Efficient Agriculture Surveys: Machine Learning’s Role in Accurate Yield Estimations
The World Bank study demonstrates the effectiveness of machine learning in filling agricultural data gaps by imputing missing crop yield data, especially when integrating geospatial variables. While within-survey imputations proved accurate, cross-year extrapolations were less reliable, highlighting the need for high-quality, consistent data collection.
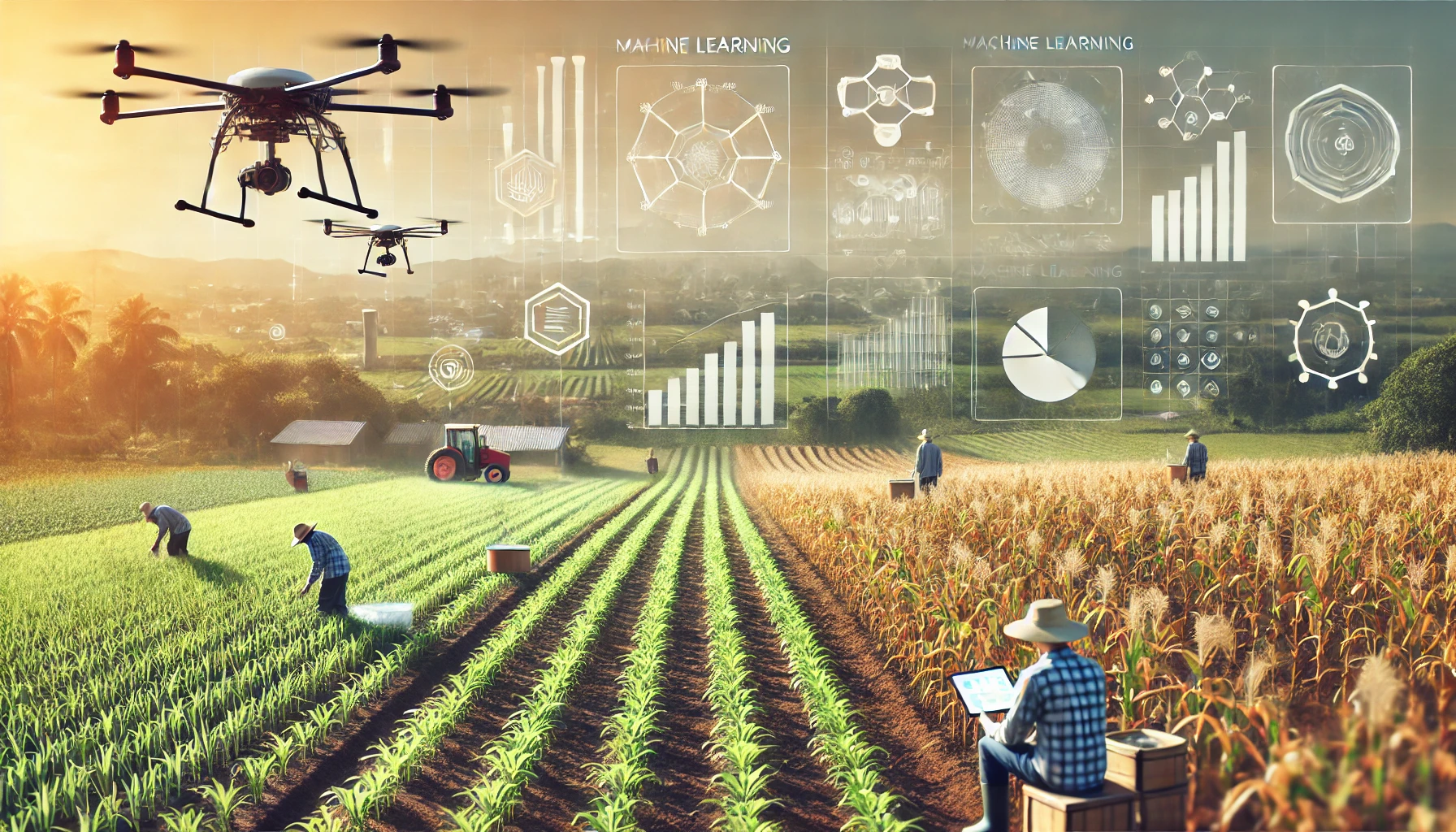
A recent working paper by Ismaël Yacoubou Djima, Marco Tiberti, and Talip Kilic from the World Bank’s Development Data Group presents a compelling case for using machine learning to fill data gaps in agricultural yield measurements. This research addresses a common issue in large-scale agricultural surveys where crop-cutting, the most accurate yield measurement method, is often constrained by costs, making comprehensive data collection challenging in low-resource settings. Leveraging machine learning techniques, the authors developed multiple imputation models to predict missing crop yield data, using survey data from Mali as a primary case study. In Mali, the Living Standards Measurement Study - Integrated Surveys on Agriculture (LSMS-ISA) project gathered data including crop-cut and self-reported yield figures across multiple crop types, providing a robust dataset for testing the models. This approach is particularly valuable in agricultural studies where obtaining objective crop yield data at a granular level is crucial for understanding smallholder productivity but often remains incomplete due to cost constraints.
