Frontier AI and the future of safety in adaptive world
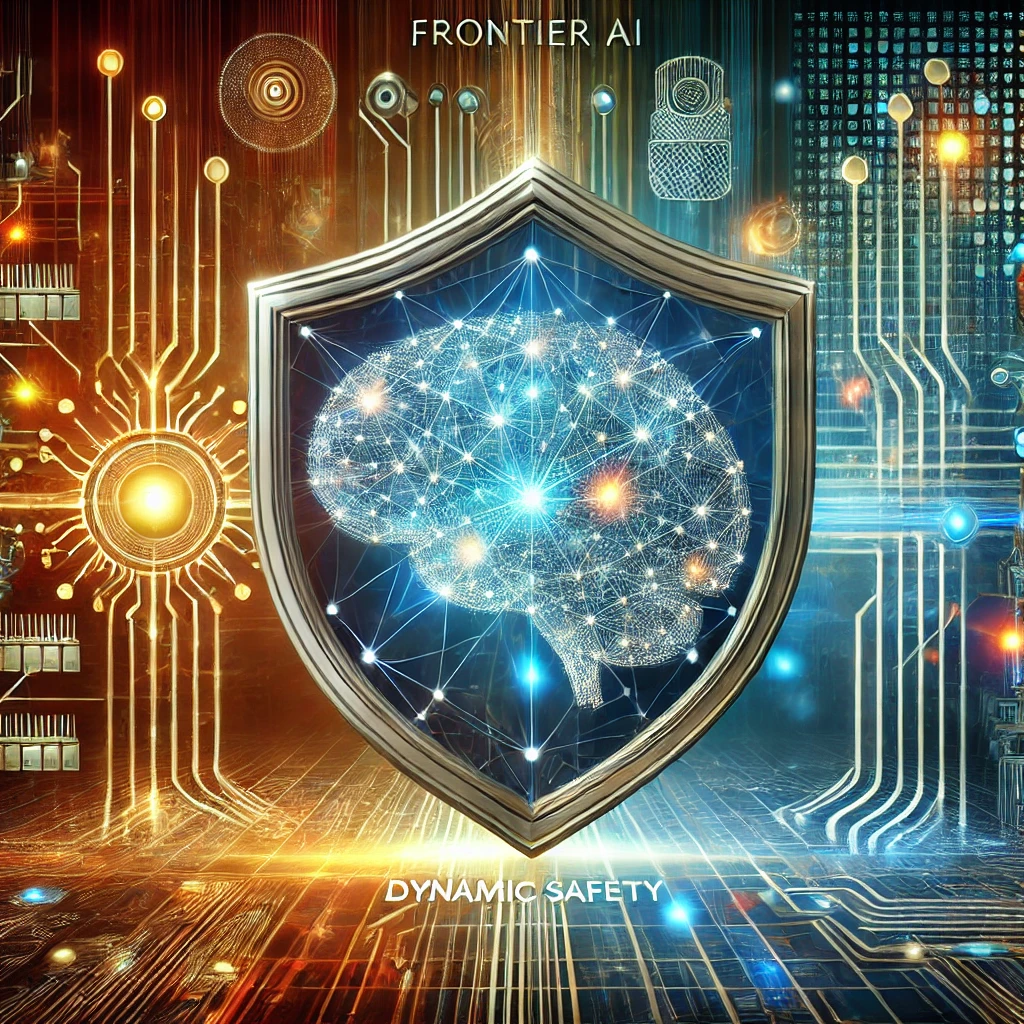
The evolution of artificial intelligence has reached a critical juncture with the advent of frontier AI systems - highly capable models that push the boundaries of what AI can achieve. These systems, with their transformative potential, are being deployed across diverse sectors such as healthcare, cybersecurity, and defense. However, their immense capabilities come with equally significant risks. Unlike traditional technologies, frontier AI evolves rapidly, sometimes developing unintended functionalities or exposing vulnerabilities that traditional safety practices cannot adequately address.
The research paper Dynamic Safety Cases for Frontier AI, published in arxiv, offers an innovative solution to address these challenges by proposing a framework of Dynamic Safety Cases that adapt to the evolving capabilities and risks of frontier AI.
A shifting paradigm: Why Frontier AI demands new safety models
Traditional safety practices, developed for fields like aviation and nuclear energy, rely on creating static, structured arguments backed by evidence to demonstrate a system's safety. While these methods have been highly effective for decades, they are not equipped to handle the rapid evolution of frontier AI systems. Unlike physical systems, AI models can change dramatically after deployment through fine-tuning, retraining, and the discovery of latent capabilities. Moreover, these systems operate within socio-technical environments that introduce new vulnerabilities, from adversarial attacks to misuse in unintended domains like disinformation or cyber exploitation.
