SIDQL: Transforming Motion Capture for Ultra-Low Latency and High Accuracy in the Metaverse
Researchers have developed SIDQL, a novel framework using Deep Q-Learning to enhance motion capture by efficiently extracting keyframes and reconstructing motion, significantly reducing data volume and transmission latency while maintaining high accuracy. This advancement is pivotal for the Metaverse, ensuring seamless and realistic avatar movements in immersive virtual environments.
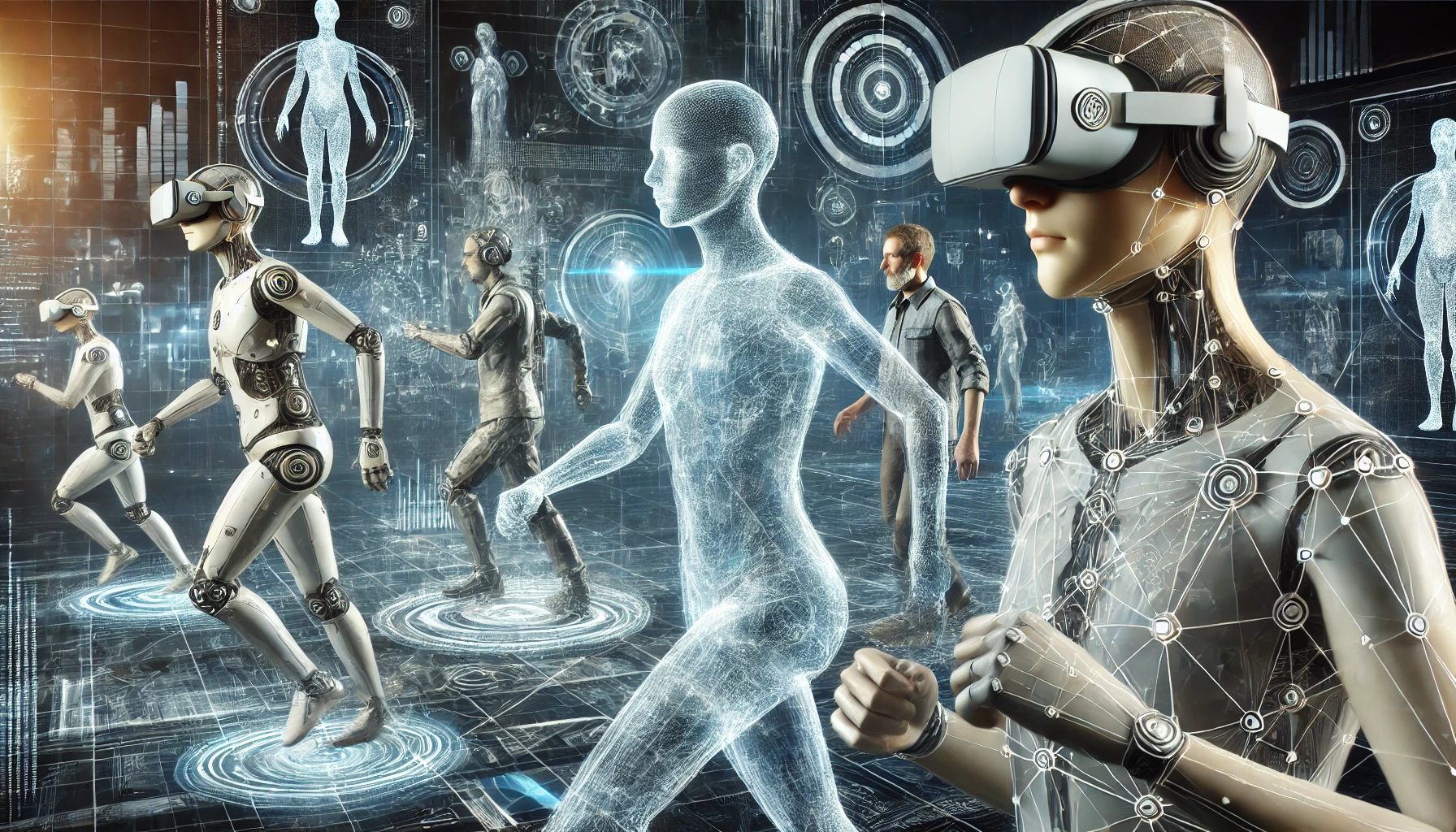
Researchers from the Hong Kong University of Science and Technology and the Hong Kong Polytechnic University have developed SIDQL, a novel framework that aims to revolutionize motion capture technology, addressing critical challenges faced by the Metaverse—a burgeoning virtual realm that seamlessly integrates with the physical world. This innovation enhances the synchronization of avatar movements with human actions, a necessity for the immersive environments facilitated by AI, VR, AR, and human-machine interaction technologies.
