A global call for equity: Fixing bias in AI-driven healthcare
As AI continues to shape the future of healthcare, ensuring its equitable application is paramount. The STANDING Together Consensus Recommendations offer a critical blueprint for tackling algorithmic bias and promoting transparency in health datasets. By embracing these guidelines, the healthcare community can build systems that uphold trust, inclusivity, and fairness.
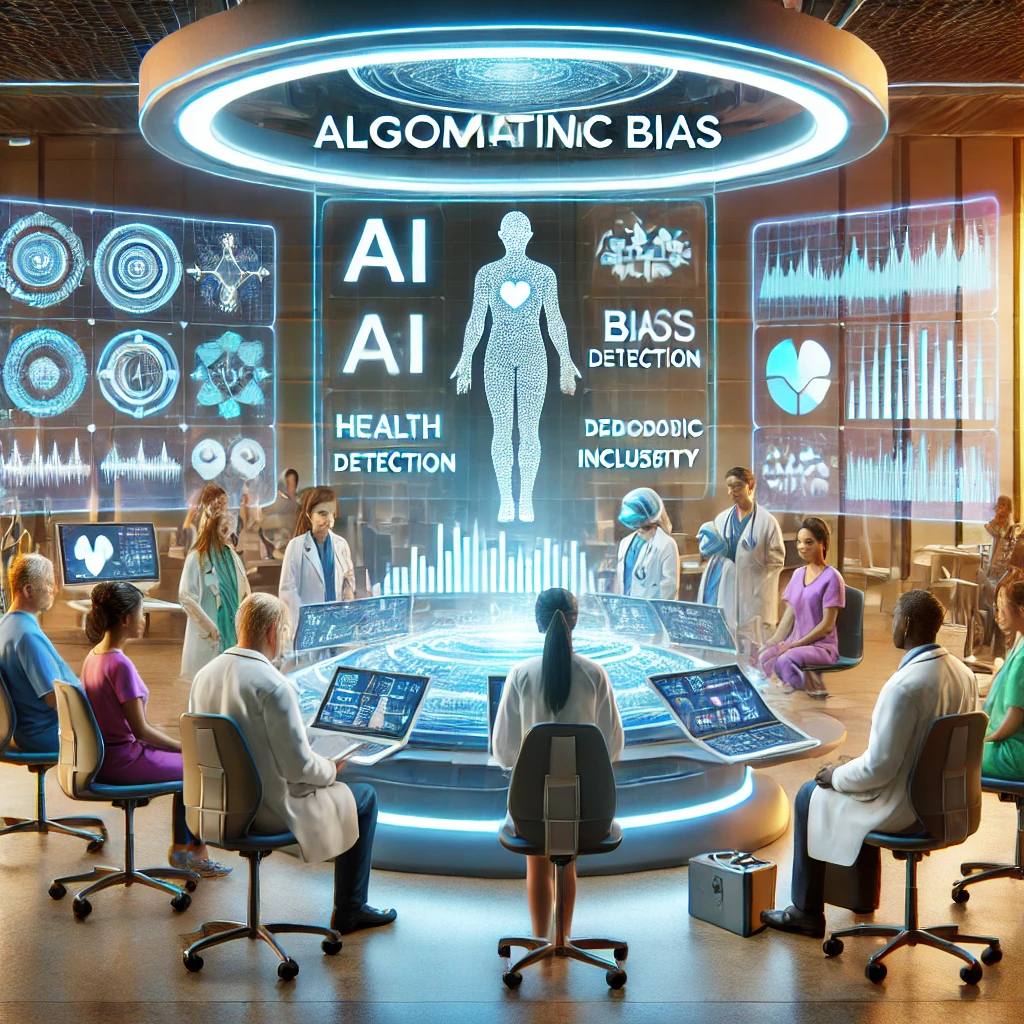
Artificial intelligence (AI) in healthcare is transforming patient care, diagnostics, and treatment outcomes. Yet, this revolution comes with a critical challenge: algorithmic bias embedded within health datasets. Such biases, if unchecked, risk exacerbating health inequities instead of reducing them. To address these pressing concerns, the study titled “Tackling Algorithmic Bias and Promoting Transparency in Health Datasets: The STANDING Together Consensus Recommendations”, published in The Lancet Digital Health, presents a comprehensive roadmap for building fairness, inclusivity, and transparency in AI health technologies.
