Scalable AI-Driven 3D Model Reconstruction from Smartphone Images for Industry 4.0
Researchers from Wideverse and SisInfLab at Politecnico di Bari developed a scalable cloud-native pipeline for 3D model reconstruction using smartphone images, leveraging AI and machine learning for efficient and customizable models. The system integrates low-end hardware with high-end cloud resources, enhancing industrial applications like Digital Twins and augmented reality.
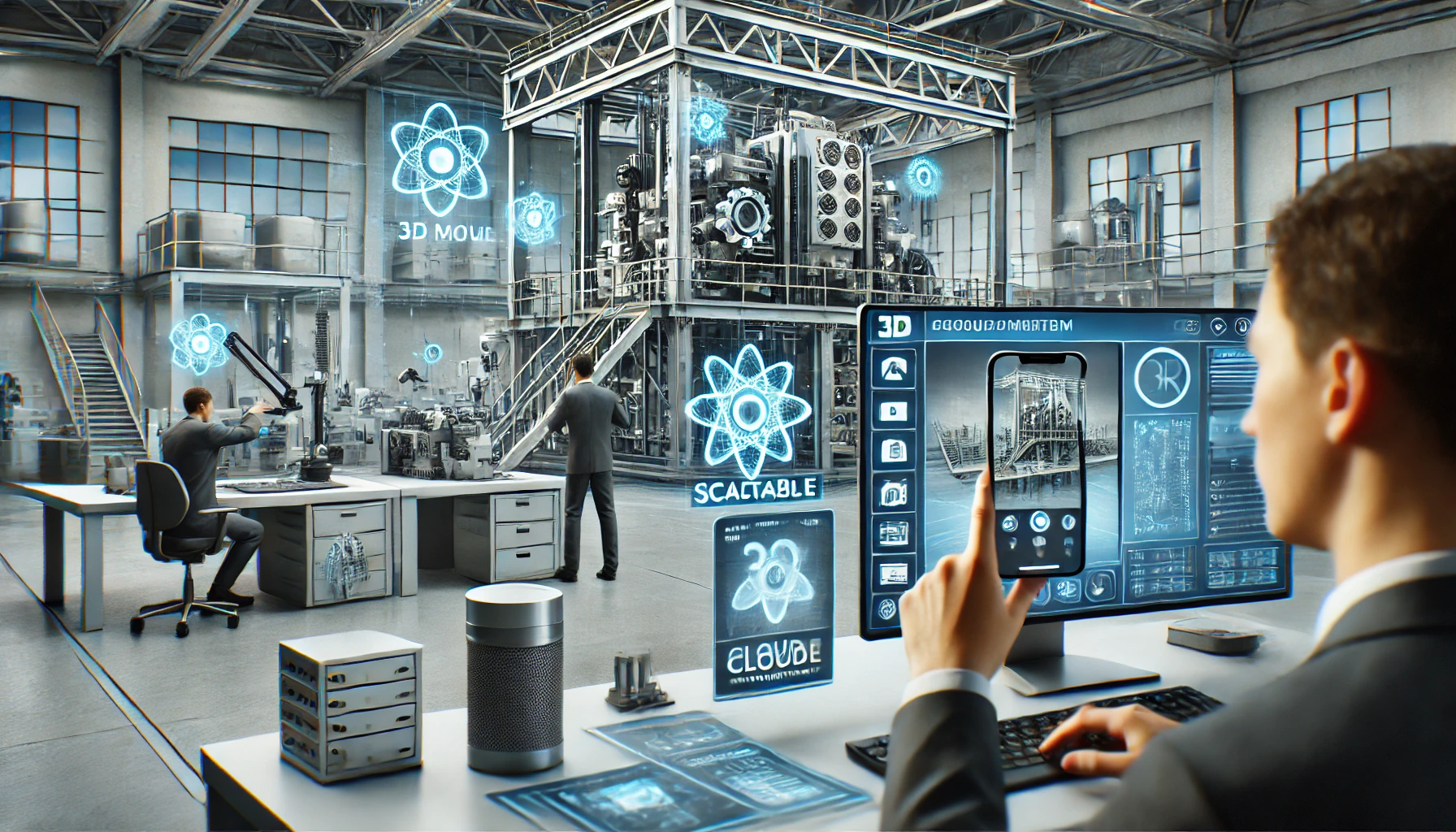
Researchers from Wideverse and SisInfLab at Politecnico di Bari have developed a novel cloud-native pipeline to efficiently reconstruct 3D models using 2D images captured by smartphone cameras. The project addresses the growing need for scalable and cost-effective 3D model creation across various industries like entertainment, manufacturing, and simulation. Traditional methods of creating 3D models, which often involve manual techniques, are resource-intensive and impractical for large-scale industrial applications. In response to this challenge, the researchers implemented a system that leverages advanced artificial intelligence (AI) and machine learning (ML) techniques, particularly those developed by NVIDIA, such as Instant NeRF and nvdiffrec. These models are capable of automatically generating reusable and customizable 3D models, complete with embedded materials and textures, that can be exported into external 3D modeling software or engines. The pipeline is designed to be adaptable to different use cases and can operate at scale, meeting Industry 4.0 standards for creating Digital Twin (DT) models. These DT models, which serve as virtual replicas of physical objects, are increasingly important in industries aiming to enhance productivity, particularly in worker training and operational efficiency.
