Harnessing AI and ML to Enhance Space Domain Awareness Amid Rising Orbital Challenges
The RAND report highlights how AI/ML can revolutionize the U.S. Space Force's Space Domain Awareness by enhancing sensor tasking, data integration, and orbit determination. These technologies address the challenges of increasing space activity and aging systems, enabling more accurate, efficient, and responsive space operations.
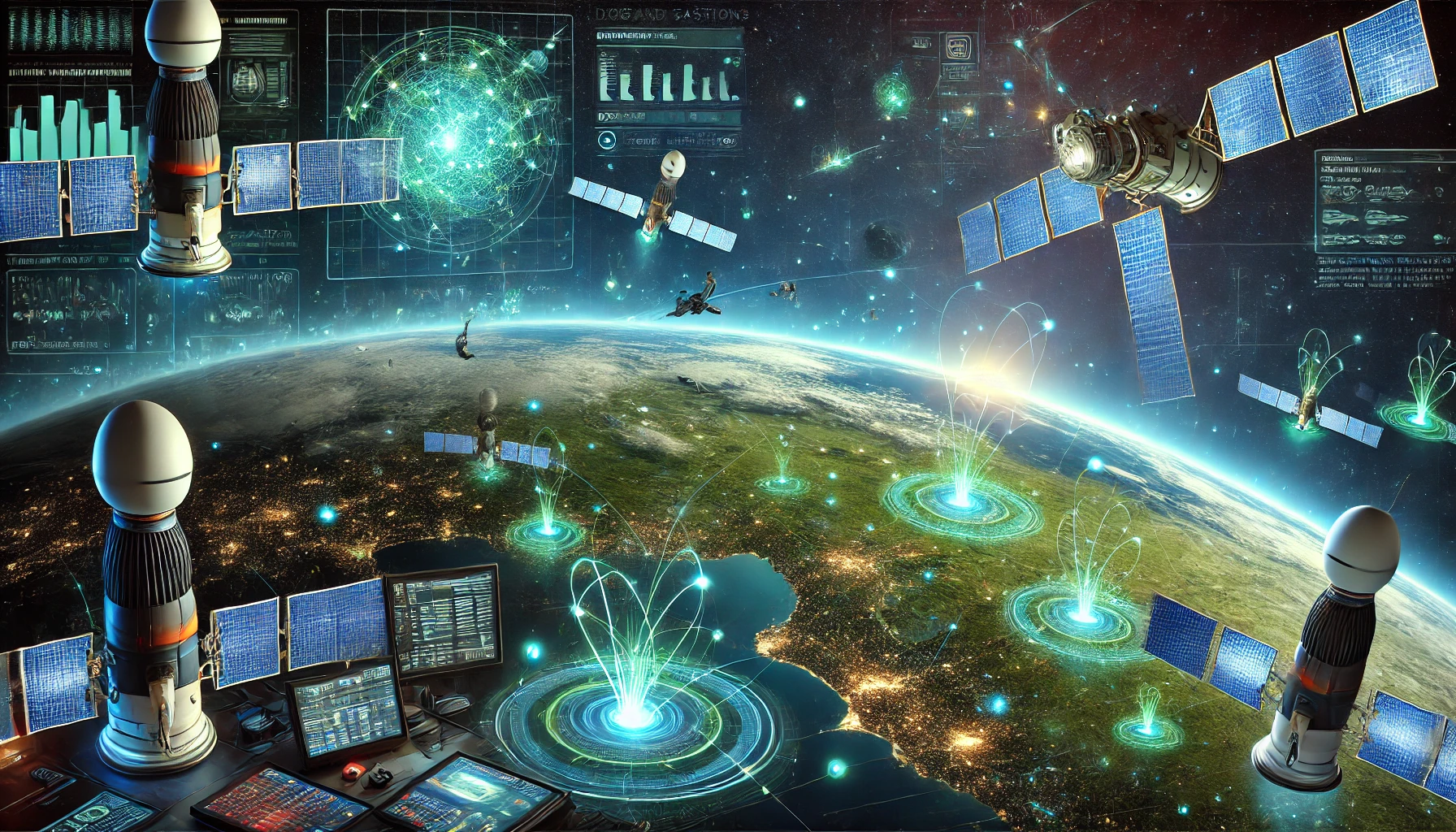
The RAND Corporation’s report explores how artificial intelligence (AI) and machine learning (ML) can transform the Space Domain Awareness (SDA) mission of the U.S. Space Force (USSF). This mission, critical for ensuring the safety of orbital activities, involves monitoring space objects and assessing potential threats. Researchers highlight the pressing need for AI/ML solutions to address the growing complexity of space operations, from tracking mega-constellations to identifying maneuverable and non-cooperative satellites. Existing systems like the Space Defense Operations Center (SPADOC) were not designed to cope with today’s dynamic space environment, making them insufficient for the modern warfighting domain. AI/ML offers opportunities to enhance SDA operations, optimize sensor tasking, improve data integration, and refine orbit determination processes.
