The Invisible Hand of AI: Addressing Market Concentration Risks in Generative AI
The paper explores how economies of scale and competition dynamics in the foundation model market favor dominant players like OpenAI and Google DeepMind, raising concerns about market concentration and equitable access. It advocates for proactive policies to balance innovation, competition, and societal benefits in the evolving AI landscape.
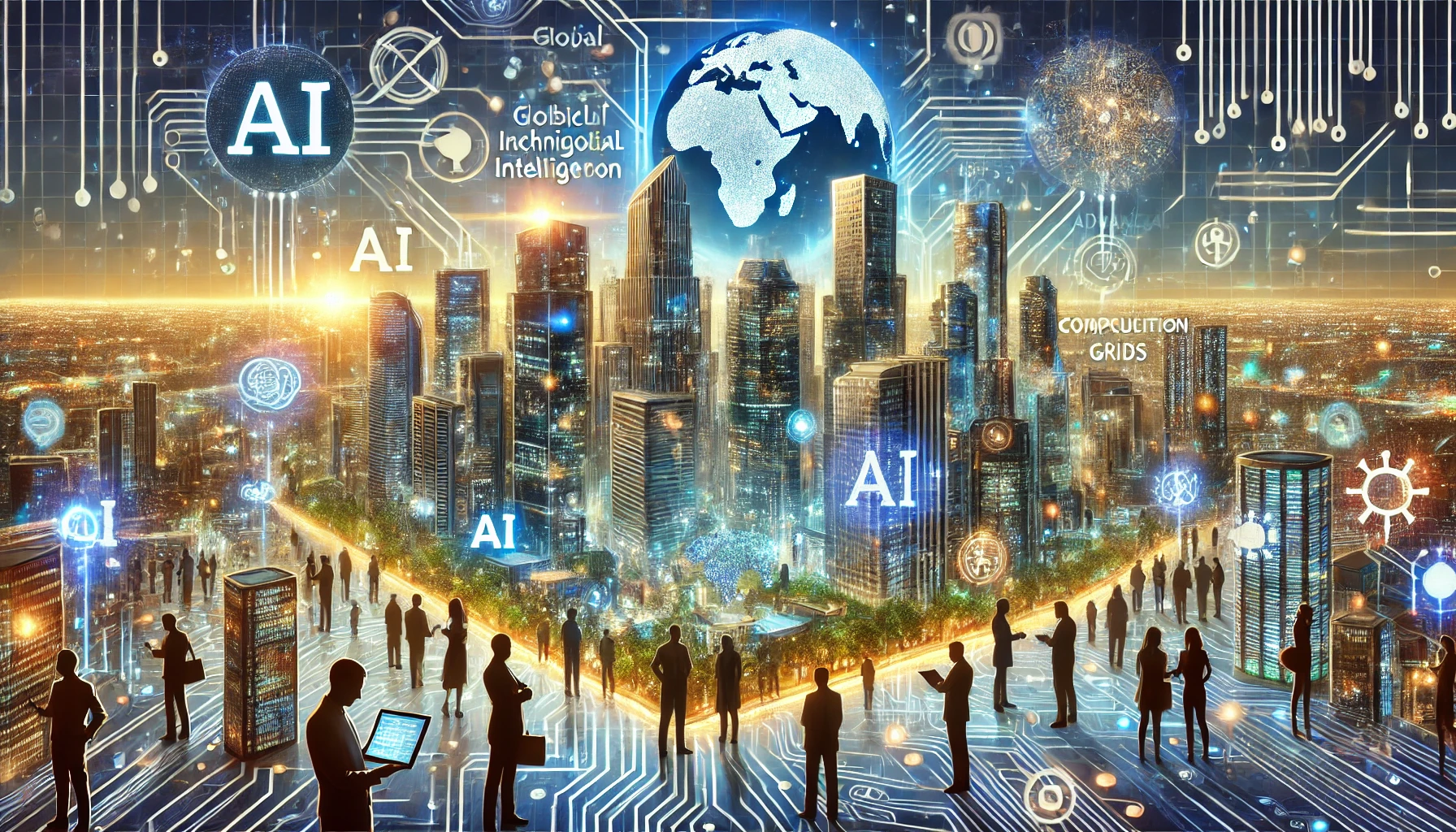
The National Bureau of Economic Research paper authored by Anton Korinek from the University of Virginia and Jai Vipra from Cornell University, explores the rapidly evolving market for artificial intelligence (AI), particularly foundation models like large language models (LLMs). These models, which underpin much of today’s generative AI technology, have sparked fierce competition due to their transformative potential across industries. The study examines the technological characteristics and market dynamics that are shaping this sector, highlighting the interplay of scaling, costs, and market power. With advancements in computational power and deep learning techniques, foundation models have demonstrated significant economic utility. However, their development involves immense costs related to computing infrastructure, data acquisition, and skilled labor. These factors create significant barriers to entry, favoring early leaders such as OpenAI and Google DeepMind, and laying the groundwork for market concentration.
