Human-Like Conversations with PerceptiveAgent: Enhancing AI Empathy and Interaction
PerceptiveAgent is an advanced multi-modal dialogue system that integrates speech perception with LLMs to generate empathetic and contextually appropriate responses by accurately interpreting acoustic and textual information.
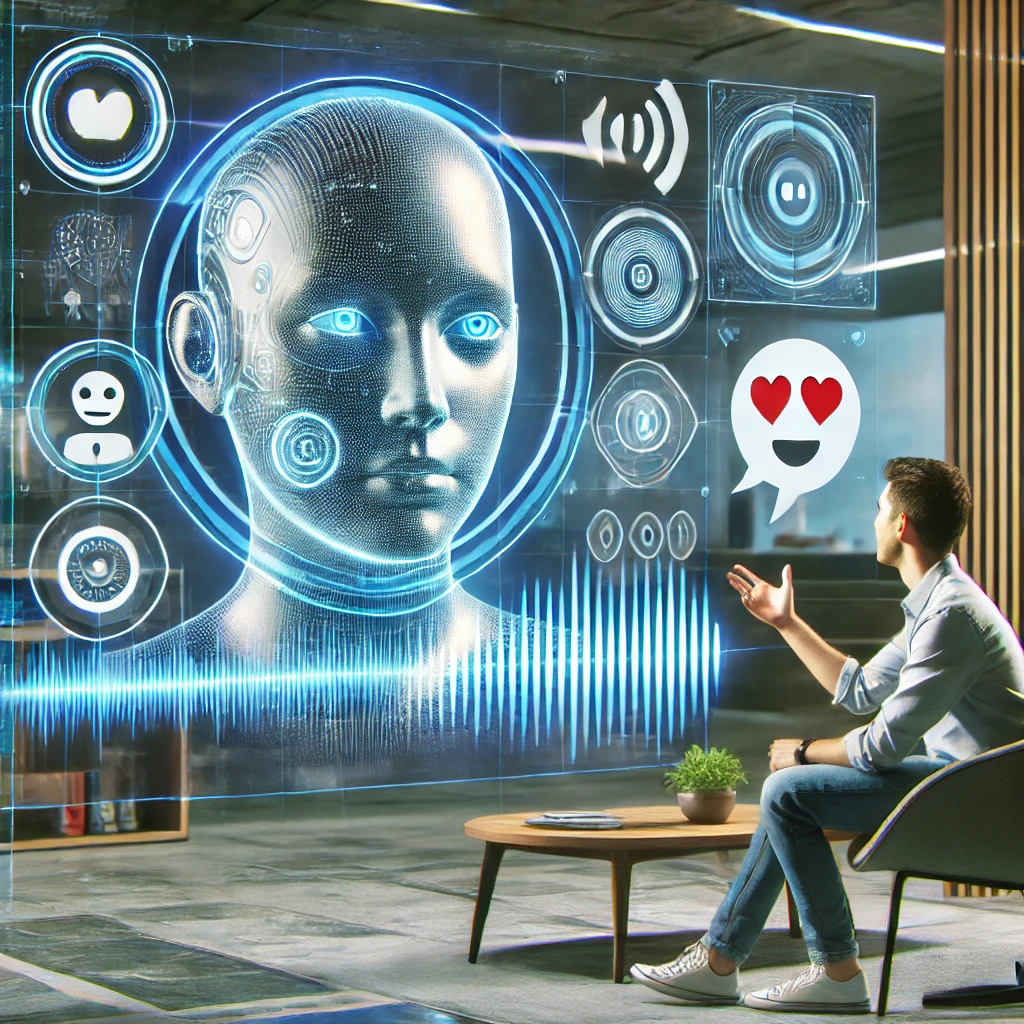
PerceptiveAgent, an empathetic multi-modal dialogue system, marks a significant advancement in Human-AI communication by addressing the limitations of current dialogue systems that fail to incorporate the acoustic information present in speech. This gap often results in misinterpretations and inconsistent responses in dialogues. Developed by researchers from the University of Science and Technology of China and Tencent Youtu Lab, PerceptiveAgent integrates speech modality perception with Large Language Models (LLMs) to discern deeper meanings and generate empathetic responses based on speaking styles. The system comprises three main components: the speech captioner, the LLM cognitive core, and the Multi-Speaker and Multi-Attribute Synthesizer (MSMA-Synthesizer).
