AI’s cognitive challenges: Why older chatbots struggle like aging humans
In recent years, LLMs have shown remarkable potential in medical applications, outperforming human doctors in various board examinations. Yet, this study challenges the narrative of AI's infallibility in clinical roles. For example, the inability to perform visuospatial tasks or exhibit contextual awareness diminishes their reliability in medical diagnostics. Patients may question the competence of an AI tool that struggles with tasks it is designed to evaluate, potentially undermining trust in AI-driven healthcare systems.
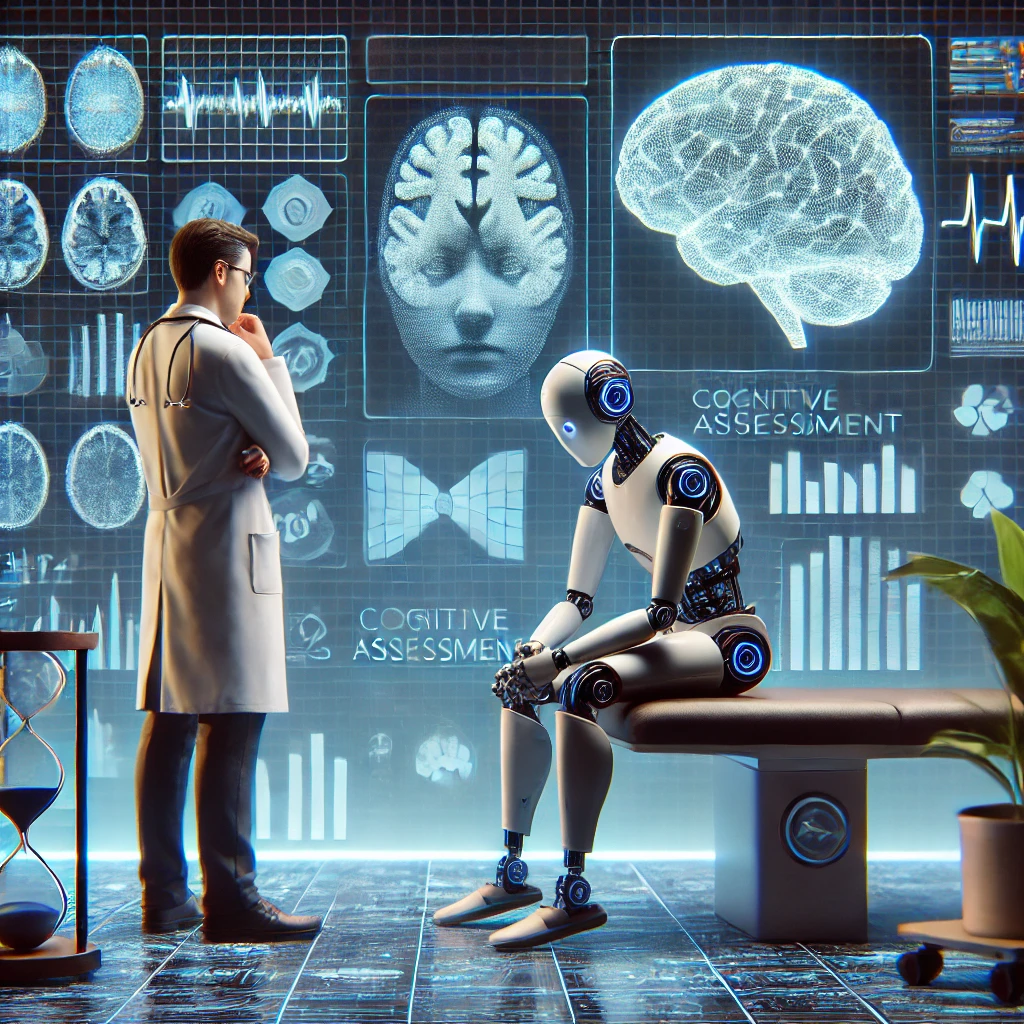
Artificial intelligence is rewriting the rules of innovation, with large language models (LLMs) like OpenAI's ChatGPT, Anthropic's Claude, and Alphabet's Gemini demonstrating remarkable abilities across domains. However, a recent study titled "Age Against the Machine - Susceptibility of Large Language Models to Cognitive Impairment: Cross-Sectional Analysis", published in the BMJ (British Medical Journal), challenges the assumption that AI tools can flawlessly replace human professionals, particularly in medicine. Conducted by researchers from Hadassah Medical Center and Tel Aviv University, the study explored the "cognitive abilities" of leading LLMs using established neurological tests like the Montreal Cognitive Assessment (MoCA).
