Breaking Barriers: How CryptoGPT Competes with GPT-4 in Financial Market Insights
CryptoGPT, a 7B language model, rivals GPT-4 in real-time financial news analysis through advanced annotation and fine-tuning techniques. This model provides SMEs with accessible and precise market insights, overcoming the limitations of traditional financial models.
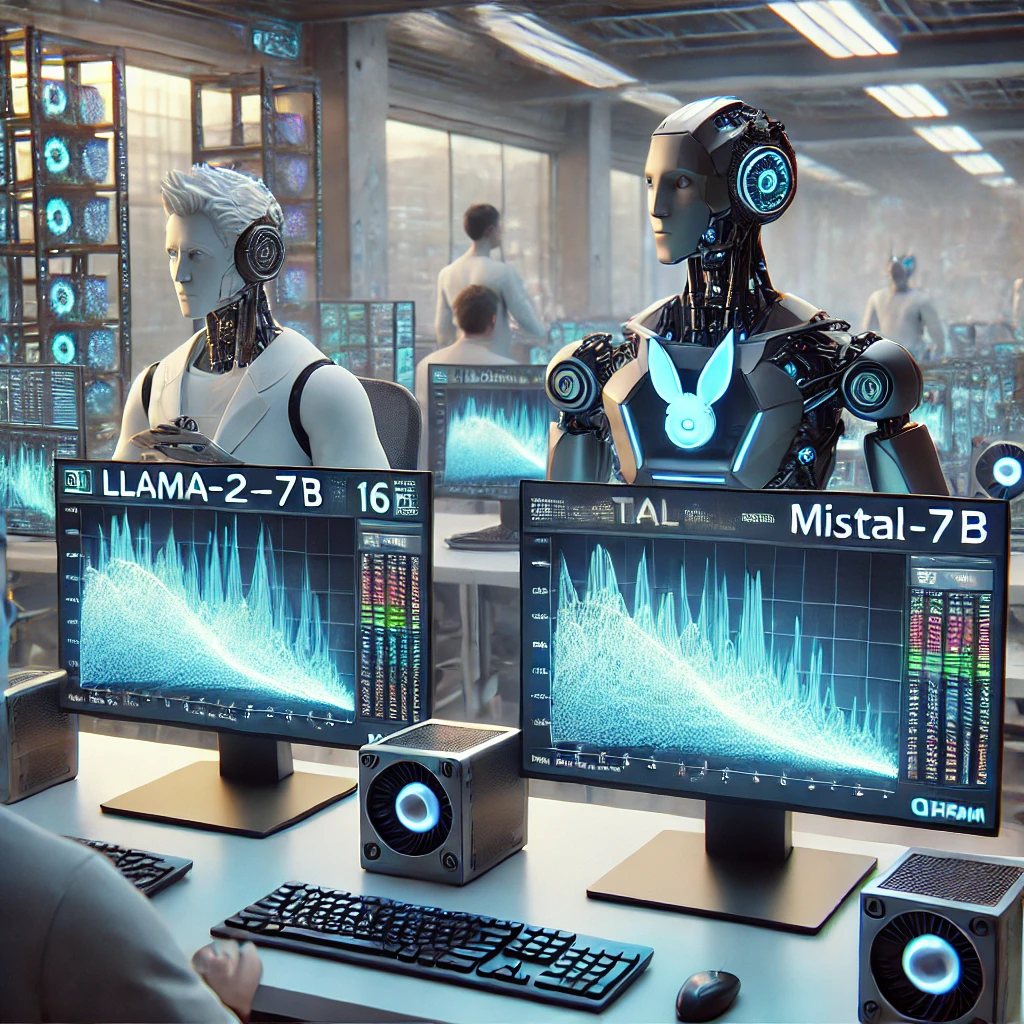
Ying Zhang from Beyond Horizon (Cannes, France), Matthieu Petit Guillaume from Omnios (Aix-les-Bains, France), and Aurelien Krauth from Leviatan (Aix-les-Bains, France) have conducted groundbreaking research on the cryptocurrency market, known for its extreme volatility and unique analytical challenges. Sudden fluctuations can be triggered by diverse events such as influential individuals' communications, political decisions, or rumors. These dynamics often escape traditional financial models, making it difficult to accurately interpret real-time market impacts. Major advancements in large language models (LLMs) have enabled real-time analysis of diverse events affecting the cryptocurrency market. However, these advancements come with increased complexity and size, posing significant training and deployment challenges, especially for small and medium-sized enterprises (SMEs). This complexity requires powerful server infrastructures and rigorous cost management, leading SMEs to depend on APIs provided by large companies. This dependence not only hampers SMEs' autonomous development but also exposes their and their clients' sensitive data to security and confidentiality risks on external servers.