Conducted by an international team of over 350 experts from 58 countries, the research highlights actionable solutions to mitigate algorithmic bias, improve data transparency, and ensure that AI health systems serve all populations equitably.
Algorithmic bias in healthcare
AI algorithms are only as unbiased as the data they are trained on. Unfortunately, many health datasets fail to represent diverse populations, resulting in systems that disproportionately favor certain demographics while disadvantaging others. This bias manifests in several ways:
- Underrepresentation of Marginalized Groups: Key populations, including racial minorities and individuals from low-income regions, are often excluded or underrepresented in datasets, leading to AI systems that fail to meet their specific needs.
- Embedded Historical Disparities: Existing inequities in healthcare access and outcomes are often reflected in training data, perpetuating systemic inequalities.
- Lack of Dataset Transparency: Without clear documentation on how datasets are collected, curated, and validated, stakeholders cannot fully assess their limitations or biases.
The implications are far-reaching. From algorithms misdiagnosing skin conditions in darker skin tones to biased allocation of healthcare resources, these disparities can erode trust in AI-driven healthcare systems and jeopardize patient safety.
The STANDING Together recommendations: A comprehensive framework
The STANDING Together initiative aims to tackle these challenges head-on through a set of 29 consensus recommendations targeting two key areas: documentation of health datasets and use of health datasets in AI systems. These guidelines were developed using the rigorous Delphi method, which involved multiple rounds of expert feedback to ensure broad applicability and consensus.
Documentation of Health Datasets
Transparent dataset documentation is at the core of these recommendations. The framework calls for clear and detailed records on:
- Data Origin and Collection Methods: Providing information on where and how data was collected ensures stakeholders can assess its relevance and limitations.
- Demographic Representation: Datasets should include details about the diversity of populations, particularly in terms of race, ethnicity, age, and gender.
- Limitations and Bias Awareness: Dataset curators must disclose potential biases or gaps, enabling developers to account for these shortcomings during AI model development.
This emphasis on transparency ensures that datasets meet ethical standards and can be evaluated critically by users and regulators alike.
Ethical Use of Health Datasets
For developers and users of AI systems, the recommendations provide practical strategies to identify and mitigate biases:
- Bias Testing Across Populations: Algorithms must be rigorously tested on diverse demographic groups to detect performance disparities.
- Documenting Model Limitations: Developers should publish detailed reports outlining where and why an algorithm may fail, fostering accountability.
- Stakeholder Involvement: Including clinicians, patient advocates, and policymakers in the development process ensures AI technologies align with real-world needs.
What sets the STANDING Together recommendations apart is the diversity of perspectives involved in their creation. The initiative included not only researchers but also healthcare providers, policymakers, and patient advocates, ensuring that the guidelines address the needs of a broad range of stakeholders. With contributions from 58 countries, the recommendations are designed to be adaptable across different healthcare systems and socio-economic contexts.
The use of the Delphi method for achieving consensus adds another layer of rigor. By iteratively refining the recommendations through expert feedback, the process ensured that the guidelines are both actionable and widely endorsed.
Implications for the future of AI in healthcare
Adopting the STANDING Together recommendations has profound implications for the future of AI-driven healthcare. These guidelines provide a clear framework for creating systems that are transparent, ethical, and equitable. By addressing algorithmic bias and improving dataset quality, AI technologies can deliver consistent and reliable care across diverse populations.
For healthcare organizations and developers, these recommendations underscore the importance of proactive bias mitigation. Regulators and funding bodies are also encouraged to enforce these standards, ensuring that ethical considerations remain central to AI innovation.
The path forward
As AI continues to shape the future of healthcare, ensuring its equitable application is paramount. The STANDING Together Consensus Recommendations offer a critical blueprint for tackling algorithmic bias and promoting transparency in health datasets. By embracing these guidelines, the healthcare community can build systems that uphold trust, inclusivity, and fairness.
This research is a call to action for developers, policymakers, and clinicians to prioritize equity and accountability in AI-driven healthcare. The promise of AI is immense, but its potential can only be realized through ethical and inclusive practices. With the implementation of these recommendations, the vision of equitable AI in healthcare becomes an achievable reality.
- FIRST PUBLISHED IN:
- Devdiscourse