Generative AI and the future of creative problem-solving: A paradigm shift
By embracing human-AI collaboration, organizations can unlock new levels of innovation and efficiency, paving the way for a future where creative problem-solving is accessible to all. While challenges remain, the research highlights the transformative possibilities of generative AI, setting the stage for a new era of innovation.
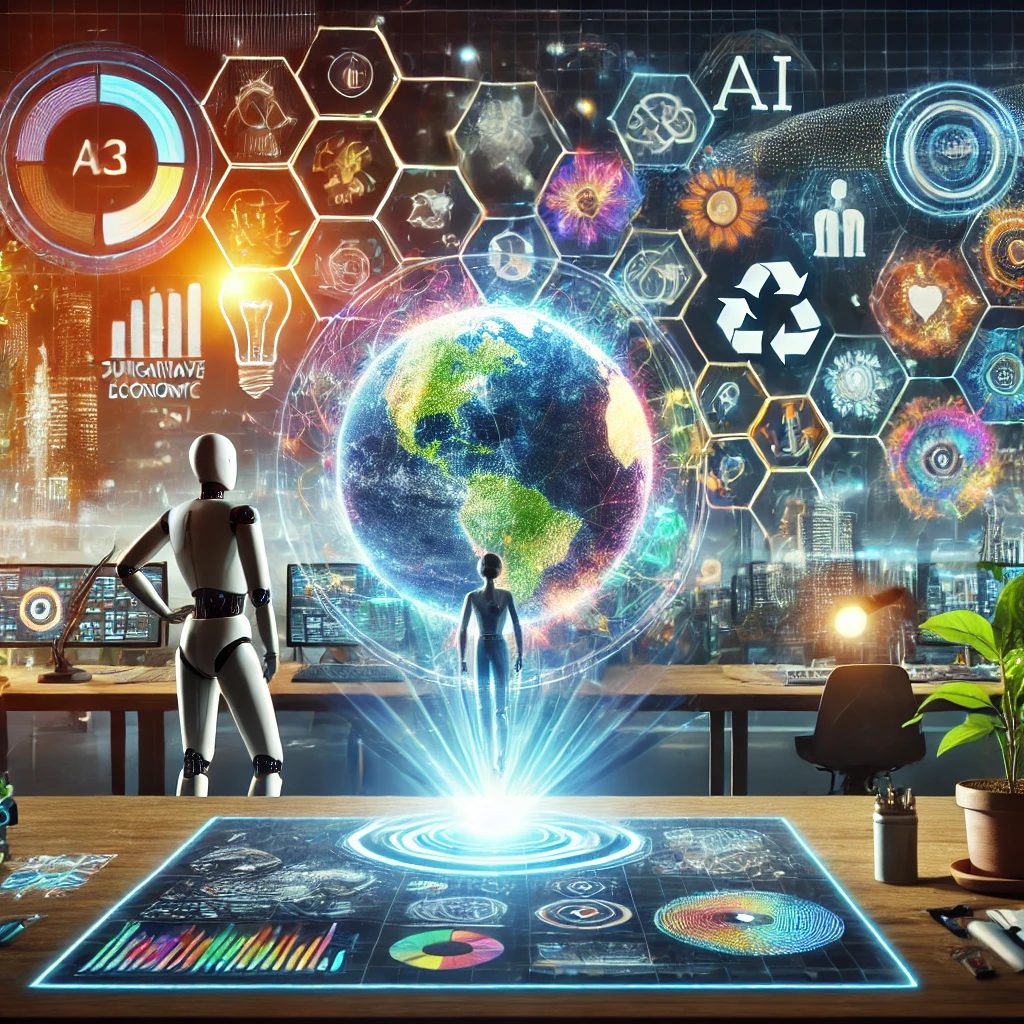
In recent years, artificial intelligence (AI) has become a central force in transforming how we approach complex challenges. Generative AI, in particular, is reshaping industries by enabling unprecedented collaboration between human creativity and machine intelligence. A recent study titled "The Crowdless Future? Generative AI and Creative Problem-Solving" by Léonard Boussioux, Jacqueline N. Lane, Miaomiao Zhang, Vladimir Jacimovic, and Karim R. Lakhani, published in Organization Science, delves deeply into this transformative potential. The paper explores how generative AI compares to traditional crowdsourcing methods in creative problem-solving and highlights the advantages of this groundbreaking technology in terms of efficiency, scalability, and innovation.
The study provides a detailed examination of human-AI collaboration in solving strategic problems. Using generative AI models such as large language models (LLMs), researchers investigated how AI could complement and even surpass human ingenuity in creating innovative solutions. The research offers compelling evidence that human-AI partnerships are not just cost-effective but also capable of producing high-quality outputs that rival or outperform those generated by traditional crowdsourcing.
