Future of industrial metaverse: Tackling counterfeit Digital Twins with AI
The industrial metaverse offers immense potential. By 2030, the global digital twin market is expected to reach $73.5 billion, with industries like manufacturing, energy, and healthcare leading the adoption curve. DTs allow businesses to optimize operations, test new strategies, and predict equipment failures - all without physical intervention. For example, a wind farm operator can use DTs to monitor turbine performance, identifying potential issues before they escalate into failures. Similarly, car manufacturers can simulate crash tests with DTs, accelerating the design process and reducing physical prototype costs.
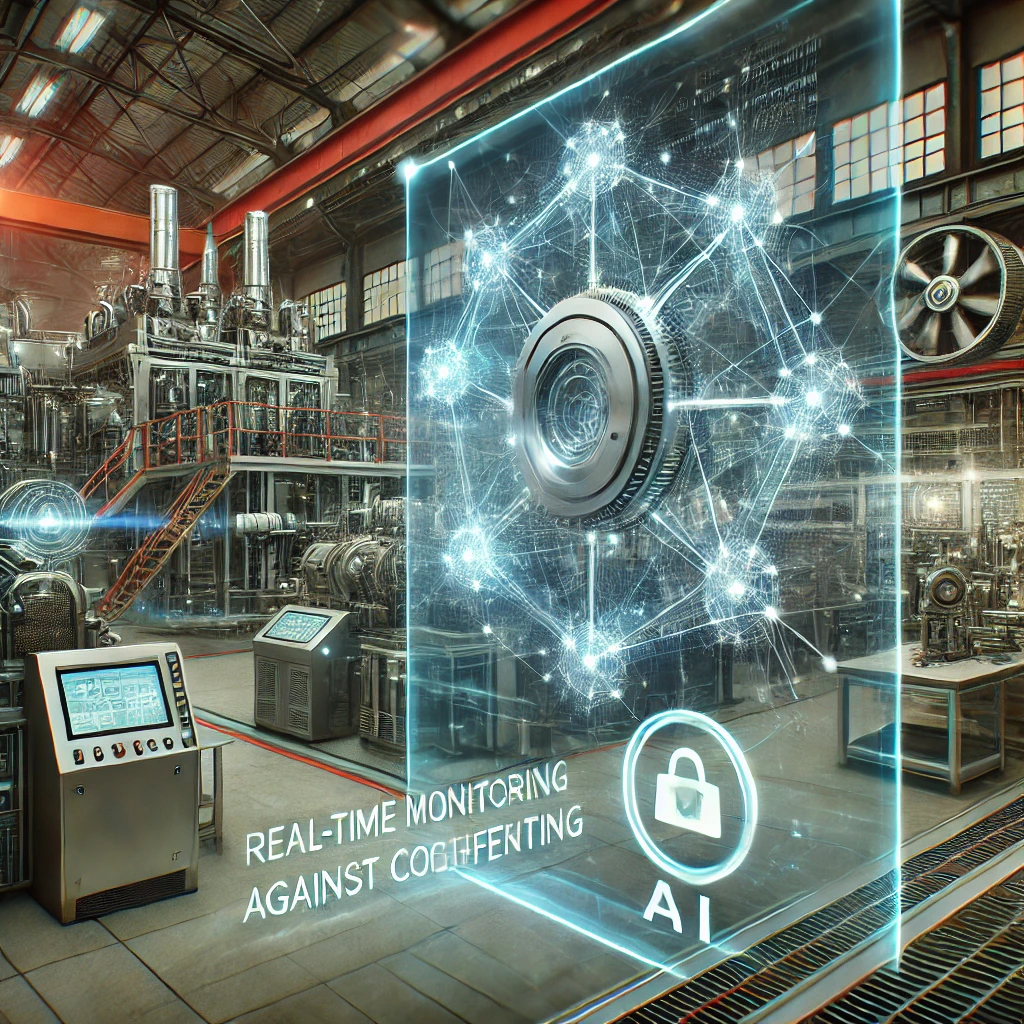
The industrial metaverse is emerging as a transformative force, merging the digital and physical worlds to revolutionize industries such as manufacturing, healthcare, and energy. From real-time process simulations to predictive maintenance, it enables businesses to improve efficiency, reduce costs, and innovate like never before. At the heart of this revolution are Digital Twins (DTs)—virtual replicas of physical assets that simulate their behaviour in the real world. But as industries integrate DTs into their operations, a critical question arises: How can we ensure the security and authenticity of these virtual entities?