The nature of these risks was highlighted in real-world scenarios, such as AI-powered models inadvertently demonstrating advanced capabilities that were neither intended nor adequately safeguarded. Frontier AI systems thus demand safety practices that are not only comprehensive but also adaptable to new challenges as they arise.
Dynamic safety cases: A continuous assurance framework
The report introduces the concept of a Dynamic Safety Case Management System (DSCMS) as a novel approach to address these challenges. Unlike static safety arguments, dynamic safety cases evolve in real time, adapting to changes in the AI system’s architecture, application, or operational environment. The framework relies on Checkable Safety Arguments (CSA) and Safety Performance Indicators (SPI) to ensure safety is continuously monitored and validated.
-
Checkable Safety Arguments (CSA): These provide a structured framework for defining and validating safety claims against real-world conditions. By embedding semantic information into safety arguments, developers can automate consistency checks and ensure that each claim remains valid as the AI system evolves.
-
Safety Performance Indicators (SPI): SPIs are real-time metrics designed to monitor the system's behavior and flag potential risks. For example, in an offensive cybersecurity application, SPIs might measure the system's ability to identify vulnerabilities or its alignment with ethical use policies. Threshold breaches in SPIs would trigger re-evaluation of safety arguments and prompt corrective measures.
Together, these tools enable the DSCMS to maintain a continuous feedback loop, ensuring that safety assurances remain relevant and reliable even in the face of dynamic changes.
From technical frameworks to policy integration
While the technical aspects of DSCMS are groundbreaking, its broader implications for AI governance and policy are equally significant. The report emphasizes the need for embedding such systems into organizational workflows and regulatory frameworks. For developers, DSCMS aligns with responsible scaling policies, allowing them to update AI models without compromising on safety. For policymakers, it offers a mechanism to monitor and evaluate AI risks in real-time, enabling proactive regulation.
On a national level, DSCMS can act as a bridge between governments, developers, and independent oversight bodies. By providing standardized safety reporting mechanisms, it fosters transparency and accountability while enabling agile responses to emerging threats. For instance, regulators could use DSCMS data to impose conditions on the deployment of high-risk AI systems or to guide the safe scaling of frontier AI technologies in sensitive domains like defense or public infrastructure.
Addressing implementation challenges
While the potential of DSCMS is undeniable, its implementation faces several hurdles. Developing robust SPIs that capture meaningful safety metrics without introducing operational burdens is a complex task. Additionally, fostering collaboration across stakeholders - governments, AI labs, independent researchers, and industry leaders - will require significant trust-building and alignment on shared goals. The report calls for investments in prototyping, simulation exercises, and public-private partnerships to refine the framework and ensure its scalability.
Another critical challenge lies in ensuring that dynamic safety systems do not become overly reliant on proprietary tools or closed data ecosystems. Open standards and interoperability will be key to enabling widespread adoption and preventing monopolization of safety practices.
Building trust and resilience
The success of frontier AI relies not only on its technical sophistication but also on the trust it garners from the public and stakeholders. Transparent safety practices play a critical role in building this trust. Developers must openly share insights into their methods for ensuring system safety, while policymakers need to foster regulatory environments that encourage and reward responsible innovation. Embedding accountability at every stage of the AI lifecycle - from research and development to deployment and monitoring - can help alleviate public fears and ensure that the benefits of frontier AI are equitably shared. Dynamic safety cases serve as a crucial tool in this endeavour, offering a way to continually validate and adapt safety measures in response to evolving risks.
The study presents a forward-thinking framework to meet these challenges. It acknowledges that frontier AI systems are not static; their capabilities and risks evolve over time, necessitating equally adaptive safety practices. By integrating tools like the Dynamic Safety Case Management System (DSCMS), Checkable Safety Arguments (CSAs), and Safety Performance Indicators (SPIs) into both technical workflows and governance structures, society can effectively balance innovation with safety. These mechanisms provide a robust foundation for mitigating risks while harnessing the transformative potential of frontier AI.
As these advanced systems continue to redefine technological possibilities, dynamic safety cases have the potential to become the cornerstone of a secure and resilient AI-driven future. They offer not just a way to safeguard AI systems but also a roadmap for fostering innovation that aligns with societal values and ethical priorities. In this rapidly advancing field, safety can no longer be viewed as a static certification - it must be a continuous commitment to adaptability, transparency, and trust. By embracing this approach, the benefits of frontier AI can be realized responsibly and sustainably.
- FIRST PUBLISHED IN:
- Devdiscourse