A Modular Architecture for Flexibility and Scalability
One of the key innovations of this pipeline is its microservices architecture, which allows each component of the system to function independently. This modular design ensures that each part of the pipeline can be replaced or updated without disrupting the entire system, making it highly scalable and flexible for various industrial applications. The pipeline uses a custom-designed pose recorder based on Google’s ARCore framework to capture accurate camera poses during image acquisition, which is essential for creating precise 3D models. The reconstruction process starts with capturing a series of monocular 2D images using a smartphone camera. These images are then fed into the pipeline, where a machine learning model processes them to create a 3D model. The ARCore framework is critical in ensuring that the images and their associated camera poses are accurately recorded, which directly impacts the quality of the final 3D reconstruction. The system supports seamless interaction between the user and the generated 3D models, allowing users to view the models from various angles on their smartphones. The data flow between different components of the pipeline is managed using a high-performance storage system called MinIO, which is compatible with the S3 protocol and caches all intermediate outputs, ensuring efficient performance across different stages of the workflow.
Efficient Data Acquisition and Processing
The pipeline is divided into several stages, each performing specific operations on the dataset to ensure a smooth and accurate reconstruction. First, a custom pose recorder collects images and camera poses, applying real-time pose compensation to correct any inconsistencies caused by sudden camera movements. Next, the images are preprocessed, including resizing them to fit the required dimensions and generating alpha masks that outline the object’s silhouette. The alpha masks are crucial for the subsequent reconstruction phase, where they help the machine learning model define the boundaries of the 3D object. Once the preprocessing is complete, the reconstruction phase begins. At this stage, the nvdiffrec tool from NVIDIA is used to reconstruct the 3D model. This tool applies a differentiable rendering technique to create a UV-mapped mesh, complete with texture maps that define the object’s material properties. The final 3D model can be downloaded and viewed interactively on various devices, including smartphones and web browsers.
Blending Low-End Devices with High-End Cloud Computing
A significant advantage of this pipeline is its ability to integrate low-end hardware, such as standard 2D cameras, with high-end cloud infrastructure for more resource-intensive tasks like model reconstruction. This makes the system both cost-effective and versatile, allowing it to be used in various industrial contexts. The research team emphasizes the potential applications of this technology in areas like Digital Twin systems, where accurate virtual models of physical objects are crucial for tasks such as process optimization and worker training. In industrial settings, Digital Twins can simulate real-world operations, allowing workers to train on virtual models before interacting with the actual machinery, thereby enhancing safety and reducing training time. The inclusion of augmented reality (AR) features also enables real-time feedback during the data acquisition process, further streamlining the workflow.
Addressing Challenges and Enhancing Model Quality
While the proposed pipeline has demonstrated its ability to efficiently reconstruct 3D models, the researchers have identified areas for potential improvement. They suggest adopting more advanced machine learning models to produce smoother edges and higher-quality reconstructions. Another area of focus is improving the alpha mask generation process, which is currently based on machine learning techniques that may introduce errors if not properly supervised. Future work could also involve breaking down the 3D models into smaller constituent parts to provide a more detailed and interactive user experience. Overall, this research presents a scalable and cost-effective solution for 3D model generation, with wide-ranging implications for industries such as augmented reality, manufacturing, and digital simulation.
Implications for Industry 4.0 and Future Potential
The combination of low-end data acquisition hardware with high-end cloud computing resources makes the system a valuable tool for industries looking to adopt Digital Twin technology and enhance their productivity in the context of Industry 4.0. The proposed pipeline not only achieves efficient 3D reconstruction but also lays the groundwork for future innovations in automated modeling, immersive training, and augmented reality-based workflows in industrial settings. By addressing the challenges of 3D model creation with scalable, cloud-native solutions, this research offers exciting prospects for industries moving towards more digitized and automated operations.
- FIRST PUBLISHED IN:
- Devdiscourse