Breaking Barriers with Bloomberg GPT
In March 2023, Bloomberg introduced Bloomberg GPT, a 50-billion parameter LLM designed for the financial sector. Despite its capabilities, its considerable size and resource requirements have limited its accessibility, particularly for resource-constrained environments. Initial open-source models of sizes between 7B and 30B have shown relatively poor performance in financial tasks. However, fine-tuning these open-source models can yield excellent results comparable to large commercial models like GPT-4, albeit at high costs. This study explores the use of a more accessible LLM to analyze real-time data from tweets, recent news, and cryptocurrency market trends, providing clients with real-time alerts on potential price rises and falls. Unlike classical sentiment analysis, text analysis in the financial domain is distinctly different due to the lack of open datasets for financial sentiment analysis. Creating such a dataset is the first challenge. This article presents an industrial research project conducted with limited resources, balancing annotation, training, and deployment costs with achieving high-performance results. Methodological aspects of the evaluation and the cost implications are also discussed.
Innovative Annotation Techniques
In developing an annotation methodology for in-depth cryptocurrency market analysis, the researchers partnered with expert financial analysts specializing in digital assets. This collaboration enabled the definition of 21 essential financial categories capturing various facets directly or indirectly influencing the cryptocurrency market. These categories include Regulation and Legislation, Adoption and Usage, Geopolitical Events, Technology and Infrastructure, Market Performances, Market Sentiment, Competition among Cryptocurrencies, Partnerships, Initial Coin Offerings (ICO), Media Coverage, Exchange Listings and Delistings, Trading Volume and Liquidity, Market Manipulation and Fraud, Influential Players' Interventions, Expert Analyses and Forecasts, Integration with Financial Services, Macroeconomic Indicators, Cryptocurrency Events and Conferences, Rumors and Speculations, and ESG Impact.
The annotation approach utilizes two main large language models specialized in identifying and categorizing financial texts, marking a significant advancement in annotation automation. A third model resolves annotation ambiguities provided by the first two models, enhancing accuracy while minimizing human intervention. A broad range of financial articles was collected via the Usearch platform over more than a year, totaling 42,600 articles (about 15,747,000 tokens). From these, 2,000 articles were semi-randomly selected for annotation, ensuring diverse market condition representation. Using the GPT-3.5 Turbo API for automatic annotation optimized costs and efficiency. Following recommendations from recent studies, three distinct prompts were developed to enrich the variety of automatic annotations. This process resulted in substantial token volumes, significantly reducing the time and resources typically dedicated to manual annotation through automatic verification.
Effective Fine-Tuning for Enhanced Performance
The fine-tuning process used pre-trained models like LLaMa-2-7B and Mistral-7B-v0.1 with the QLoRA method. To work within limited infrastructure, the training was adjusted for a maximum input length suitable for a single T4 GPU. The fine-tuning, done in half-precision, took significantly less time on a more powerful GeForce RTX 4090 GPU compared to a T4 GPU. The efficiency of this method was confirmed by the training loss curves for both models.
Due to the lack of existing manually annotated datasets, manual evaluation was necessary. Using intrinsic evaluation methods, 50 financial articles from various categories were selected for assessment. Seven models were tested, including fine-tuned and non-fine-tuned versions of LLaMa-2 and Mistral, as well as GPT-3.5 Turbo and GPT-4-0613. The results showed that GPT-4 scored the highest, while the fine-tuned Mistral-7B model performed almost as well. Non-fine-tuned models performed significantly worse.
Short-term efforts will focus on enhancing the evaluation process by increasing the dataset volume and involving a panel of economic and market finance experts for deeper assessment. Additionally, further fine-tuning of the current dataset using models like Zephyr-7b-alpha and LLaMa-3-8B, along with automatic annotation methods using GPT-4 for reevaluation, is planned. Long-term goals include developing a reinforcement learning reward model based on the evaluation dataset to refine the predictive and analytical capabilities of the models in complex market scenarios.
- FIRST PUBLISHED IN:
- Devdiscourse