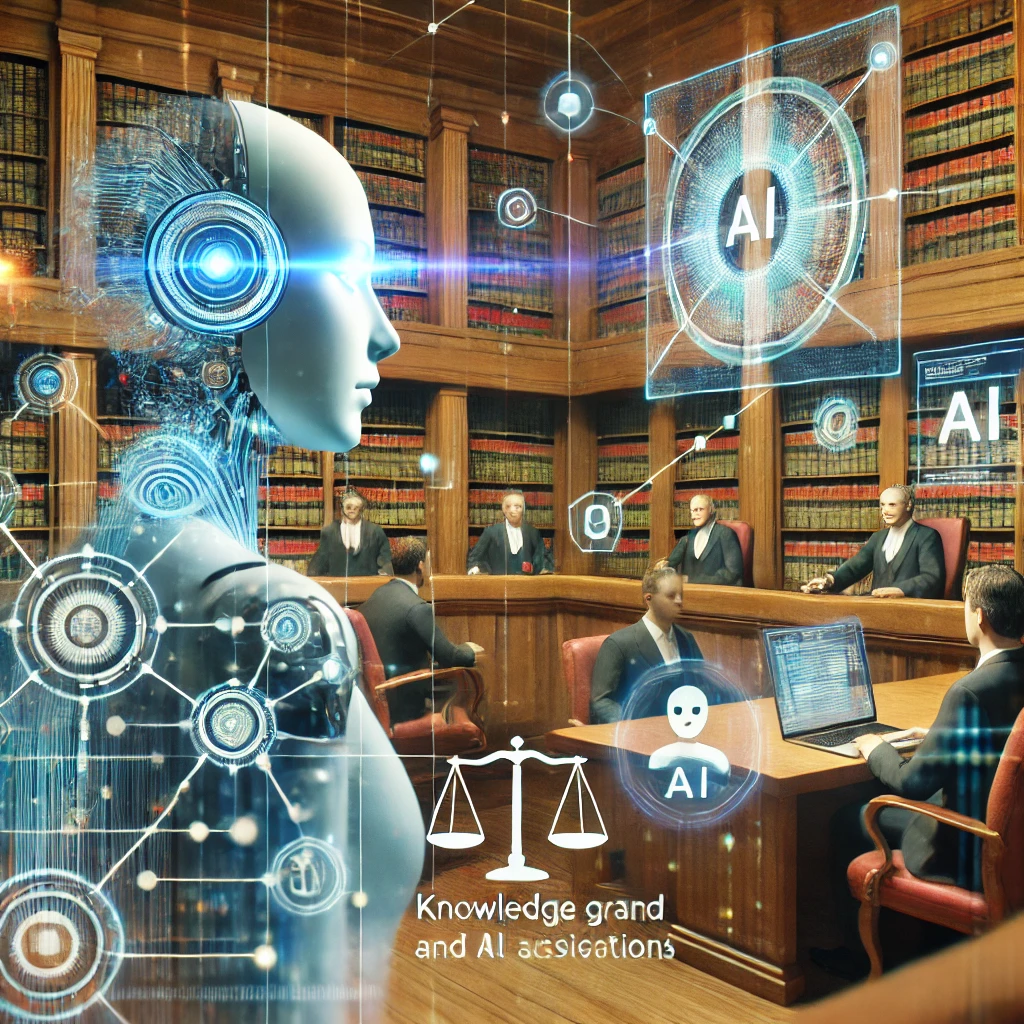
Artificial Intelligence (AI) is transforming industries, and the legal profession is no exception. Known for its heavy reliance on accuracy, detailed documentation, and nuanced logic, the legal domain is witnessing a technological revolution driven by advancements in AI. The study "A Comprehensive Framework for Reliable Legal AI: Combining Specialized Expert Systems and Adaptive Refinement" by Sidra Nasir, Qamar Abbas, Samita Bai, and Rizwan Ahmed Khan, available on arXiv, provides a groundbreaking framework that addresses the limitations of current legal AI systems. By integrating specialized expert systems with advanced AI techniques, the research introduces a scalable, accurate, and contextually relevant approach to enhancing AI's role in the legal domain.