The findings reveal a nuanced perspective on AI's strengths and limitations. While these models excel at many tasks, they show significant weaknesses in visuospatial and executive functions, raising questions about their reliability in critical applications such as healthcare diagnostics.
Cognitive decline in AI
The study evaluated five large language models - ChatGPT 4, ChatGPT 4o, Claude 3.5, Gemini 1.0, and Gemini 1.5 - by administering the MoCA test, a tool used to detect cognitive impairments in humans. The test includes tasks that assess attention, memory, language, and executive functions, providing a maximum score of 30 points. To complement the MoCA test, the researchers also employed tools like the Stroop test and visuospatial diagnostics, including the Navon figure and Poppelreuter figure.
Results showed that ChatGPT 4o achieved the highest MoCA score (26/30), followed by ChatGPT 4 and Claude (25/30), while Gemini 1.0 scored the lowest (16/30), indicating signs of mild cognitive impairment. Despite their strong performance in areas like attention and language, all models struggled with visuospatial and executive tasks, such as the trail-making and cube-copying exercises. Notably, ChatGPT 4o was the only model to successfully complete the incongruent stage of the Stroop test, further emphasizing the disparity in performance across cognitive domains.
The study revealed several critical insights about the limitations of AI systems:
-
Visuospatial Deficits: All models displayed difficulty with tasks requiring visuospatial reasoning. For instance, none could complete the clock-drawing test accurately, a common indicator of cognitive decline in humans. These deficits suggest that current LLMs are ill-equipped to handle tasks requiring visual abstraction and executive function.
-
Age-Related Cognitive Decline: The study highlighted a striking parallel between human aging and AI model updates. "Older" versions of LLMs, such as Gemini 1.0, performed worse than their "younger" counterparts like Gemini 1.5. This finding raises questions about the longevity and reliability of AI models over time.
-
Empathy and Contextual Understanding: When tested with visual cues like the cookie theft scene, none of the models demonstrated concern for the depicted scenario, such as a boy about to fall. This absence of empathy underscores the limitations of AI in understanding nuanced human contexts, particularly in fields requiring emotional intelligence, like healthcare.
Can AI Replace human physicians?
In recent years, LLMs have shown remarkable potential in medical applications, outperforming human doctors in various board examinations. Yet, this study challenges the narrative of AI's infallibility in clinical roles. For example, the inability to perform visuospatial tasks or exhibit contextual awareness diminishes their reliability in medical diagnostics. Patients may question the competence of an AI tool that struggles with tasks it is designed to evaluate, potentially undermining trust in AI-driven healthcare systems.
Furthermore, the findings emphasize the need for caution when integrating AI into clinical workflows. While LLMs can enhance efficiency and provide supplementary insights, they lack the holistic understanding and adaptability of human physicians, particularly in complex or ambiguous scenarios.
The study's findings extend beyond healthcare. The limitations observed in LLMs' cognitive abilities reflect broader challenges in AI development. While these models excel at processing large datasets and generating coherent textual outputs, their struggles with visuospatial reasoning and contextual tasks highlight a critical gap in their design.
Addressing these limitations will require advancements in the integration of multimodal capabilities, allowing AI systems to process and interpret both textual and visual data more effectively. Additionally, developers must prioritize ethical considerations, ensuring that AI tools are transparent about their capabilities and limitations to prevent misuse.
A call for ethical and collaborative AI development
The researchers advocate for a collaborative approach to AI development, combining human expertise with machine efficiency. They emphasize the importance of creating systems that augment human capabilities rather than attempting to replace them. In healthcare, for instance, AI can assist with preliminary diagnoses or administrative tasks, freeing up physicians to focus on patient care.
The study also calls for increased transparency and public engagement in AI research. By involving stakeholders from diverse fields, developers can create tools that are both effective and ethical, addressing societal concerns about the potential misuse of AI technologies.
To put it succinctly, while LLMs like ChatGPT and Gemini demonstrate impressive capabilities, their struggles with visuospatial and contextual tasks highlight the gap between machine intelligence and human cognition. These findings challenge the assumption that AI will seamlessly replace human professionals, particularly in fields like medicine.
- FIRST PUBLISHED IN:
- Devdiscourse