A new lens on AI: Building systems that think, learn, and act like humans
As this research continues to evolve, it has the potential to redefine AI’s role in industries ranging from robotics to smart systems. The Thousand Brains Project offers a vision of AI that is not just intelligent but truly adaptable, opening the door to a new era of machine learning and sensorimotor intelligence.
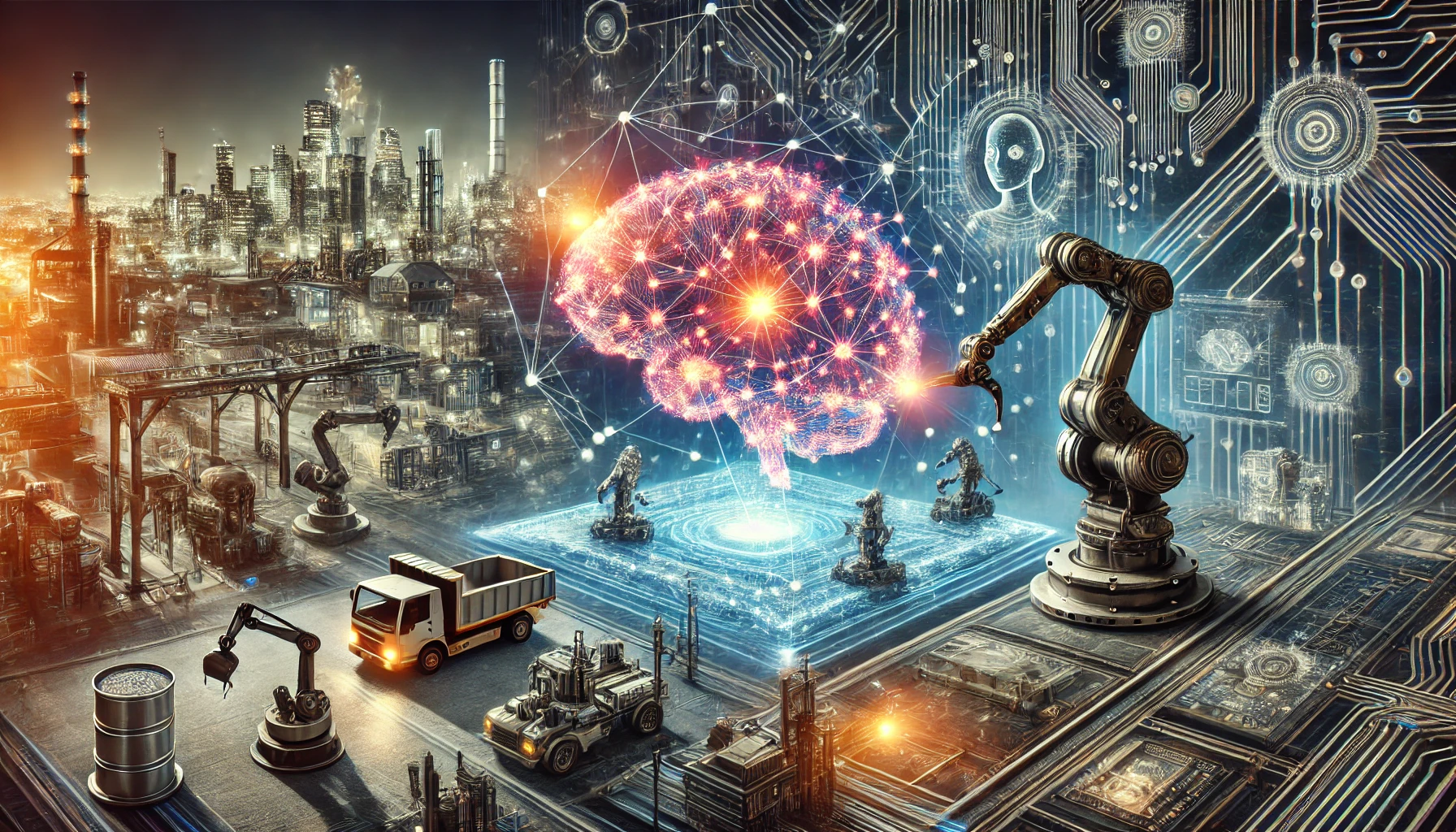
The field of Artificial Intelligence (AI) has long sought to replicate the adaptive, flexible intelligence demonstrated by humans. However, despite notable advancements, traditional AI models often struggle with dynamic environments and real-time decision-making. A groundbreaking study titled "Thousand Brains: Sensorimotor Foundations of Intelligence and Cortical Communication Protocol" by Viviane Clay, Niels Leadholm, and Jeff Hawkins, available on arXiv in December 2024, introduces a revolutionary framework inspired by the human neocortex. This research offers a new lens to develop AI systems capable of generalizing and learning through real-world interactions, promising to transform how machines perceive and act in their environments.