Revolutionizing Dialogue with Acoustic Perception
The speech captioner plays a crucial role in capturing prosodic features from speech inputs and transcribing them as textual descriptions. Using a speech encoder from ImageBind and a pre-trained GPT-2 decoder, it aligns audio features with the latent space of a pre-trained language model. This alignment enables the system to accurately perceive acoustic information and generate captions that describe speaking styles in natural language. This perceptive capability allows the dialogue system to understand not just what is being said, but also how it is being said, adding a layer of empathy to the interactions.
Integrating Cognitive Intelligence with LLMs
The LLM module acts as the cognitive core of PerceptiveAgent, employing models like GPT-3.5-Turbo to comprehend multi-modal contextual history and deliver relevant responses. By integrating both the dialogue content and the captions generated by the speech captioner, the LLM can accurately understand the speaker's intentions and produce empathetic dialogue content. This integration of text and acoustic information enables the system to interpret nuances in speech that text-only systems might miss, such as the emotional tone or stress patterns, which are crucial for generating appropriate and empathetic responses.
Expressive Speech Synthesis for Realistic Interactions
The MSMA-Synthesizer is responsible for generating expressive speech based on the captions and response content from the LLM. It incorporates multiple speaking style attributes, including pitch, speed, energy, and emotion, to synthesize nuanced and realistic speech. This synthesizer improves upon previous models by providing fine control over speech expressiveness, ensuring that the generated audio matches the emotional and prosodic context of the dialogue. This multi-attribute control is vital for creating speech that feels natural and responsive to the user's emotional state.
Superior Empathy in AI-Driven Conversations
Experimental evaluations demonstrate that PerceptiveAgent excels in generating empathetic responses that align closely with the dialogue context. Compared to baseline systems that focus solely on linguistic information, PerceptiveAgent shows superior performance in both cognitive and affective empathy. The system's ability to perceive and integrate acoustic information enables it to produce more accurate and contextually appropriate responses. For instance, in scenarios where the speaker's linguistic content contradicts their true feelings, PerceptiveAgent successfully captures the underlying emotion and generates an appropriate response. Similarly, in scenarios where the speaker's excitement aligns with their words, PerceptiveAgent matches the enthusiasm in its response, showcasing its capability to handle both contradictory and consistent emotional cues.
The Road Ahead: Challenges and Opportunities
Despite its advancements, PerceptiveAgent has some limitations. The system's perception abilities are constrained by the comprehensiveness of its training dataset, particularly in recognizing speaker identity and background noise. Additionally, the system's response time may be affected by the accumulated delays from its cascaded components. The architecture of PerceptiveAgent, comprising the speech captioner, the LLM cognitive core, and the MSMA-Synthesizer, introduces latency which can affect real-time applications. Moreover, the maximum token length in LLMs may limit the handling of multi-turn dialogues, making it challenging to maintain context over extended conversations.
In the first case study, an unplanned meeting conversation between two friends, the system interprets the underlying emotion of speaker B, who is actually disinterested despite their words suggesting otherwise. PerceptiveAgent provides a response in accordance with this nuanced understanding, contrasting with a text-only system that might misinterpret the intent and generate a less appropriate response. In the second example, where a speaker is excited to share a paper from his mother, PerceptiveAgent mirrors this enthusiasm in its response, recognizing the speaker's excited mood and generating a contextually fitting reply.
PerceptiveAgent represents a significant step forward in developing empathetic multi-modal dialogue systems. By integrating perceptive speech captions with LLMs and expressive speech synthesis, it achieves a higher level of contextual understanding and response generation. This system holds promise for enhancing human-AI interactions across various applications, from virtual assistants to intelligent robots, by providing more natural and empathetic communication experiences. Its ability to capture and interpret the subtleties of human speech, including emotional tone and prosodic features, sets it apart from existing dialogue systems and paves the way for more advanced and emotionally intelligent AI communication tools.
- FIRST PUBLISHED IN:
- Devdiscourse