Solving Latency Issues in the Metaverse
The Metaverse demands ultra-low latency in communication systems to maintain a fluid user experience. However, current methods for motion capture struggle with the increasing data volume, resulting in delays and less fluid avatar movements. SIDQL introduces an efficient keyframe extraction and motion reconstruction framework to tackle this issue. Keyframe extraction, a technique borrowed from video processing, involves selecting representative frames from motion sequences to reduce data transmission requirements. Traditional methods often rely on predefined motion types and human-selected keyframes, which are not optimal for real-time applications in the Metaverse. SIDQL leverages Deep Q-Learning (DQL) to automate this process, significantly cutting down data volume while maintaining high accuracy.
Innovative Approach to Motion Capture Data
Motion capture data, unlike video, involves complex skeletal movements that require precise frame-by-frame analysis. SIDQL converts this data into a spherical coordinate system, maintaining constant bone lengths and ensuring natural motion during reconstruction. By utilizing polynomial interpolation for root points and spherical interpolation for other points, SIDQL ensures smooth transitions between keyframes, enhancing the realism of avatar movements. This innovative approach addresses the challenge of synchronizing avatar movements in the Metaverse, where large amounts of motion data need to be transmitted quickly to avoid perceptible delays.
Rigorous Testing and Impressive Results
The framework was rigorously tested using the CMU Graphics Lab Motion Capture Database, which includes a diverse range of human motions. SIDQL's performance was benchmarked against various existing methods, demonstrating a significant reduction in data transmission latency and an impressive reconstruction error rate of less than 0.09 when extracting just five keyframes. This is achieved by formalizing the keyframe extraction problem into an optimization problem aimed at minimizing reconstruction error. Using Deep Q-Learning (DQL), the Spherical Interpolation based Deep Q-Learning (SIDQL) framework generates appropriate keyframes for reconstructing motion sequences.
Bridging the Gap Between Digital and Physical Worlds
SIDQL's method involves first developing a new motion reconstruction algorithm in a spherical coordinate system, converting location and velocity data to keep bone lengths constant, and then reconstructing middle frames using spherical interpolation. The motion of the root point is reconstructed using polynomial interpolation methods. To minimize the mean reconstruction error, SIDQL formalizes the keyframe extraction problem into an optimization problem and uses a special reward function based on mean error for training. The framework can be trained with mixed-category motion sequences without needing labeled keyframes, which addresses the issue of current AI-based methods relying heavily on labeled data.
The SIDQL framework also considers the constancy of bone lengths and incorporates velocity information of human bones, which is often disregarded in current motion reconstruction methods. By utilizing this information, SIDQL improves the quality of reconstructed motion. Additionally, the framework includes a comprehensive training process involving the CMU database, which allows for the adjustment of hyperparameters and extensive evaluation against various baselines. This ensures that SIDQL not only reduces data volume and transmission latency but also maintains high reconstruction accuracy.
The development and testing of SIDQL highlight its potential in the Metaverse, setting the stage for future advancements in motion capture technology. As virtual environments continue to evolve, frameworks like SIDQL will be crucial in bridging the gap between the digital and physical worlds, offering users an immersive and seamless experience. This research underscores the importance of innovative approaches to motion capture and reconstruction, particularly in applications requiring real-time processing and low latency, such as the Metaverse.
In addition to its technical achievements, SIDQL represents a significant step forward in the integration of advanced AI techniques with practical applications in virtual reality. The use of Deep Q-Learning to optimize keyframe extraction and motion reconstruction showcases the potential of machine learning in solving complex problems in motion capture. This approach not only enhances the efficiency and accuracy of motion data transmission but also paves the way for more sophisticated and immersive virtual experiences.
Overall, SIDQL's development marks a crucial advancement in motion capture technology, addressing key challenges in the Metaverse and beyond. Its ability to reduce data volume, maintain high accuracy, and ensure natural motion transitions positions it as a leading solution for future virtual environments. As the demand for immersive digital experiences grows, the innovations introduced by SIDQL will likely play a pivotal role in shaping the future of motion capture and virtual reality.
- FIRST PUBLISHED IN:
- Devdiscourse