The Core Idea: Thousand Brains Theory of Intelligence
At the heart of this study lies the Thousand Brains Theory of Intelligence. This theory posits that the human neocortex comprises thousands of independent yet cooperative processing units called cortical columns. Each column independently builds spatial models of objects and environments, which collectively generate robust and adaptive intelligence.
Building on this biological inspiration, the researchers developed Monty, an AI architecture that mirrors the modular and adaptive nature of cortical columns. Unlike conventional AI models that require extensive labeled datasets, Monty learns through interaction, continuously updating its understanding via sensorimotor feedback loops. This dynamic learning process allows Monty to interpret, adapt, and respond to complex environments - a significant leap from static learning systems.
The study emphasizes two pivotal concepts: sensorimotor learning and reference frames. These ideas redefine how AI systems approach problem-solving and interaction with the physical world.
-
Sensorimotor Learning: Monty integrates sensory inputs (e.g., vision, touch) with motor outputs, enabling real-time interaction and adaptation. This mechanism mimics how humans perceive the world, interact with objects, and refine their understanding through movement and observation.
-
Reference Frames: These are spatial representations that allow Monty to map sensory data into structured models. Just as humans conceptualize objects in three-dimensional space, Monty uses reference frames to understand the properties, positions, and relationships of objects, enabling it to generalize knowledge across contexts.
For example, if Monty learns how to manipulate a specific tool in one environment, it can apply that knowledge to similar tools in other scenarios. This adaptability is a significant advancement over task-specific AI models.
Innovations in AI Design: The Cortical Messaging Protocol
A critical breakthrough in this study is the development of the Cortical Messaging Protocol (CMP).This protocol acts as a universal communication framework for Monty’s modular components, including its learning modules, sensor systems, and motor controls.
-
Modular Scalability: CMP ensures seamless integration of new modules, enabling Monty to scale its capabilities without redesigning its architecture. For instance, new sensor types or motor functions can be added without disrupting the system's core functionality.
-
Cross-Domain Adaptability: The modular design allows Monty to operate across diverse domains. In a smart city scenario, for example, modules for traffic control could be integrated with others designed for building automation, all coordinated through CMP.
The CMP framework embodies the flexibility and interoperability that are essential for deploying AI systems in real-world applications.
Real-world applications
The study highlights Monty’s practical capabilities through real-world applications, particularly in object recognition, robotic manipulation, and navigation. Utilizing its sensorimotor learning and reference frames, Monty is able to identify objects, estimate their positions, and execute tasks such as picking and placing items in unstructured environments. These abilities make it highly versatile for various industries.
In autonomous vehicles, Monty’s adaptive learning could significantly improve navigation in dynamic settings, minimizing dependence on pre-programmed routes. In healthcare, its precise object manipulation offers the potential to revolutionize surgical robotics and enhance patient assistance systems, introducing new levels of accuracy and efficiency. Additionally, Monty’s scalable architecture is well-suited for industrial automation, supporting a wide range of manufacturing processes, including quality control and assembly line optimization. These applications underline Monty’s adaptability and its potential to transform diverse domains with its innovative approach to AI.
Challenges and future directions
The study acknowledges challenges such as computational demands and ensuring real-time efficiency in complex environments. Future research will focus on optimizing Monty’s architecture for multi-agent collaboration and exploring its potential for abstract reasoning tasks.
The team also envisions integrating Monty into cloud-based ecosystems, enabling distributed learning and resource sharing across interconnected devices.
As this research continues to evolve, it has the potential to redefine AI’s role in industries ranging from robotics to smart systems. The Thousand Brains Project offers a vision of AI that is not just intelligent but truly adaptable, opening the door to a new era of machine learning and sensorimotor intelligence.
- FIRST PUBLISHED IN:
- Devdiscourse