The intersection of AI and Law: Opportunities and challenges
AI has created unparalleled opportunities for the legal sector. Traditionally time-consuming tasks like contract drafting, legal research, and document review can now be automated, saving resources and improving efficiency. However, this potential is tempered by significant challenges, particularly the phenomenon of "hallucinations." This occurs when large language models (LLMs) generate text that is coherent but factually inaccurate or misleading. Such errors, if unchecked, can lead to dire legal consequences, undermining trust in AI-driven systems.
Moreover, while traditional legal services are often prohibitively expensive, leaving many without adequate representation, AI holds the promise of democratizing access to justice by providing affordable solutions for routine legal tasks. The challenge, however, lies in ensuring that these AI systems are reliable, ethical, and capable of handling the complexities of legal language, logic, and domain-specific requirements.
A new framework: Bridging accuracy and efficiency
The study proposes a novel AI framework that combines advanced methodologies such as Retrieval-Augmented Generation (RAG), Knowledge Graphs (KG), and Reinforcement Learning from Human Feedback (RLHF) to address these challenges. At its core, the framework employs a Mixture of Experts (MoE) model, wherein specialized modules are fine-tuned for distinct legal tasks like case analysis, contract classification, and statutory interpretation. This modular approach ensures that complex queries are routed to the most relevant domain expert, significantly improving accuracy and contextual relevance.
Key Components of the Framework:
-
Retrieval-Augmented Generation (RAG): The RAG model retrieves relevant legal documents from extensive repositories, grounding AI responses in verified information. By leveraging a dense vector-based retrieval system, RAG ensures that only the most contextually pertinent documents contribute to the generated outputs.
-
Knowledge Graphs (KG): These graphs enrich the retrieval and generation process by incorporating relational context, such as how statutes, cases, and principles interconnect. For example, a query about "precedents supporting Statute X in contract disputes" would be enhanced with relational data from KG, yielding not only relevant cases but also their significance and applicability.
-
Reinforcement Learning from Human Feedback (RLHF): By integrating direct feedback from legal experts, the framework continually refines its performance, aligning outputs with human expectations and established legal standards. This feedback loop addresses limitations like hallucinations and improves the system's ability to handle nuanced legal queries.
-
Structured Operational Guidelines: Modeled on real-world legal practices, these guidelines ensure the system adheres to systematic workflows, emulating the methodologies used by law firms in case analysis, precedent research, and drafting.
Real-world implications
The proposed system represents a significant leap forward in legal AI capabilities. In benchmarking tests across nine legal tasks—including document summarization, case classification, judgment prediction, and legal element extraction - the framework consistently outperformed existing models. Fine-tuned models utilizing RAG and KG achieved higher accuracy rates and lower abstention rates compared to baseline models. RLHF-enhanced systems demonstrated improved alignment with user expectations, reducing errors in open-ended and complex tasks. Modular specialization within the MoE framework ensured task-specific precision, enabling the system to handle both structured tasks such as text classification and unstructured tasks like judgment prediction with remarkable efficacy.
These advancements translate into practical benefits for the legal profession. By automating routine tasks, the framework reduces reliance on expensive human labor, making legal services more accessible. It can handle large volumes of legal queries, from document reviews to advisory consultations, with consistent quality. Grounded in verified legal data and refined through expert feedback, the framework mitigates risks associated with inaccurate or biased outputs.
Ethical and practical considerations
While the framework addresses many of the technical challenges of legal AI, the study emphasizes the importance of ethical considerations. The integration of AI into legal decision-making must prioritize transparency, fairness, and accountability. The framework's use of structured guidelines and RLHF ensures that outputs are not only accurate but also ethically aligned with human-centric values.
Furthermore, the authors advocate for ongoing collaboration between AI developers and legal professionals to refine and expand the system's capabilities. Future directions include incorporating real-time legal updates to ensure responses remain current, expanding expert modules to cover additional legal domains, such as intellectual property or international law, and enhancing explainability features to make AI-generated outputs more comprehensible to non-experts.
Overall, the study offers a visionary roadmap for integrating AI into the legal sector. By combining specialized expert systems with cutting-edge AI methodologies, the proposed framework delivers a scalable, accurate, and ethical solution to the challenges of modern legal practice. It not only addresses current limitations but also sets the stage for a future where AI acts as a trusted partner in delivering justice, safeguarding the principles of accuracy, fairness, and accountability that underpin the rule of law.
- READ MORE ON:
- AI in legal practice
- legaltech
- AI and Law
- legal AI system
- AI
- FIRST PUBLISHED IN:
- Devdiscourse