AI-Driven Model Revolutionizes Road Safety in Senegal with 95% Accuracy
Researchers from Alioune Diop University and AGEROUTE in Senegal developed a deep learning model that detects road signs and deformations with 95.34% accuracy, enhancing road safety by providing real-time alerts to drivers. The model, using YOLOv8, integrates sign recognition and deformation detection, aiming to reduce accidents on Senegalese roads.
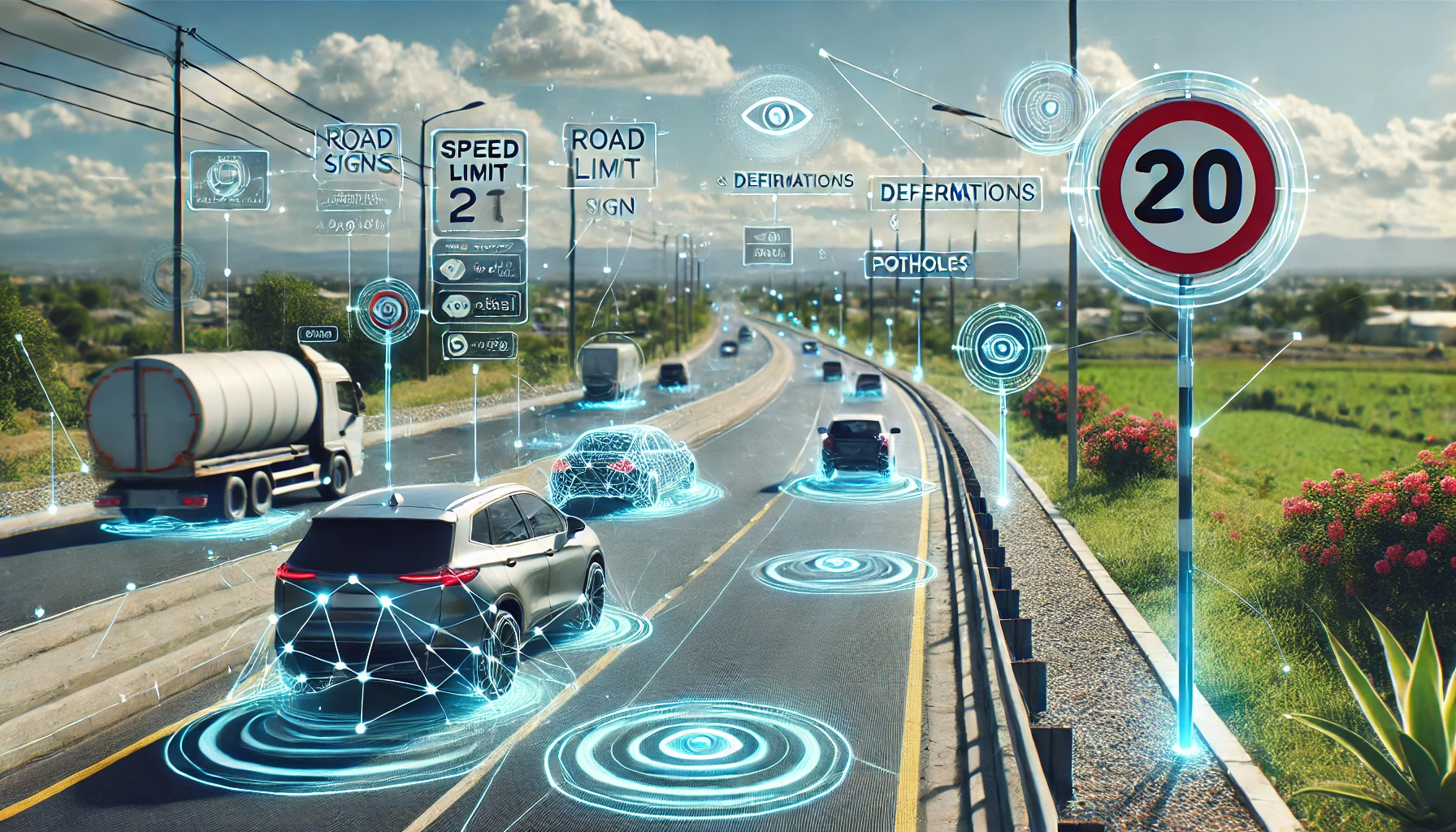
Researchers from Alioune Diop University and the Autonomous Agency for Works and Road Management (AGEROUTE) in Senegal have embarked on a significant initiative aimed at enhancing road safety through advanced technological solutions. In recent years, road safety has emerged as a critical issue in Senegal, particularly on national, secondary, and classified roads where accidents are alarmingly frequent. The primary causes of these accidents include non-compliance with traffic signs and deteriorating road conditions. These factors, compounded by the increasing number of vehicles on the roads, have led to a troubling rise in road accidents. The issue is not just limited to urban areas but extends across the entire country, affecting both major highways and remote routes. The challenge is exacerbated by the fact that road users often miss important roadside signs or fail to notice road deformations in unfamiliar areas, leading to dangerous situations for drivers and passengers alike. Despite efforts by Senegalese authorities to mitigate these risks through safety inspections and road network surveillance, the problem persists, largely due to the limited application of emerging technologies in road safety management.