Costs, Competition, and Economies of Scale
The authors analyze the cost structure of developing foundation models, emphasizing three critical components: pre-training expenses, fine-tuning costs, and variable operational costs. Pre-training, the most resource-intensive stage, requires massive computational power, which has driven up investment in hardware like GPUs. Companies like Nvidia dominate this space, with an estimated 98% market share in data-center GPUs, creating a bottleneck for newer entrants. Fine-tuning, while less expensive, often requires proprietary data, giving an edge to firms with extensive datasets. Variable costs, like inference expenses, are relatively low but become significant at scale. The convergence of these factors creates economies of scale, enabling major players to maintain a competitive edge. Additionally, the versatility of these models usable across sectors from healthcare to education offers economies of scope, further entrenching the dominance of leading firms.
The Risks of Tipping and Integration
The paper identifies two significant risks that threaten competition in this burgeoning market. The first is market tipping, where competition gradually narrows to a few dominant players due to their ability to leverage economies of scale, data feedback loops, and user inertia. These dynamics echo those observed in digital platforms, where first-mover advantages have often led to monopolistic outcomes. The second risk is vertical integration, where AI firms merge with upstream or downstream businesses, consolidating control over critical inputs like data and computing or embedding their models into widely used applications. For instance, Microsoft’s partnership with OpenAI and its exclusive provision of cloud services exemplifies how such alliances can reshape market dynamics. Similarly, Google DeepMind’s use of its proprietary TPU chips demonstrates the competitive advantage conferred by in-house infrastructure.
Policies for Fair AI Competition
As AI becomes increasingly embedded across industries, concerns about power concentration and its implications for innovation and fairness are growing. Policymakers are urged to take proactive measures to address these risks. The authors propose several strategies, including enforcing data-sharing mandates, fostering open-source AI development, and ensuring interoperability standards to reduce switching costs for users. They also advocate for scrutinizing mergers and acquisitions that could limit competition and implementing regulations akin to those for public utilities to prevent discriminatory practices in access to foundational AI systems. However, such measures must be balanced with the need to safeguard AI systems against potential misuse or safety risks, especially as models become more advanced and capable.
Balancing Innovation, Safety, and Societal Benefits
The paper also explores the broader societal implications of AI’s rise. As these systems gain the capacity to perform a growing range of cognitive tasks, they could significantly disrupt labor markets and reshape economic structures. This potential concentration of economic and technological power raises questions about equitable access and the distribution of benefits. If not managed carefully, the advantages of AI could accrue disproportionately to a few firms or regions, exacerbating inequality. The authors emphasize the need for international cooperation to develop regulatory frameworks that balance competition, safety, and societal welfare.
The paper offers a comprehensive analysis of the intersection between AI innovation and market dynamics. It underscores the urgency of addressing the competitive and ethical challenges posed by foundation models to harness their transformative potential responsibly. By balancing regulation with innovation, policymakers can create an environment where AI advances serve a broad spectrum of societal needs while minimizing risks of concentration and misuse. As the field continues to evolve, the insights from this paper provide a roadmap for fostering a competitive and equitable AI landscape.
- FIRST PUBLISHED IN:
- Devdiscourse