A revolution in creative problem-solving
Creative problem-solving has always been a cornerstone of progress in various fields, from business strategy to scientific discovery. Traditional approaches often rely on crowdsourcing, where diverse human contributors are tasked with generating ideas or solving problems. While effective, this method has significant limitations. Crowdsourcing can be time-consuming, expensive, and difficult to manage, especially when dealing with large-scale or open-ended challenges.
Generative AI introduces a game-changing alternative. With its ability to process and analyze vast amounts of data, generate diverse solutions, and adapt to iterative feedback, AI offers a scalable and efficient way to approach complex problems. In this study, researchers compared two distinct methods of human-AI collaboration: independent search, where AI generates solutions autonomously based on initial prompts, and differentiated search, where humans guide the AI iteratively to refine and expand its outputs. These approaches were tested against traditional human crowdsourcing methods in a challenge focused on generating sustainable business ideas for the circular economy.
Human-AI collaboration: Key insights
The research was designed to evaluate the effectiveness of generative AI in producing creative solutions compared to human crowds. A total of 234 solutions were analyzed, with metrics such as novelty, strategic viability, environmental impact, financial value, and overall quality used to assess their effectiveness. The findings were striking. While human-generated solutions exhibited slightly higher average novelty, the solutions created through human-AI collaboration excelled in other critical areas, including strategic viability and overall quality.
The differentiated search method, which involved iterative human guidance, emerged as particularly effective. This approach allowed researchers to leverage AI's computational power while incorporating human creativity and strategic thinking. The results highlighted the unique strengths of human-AI partnerships: humans can guide AI to explore diverse solution spaces, while AI provides rapid, cost-effective generation of high-quality ideas. These findings underscore the complementary nature of human and machine intelligence in addressing complex challenges.
The generative AI advantage
One of the most compelling aspects of generative AI is its cost-effectiveness. In this study, the average cost per AI-generated solution was just $0.037, a fraction of the expense associated with traditional crowdsourcing methods. This affordability opens up new possibilities for organizations and researchers, particularly those with limited resources. Additionally, the scalability of generative AI means that it can be applied to a wide range of problems, from early-stage ideation to detailed strategic planning.
The study also emphasizes the speed with which AI can generate and refine solutions. Traditional crowdsourcing often involves logistical challenges, such as recruiting and managing participants, which can delay the problem-solving process. In contrast, AI can produce a large volume of ideas in a fraction of the time, enabling faster decision-making and innovation.
Implications for organizations and innovation
The implications of this research are far-reaching. By integrating generative AI into their workflows, organizations can enhance their innovation capacity and improve the quality of their outputs. The study highlights several key areas where AI can make a significant impact, including sustainable development, product design, and business strategy. For instance, in the context of the circular economy, AI-generated solutions demonstrated a high level of strategic viability, offering practical and scalable ideas for reducing waste and promoting sustainability.
Moreover, generative AI can democratize access to creative problem-solving tools. By reducing costs and eliminating the logistical barriers associated with traditional methods, AI makes it possible for a broader range of organizations, including small businesses and non-profits, to tackle complex challenges. This democratization of innovation has the potential to accelerate progress across industries and drive more inclusive growth.
Challenges and limitations
While the study paints a promising picture of human-AI collaboration, it also acknowledges certain challenges. One significant limitation is the dependence on human skill in crafting effective prompts and guiding AI systems. Poorly designed prompts can lead to suboptimal outputs, highlighting the need for training and expertise in working with generative AI. Additionally, the potential biases embedded in AI training data can influence the quality and diversity of the solutions generated. Addressing these issues will be critical to maximizing the benefits of AI in creative problem-solving.
The research also notes that while generative AI excels in certain aspects, such as scalability and strategic value, it may not fully replace human crowdsourcing in scenarios where extreme novelty or highly contextual knowledge is required. Instead, the authors advocate for a hybrid approach that combines the strengths of human and machine intelligence to achieve the best results.
Toward a crowdless future?
The study's title itself poses an intriguing question about the role of crowdsourcing in a world increasingly influenced by AI. While the findings suggest that AI can serve as a powerful alternative to human crowds, the authors stop short of declaring the end of crowdsourcing. Instead, they envision a future where human-AI partnerships become the norm, enabling more efficient and effective problem-solving across a wide range of domains.
Overall, Generative AI has the potential to complement human ingenuity, offering scalable, cost-effective, and high-quality solutions to complex challenges. By embracing human-AI collaboration, organizations can unlock new levels of innovation and efficiency, paving the way for a future where creative problem-solving is accessible to all. While challenges remain, the research highlights the transformative possibilities of generative AI, setting the stage for a new era of innovation.
- FIRST PUBLISHED IN:
- Devdiscourse