Legacy Systems Struggle with Modern Demands
The SDA mission has evolved significantly with the exponential increase in space activities. Satellite launches occur with unprecedented frequency, mega-constellations deploy hundreds of satellites at once, and non-cooperative operators introduce new challenges. Designed decades ago, SPADOC remains the backbone of SDA but was built primarily for cataloging space objects rather than supporting today’s operational tempo and complexity. This aging system struggles to keep pace with the growing number of satellites and debris, limiting its ability to deliver timely and accurate situational awareness. The shift from a relatively static environment to a contested and congested space domain necessitates the use of more advanced tools. AI/ML technologies can bridge this gap by automating repetitive tasks, identifying patterns in vast datasets, and providing predictive insights, enabling SDA to address these challenges more effectively.
Enhancing Sensor Tasking and Observation Efficiency
A critical area where AI/ML can make a profound impact is in optimizing sensor tasking and utilization. SDA relies on a network of ground- and space-based sensors to monitor space objects, but these resources are limited and often overwhelmed. AI/ML tools can dynamically prioritize sensor observations, ensuring high-interest objects are adequately tracked while maintaining overall situational awareness. For instance, an AI-driven tasking system could allocate sensors more efficiently during events like satellite maneuvers or fragmentation incidents, which require rapid response. Higher revisit rates enabled by AI/ML tools would reduce the likelihood of losing track of objects, thereby mitigating the problem of uncorrelated tracks. By automating sensor calibration and tasking, AI/ML can also improve data quality and free up analysts to focus on higher-priority tasks.
Revolutionizing Data Integration and Orbit Determination
Data integration and orbit determination are foundational to SDA operations, yet current systems face significant limitations. SPADOC’s outdated architecture hampers the ability to process and fuse data from diverse sources, including commercial and international sensors. AI/ML can revolutionize this process by enabling more seamless integration and real-time analysis of vast datasets. Neural networks, for example, have shown promise in rapidly approximating complex orbital models, allowing for more frequent updates to orbital records. Such improvements are critical for maintaining the High Accuracy Catalog (HAC), which underpins many SDA processes, including collision avoidance. By improving orbit determination accuracy and timeliness, AI/ML tools can enhance predictive capabilities, reducing the risk of collisions and enabling more effective decision-making.
Overcoming Implementation Barriers
Despite their potential, implementing AI/ML solutions for SDA is not without challenges. Legacy systems, such as SPADOC, lack the computational power and flexibility required to support modern AI/ML applications. Moreover, the development of effective tools hinges on the availability of high-quality training data, which is often limited due to non-cooperative operators and the lack of standardized datasets. Human expertise is another critical factor; the technical nature of SDA tasks demands skilled personnel to develop, validate, and operate AI/ML tools. However, the USSF faces constraints in retaining such expertise, as personnel often transfer before mastering the complexities of SDA operations. Addressing these barriers will require investments in infrastructure modernization, data management, and workforce development. The deployment of the Advanced Tracking and Launch Analysis System (ATLAS) is a promising step toward enabling AI/ML integration, though its success depends on aligning tool development with future architectural capabilities.
Paving the Way for a Modernized SDA Mission
The RAND report emphasizes that while AI/ML tools cannot solve all the challenges of the SDA mission, they serve as invaluable force multipliers. These technologies can automate routine tasks, such as sensor calibration and uncorrelated track processing, allowing analysts to concentrate on strategic priorities. By increasing the responsiveness and accuracy of SDA processes, AI/ML tools can address the demands of a more contested and congested space domain. The report also highlights the importance of aligning tool development with the anticipated modernization of SDA infrastructure, ensuring that these technologies remain relevant and impactful in the long term. Building high-quality datasets, fostering collaboration with commercial and international partners, and investing in test and validation platforms will be crucial for realizing the full potential of AI/ML in SDA. With these advancements, the USSF can maintain a strategic edge, ensuring the security and sustainability of orbital operations in an increasingly complex environment.
- READ MORE ON:
- artificial intelligence
- machine learning
- Space Domain Awareness
- SDA
- SPADOC
- FIRST PUBLISHED IN:
- Devdiscourse