A study titled "Towards Secure AI-driven Industrial Metaverse with NFT Digital Twins," currently available on arXiv, sheds light on the growing challenges of securing Digital Twins, particularly those authenticated using Non-Fungible Tokens (NFTs). Counterfeit DTs not only compromise operational efficiency but also pose severe risks of industrial espionage and process failures.
The study presents a novel solution to protect NFT-based DTs from counterfeiting, a vulnerability that could compromise the integrity of the industrial metaverse. By leveraging cutting-edge AI techniques and dynamic metadata, the proposed framework sets a benchmark for securing the future of digital twins.
The industrial metaverse: Opportunity meets risk
The industrial metaverse offers immense potential. By 2030, the global digital twin market is expected to reach $73.5 billion, with industries like manufacturing, energy, and healthcare leading the adoption curve. DTs allow businesses to optimize operations, test new strategies, and predict equipment failures - all without physical intervention. For example, a wind farm operator can use DTs to monitor turbine performance, identifying potential issues before they escalate into failures. Similarly, car manufacturers can simulate crash tests with DTs, accelerating the design process and reducing physical prototype costs.
However, the integration of DTs into business-critical operations comes with risks. NFTs, widely regarded as a solution for authenticating ownership and identity of digital assets, have become a popular choice for managing DTs. While NFTs ensure traceability and uniqueness, they are not immune to counterfeiting. Attackers can clone or manipulate NFT-based DTs, introducing vulnerabilities that undermine the entire metaverse ecosystem.
In the study, researchers highlight how such counterfeit clones could disrupt industrial processes. For example, a counterfeit DT of a factory's robotic arm might send inaccurate data, leading to costly inefficiencies or, worse, safety hazards.
Fighting counterfeiting with AI and dynamic metadata
The study proposes a cutting-edge solution to tackle counterfeit DTs, using AI-driven dynamic metadata and behavioural pattern recognition. Unlike static metadata traditionally used in NFTs, dynamic metadata evolves in real-time, tracking changes in the DT’s operational parameters. This ensures that any tampering attempt is immediately flagged.
Key to this innovation is the use of Denoising Autoencoders (DAE) and Bidirectional Gated Recurrent Units (Bi-GRU). These deep learning models analyze behavioural patterns of DTs, distinguishing genuine entities from fake clones with exceptional accuracy.
In their experiments, the researchers tested the proposed framework using a simulated industrial dataset involving heaters, and the results were remarkable. The system demonstrated an impressive 98.3% accuracy, effectively distinguishing between authentic and counterfeit digital twins with near-perfect precision. The integration of dynamic metadata and AI technologies provided robustness against tampering, making it virtually impossible for attackers to bypass detection mechanisms.
Additionally, the framework enhanced operational security by leveraging real-time updates and behavioural monitoring, which not only minimized false positives but also ensured effective mitigation of potential risks. These results underscore the framework’s potential to become a reliable solution for securing digital twins in the industrial metaverse.
Securing the future of digital twins
Ensuring the authenticity and security of Digital Twins is critical as the industrial metaverse scales. The implications of the proposed framework extend far beyond manufacturing. In healthcare, counterfeit DTs of medical devices could lead to diagnostic errors, while in urban planning, fake DTs of infrastructure assets might result in flawed city designs. Securing DTs with AI-driven solutions could help avoid such scenarios, making the metaverse a safer, more reliable space for innovation.
The industrial metaverse, with its promise of efficiency and innovation, is at a crossroads. While the opportunities are immense, the risks cannot be ignored. The study highlights how proactive security measures, such as dynamic metadata and AI-enhanced behavioural analysis, can serve as the foundation for a trustworthy metaverse. With global investments in metaverse technologies surging, addressing these challenges is not just necessary - it’s urgent.
By adopting advanced security frameworks, industries can ensure that the metaverse delivers on its promise: a seamlessly integrated world where the physical and digital coexist, driving progress and growth without compromising trust.
- FIRST PUBLISHED IN:
- Devdiscourse