Machine Learning Bridges Agricultural Data Gaps
The research demonstrates that machine learning-driven imputations are effective, especially in scenarios where crop yield data is partially or fully missing. For instance, in cases where only a subset of plots includes crop-cutting data, the authors applied within-survey imputation, predicting the missing values using other data points within the same survey. Another approach tested was survey-to-survey imputation, where the model trained on one year’s data was used to predict values in another. The study’s findings indicate that within-survey imputations are generally accurate, providing strong estimates that closely align with actual crop-cut yields. However, survey-to-survey imputations where yield data from one survey round is extrapolated to another showed limitations, largely due to inconsistencies across survey years. This outcome underscores the challenges in relying on cross-survey extrapolation for accurate data, as differences in conditions, sample representativeness, and survey design can introduce errors that affect prediction reliability.
Geospatial Data Enhances Yield Prediction Accuracy
A core aspect of this study involved analyzing the predictors that contribute most to accurate yield imputation. The authors found that integrating geospatial variables, such as rainfall patterns and elevation, with farmer-reported yields significantly improved the accuracy of machine learning predictions. Although self-reported yields alone can provide some predictive power, their utility is limited by non-classical measurement errors, a well-documented issue where farmers overestimate yields, especially on smaller plots. Geospatial data, by contrast, provided more objective environmental insights and proved particularly valuable for crops like millet, sorghum, and groundnut, which are sensitive to specific regional and climatic conditions. This finding suggests that including standardized geospatial information when combined with self-reported yields, offers a more comprehensive approach to crop yield estimation. Such integrated data allowed machine learning models to account for the non-linear interactions between various environmental and management factors that impact crop productivity.
Optimizing Sample Size for Cost-Efficiency
The study also delves into the optimal sample size for crop-cutting to ensure cost-effective data collection without compromising data quality. By conducting simulations with different training sample sizes, the authors discovered that crop-cutting on half of the plots provided reasonably high prediction accuracy, especially for within-survey imputation. For most crops, further increasing the sample size yielded only marginal gains in accuracy. The authors suggest that a sample size of one-third to one-half of the surveyed plots might offer a balanced approach, where significant cost savings could be achieved while maintaining reliable crop yield predictions. This finding holds substantial implications for agricultural data collection, particularly in regions where logistical constraints and funding limit the scope of comprehensive crop-cutting.
Crop-Specific Insights on Prediction Accuracy
One of the study’s more nuanced findings is the variation in prediction accuracy across different crops. The machine learning models performed better with crops that have low intercropping rates and are more commercially oriented. Rice and groundnut, for instance, yielded more accurate predictions, likely due to the higher standardization in measurement practices associated with these cash crops. Conversely, crops commonly intercropped or cultivated on smaller plots, such as cowpea, presented greater imputation challenges. These nuances highlight the importance of crop-specific considerations in survey design and data modeling, as yield prediction accuracy may vary significantly depending on the crop type and cultivation practices.
Improving Survey Efficiency with Machine Learning
Another key takeaway is the study's validation of machine learning as a tool for improving agricultural survey efficiency. Within-survey imputation results demonstrated that machine learning models can effectively replace missing data within the same survey, a crucial finding for agricultural surveys constrained by budget and time. However, the results indicate caution when using these models across different survey rounds, as the survey-to-survey imputation showed decreased reliability. For accurate regional estimates, the study found that machine learning models struggled to consistently provide robust results, likely due to variations in regional environmental factors and survey sample sizes that complicate cross-regional imputation.
Overall, the research adds valuable insights into the potential for machine learning to enhance agricultural data collection and yield estimation, especially in low-resource settings. The study emphasizes the need for high-quality geospatial data, standardized measurement practices, and strategic sample size planning to maximize the effectiveness of imputation models. As machine learning continues to gain traction in economic research, this study contributes a practical framework for implementing data-driven solutions to bridge the agricultural data gap, especially in countries where comprehensive crop-cutting remains logistically challenging.
- FIRST PUBLISHED IN:
- Devdiscourse