Understanding human movement: AI’s revolutionary approach to health and safety
By bridging the gap between biomechanics and AI, this study offers profound insights into how walking patterns can reveal our individuality and inform applications in healthcare and security. It highlights the transformative potential of AI in decoding human movement and underscores the importance of leveraging diverse datasets to build reliable, real-world-ready systems.
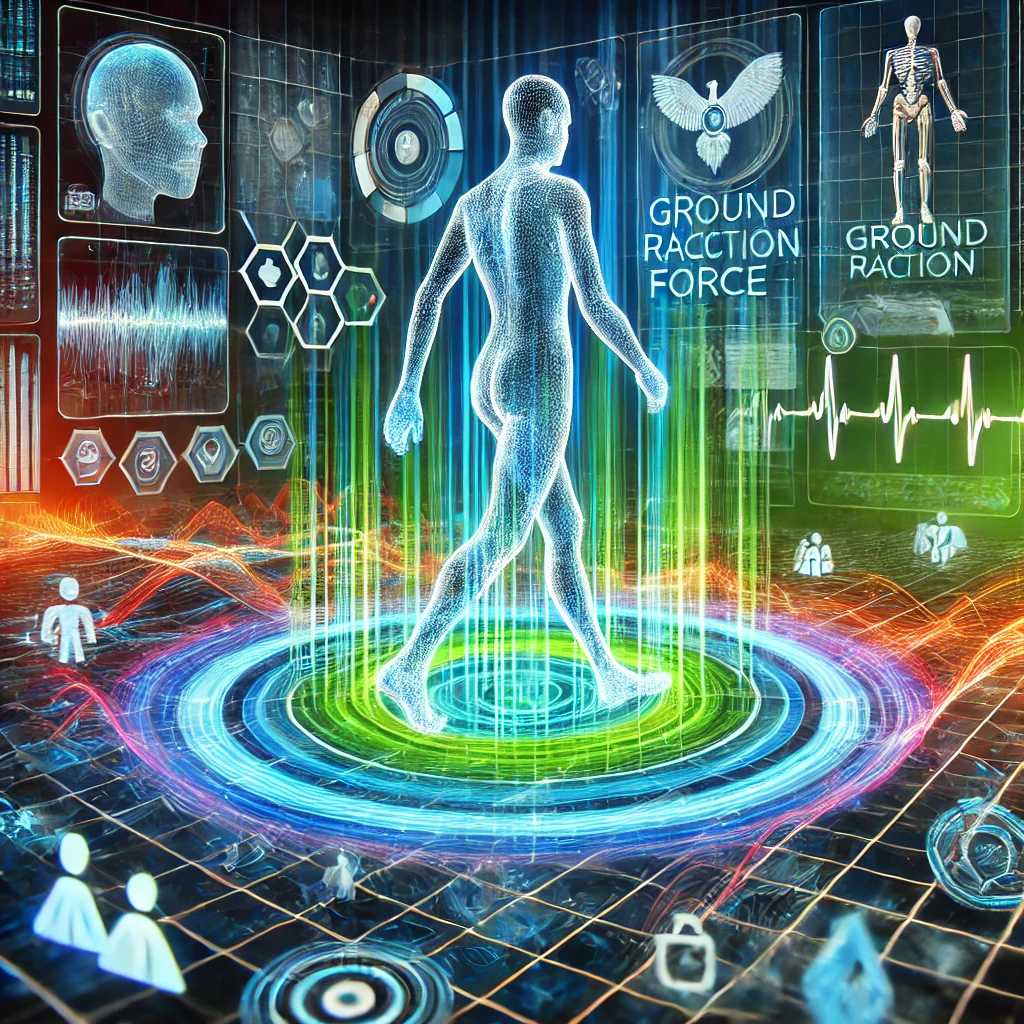
The way humans walk - our gait - is a uniquely personal trait, shaped by an intricate combination of biomechanics, environmental influences, and individual characteristics. Beyond its functional purpose, gait carries valuable information about our identity, health, and mobility. Recent advancements in artificial intelligence (AI) and machine learning (ML) have revolutionized our ability to analyze these walking patterns, unlocking transformative applications in healthcare and security.