Advancing Road Safety with Deep Learning
In response to this pressing issue, the researchers developed a deep learning model designed to detect and recognize road signs and deformations. The model is built on a hybrid dataset that combines publicly available images from Kaggle with data specifically collected by AGEROUTE in Senegal. This dataset was carefully curated to include a wide variety of road signs and deformations common in Senegal, providing a robust foundation for training the model. The training process utilized 80% of the dataset, with the remaining 20% reserved for testing. The results were promising, with the model achieving an accuracy rate of 95.34%. This high level of accuracy suggests that the model is well-suited for real-world applications, where it can be used to enhance road safety by alerting drivers to potential hazards in real time.
The Power of YOLOv8 for Real-Time Detection
The deep learning framework at the core of this model is YOLOv8, a state-of-the-art object detection system renowned for its speed and accuracy. YOLOv8 is particularly well-suited for this application because it can process images quickly, making it ideal for use in real-time detection systems. The system is capable of recognizing different types of road signs, including danger, obligation, prohibition, and other miscellaneous signs, as well as identifying various road deformations such as potholes and cracks. This dual capability makes the model particularly valuable for improving road safety in Senegal, where both sign recognition and road condition monitoring are essential for preventing accidents.
Building on Previous AI Models
The research also builds on a body of work in the field of artificial intelligence and road safety. Previous models, such as those developed by J D Zhao in 2017, focused on traffic sign recognition using video images. These earlier systems used support vector machines (SVM) for classification and achieved robust results in object detection and recognition. However, they were limited in their ability to accurately classify the specific nature of signs, such as distinguishing between different types of danger signs. The model developed by the Senegalese researchers addresses these limitations by integrating both sign detection and deformation recognition into a single system. This integration represents a significant advancement over earlier models, which typically handled these tasks separately and were less effective in real-world scenarios.
Practical Implications for Road Safety
The practical implications of this research are substantial. By providing drivers with real-time alerts about road signs and deformations, the model has the potential to significantly reduce the number of accidents caused by missed signs or unnoticed road hazards. This is particularly important in Senegal, where road safety remains a major public concern. The model’s ability to detect road deformations is especially valuable, as these hazards are often difficult for drivers to notice until it is too late to avoid them. By incorporating this capability, the researchers have created a comprehensive solution that addresses multiple facets of road safety.
Future Enhancements and Broader Impact
Looking forward, the researchers have identified several areas for further development. One of the main priorities is to expand the model's capabilities by integrating subclasses of traffic signs, which would allow the system to recognize a wider range of sign types and improve its accuracy in diverse driving conditions. Additionally, they plan to increase the variety of detectable road deformations by including more types of hazards, such as ruts and cracks. These enhancements would broaden the model’s applicability and make it even more effective in promoting road safety across Senegal and potentially other sub-Saharan countries. This research highlights the critical role that deep learning and artificial intelligence can play in addressing practical challenges in developing countries. By leveraging advanced technology, the researchers have created a powerful tool that not only enhances road safety but also sets a precedent for the broader adoption of AI-driven safety solutions in regions where such technologies have traditionally been underutilized. As the model continues to evolve and improve, it holds great promise for making roads safer and reducing the toll of traffic accidents in Senegal and beyond.
- READ MORE ON:
- road safety
- AGEROUTE
- YOLOv8
- support vector machines
- AI-driven safety
- FIRST PUBLISHED IN:
- Devdiscourse
ALSO READ
Building Road Safety and Sustainability: Corporate and Community Collaboration
West Bengal's Road Safety Overhaul: New Guidelines for Bus Operators
Gadkari's Road Safety Challenge: Aiming to Halve Accidents
Lane Indiscipline Takes Center Stage in Road Safety Debate
W Cape Graduates 133 Traffic Officers to Boost Road Safety for Festive Season