A landmark study titled "Modelling Individual Variation in Human Walking Gait Across Populations and Walking Conditions via Gait Recognition," authored by Kayne A. Duncanson, Fabian Horst, Ehsan Abbasnejad, Gary Hanly, William S. P. Robertson, and Dominic Thewlis, published in the Journal of the Royal Society Interface, delves into how AI can model and understand human gait with unparalleled precision.
Leveraging AI to decode walking patterns
The study analyzed ground reaction force (GRF) data collected from over 740 individuals across diverse populations and walking conditions. GRF data, which measures the forces exerted on the ground during walking, provides a comprehensive view of gait mechanics. By leveraging neural networks and advanced ML techniques, the researchers aimed to decode the variability in gait patterns influenced by factors such as walking speed, footwear, body mass, and demographic attributes like age, sex, and height. These AI models achieved recognition accuracies between 52% and 100%, depending on the diversity of the dataset and walking conditions. Notably, individuals walking in personal footwear at varying speeds were identified with an accuracy ranging from 89% to 99%, demonstrating the robustness of ML in capturing personalized gait traits.
One of the study's most significant contributions was its emphasis on dataset diversity. Models trained on datasets encompassing a wide range of walking conditions - such as variations in footwear, speed, and surface - were significantly better at generalizing to real-world scenarios. This finding underscores the importance of exposing AI systems to realistic variability, ensuring their robustness and reliability outside controlled laboratory environments. The use of Explainable AI (XAI) methods further enhanced the study’s insights by revealing the variables most critical to gait recognition. Among these, walking speed emerged as the most influential factor, followed by footwear type and body mass. These findings provide actionable knowledge for designing more effective AI models that are sensitive to both individual and environmental influences.
Applications, challenges, and the road ahead
The potential applications of this research span multiple domains. In healthcare, gait analysis has long been a valuable tool for diagnosing and managing conditions such as Parkinson’s disease, arthritis, and musculoskeletal disorders. This study’s findings pave the way for AI-powered systems capable of providing personalized assessments and continuous monitoring, potentially revolutionizing patient care. For instance, real-time gait analysis could help clinicians track recovery progress in post-surgical patients or detect early signs of neurodegenerative diseases. Such systems, driven by AI and GRF data, have the potential to transition from controlled lab settings to wearable devices and IoT platforms, enabling real-world, continuous monitoring.
In the realm of security, gait recognition emerges as a promising biometric tool for identity verification and surveillance. Unlike facial recognition or fingerprints, gait is non-intrusive and difficult to disguise, offering an additional layer of security. The ability to recognize individuals based on their unique walking patterns could enhance systems for access control, border security, and crime prevention. However, as the study acknowledges, implementing such systems at scale presents challenges. These include ensuring the scalability of AI models to handle larger datasets, managing distribution shifts between training data and real-world conditions, and safeguarding sensitive biometric data through robust encryption and data security measures.
The study also identifies future directions to advance the field. Integrating AI-powered gait analysis systems with wearable sensors could improve data collection, while advancements in cryptographic techniques could secure sensitive gait data from unauthorized access. The researchers advocate for interdisciplinary collaboration among computer scientists, biomechanists, and healthcare professionals to overcome existing challenges. Standardizing data collection protocols and incorporating ethical considerations into system design will be critical to ensuring that these technologies are both effective and socially responsible.
By bridging the gap between biomechanics and AI, this study offers profound insights into how walking patterns can reveal our individuality and inform applications in healthcare and security. It highlights the transformative potential of AI in decoding human movement and underscores the importance of leveraging diverse datasets to build reliable, real-world-ready systems. As AI continues to evolve, its ability to analyze gait will unlock deeper understandings of human mobility, identity, and health, paving the way for a future where every step is a key to personalization and well-being.
- FIRST PUBLISHED IN:
- Devdiscourse