Transforming Agriculture: AIoT-Driven Precision Water Management and Crop Optimization
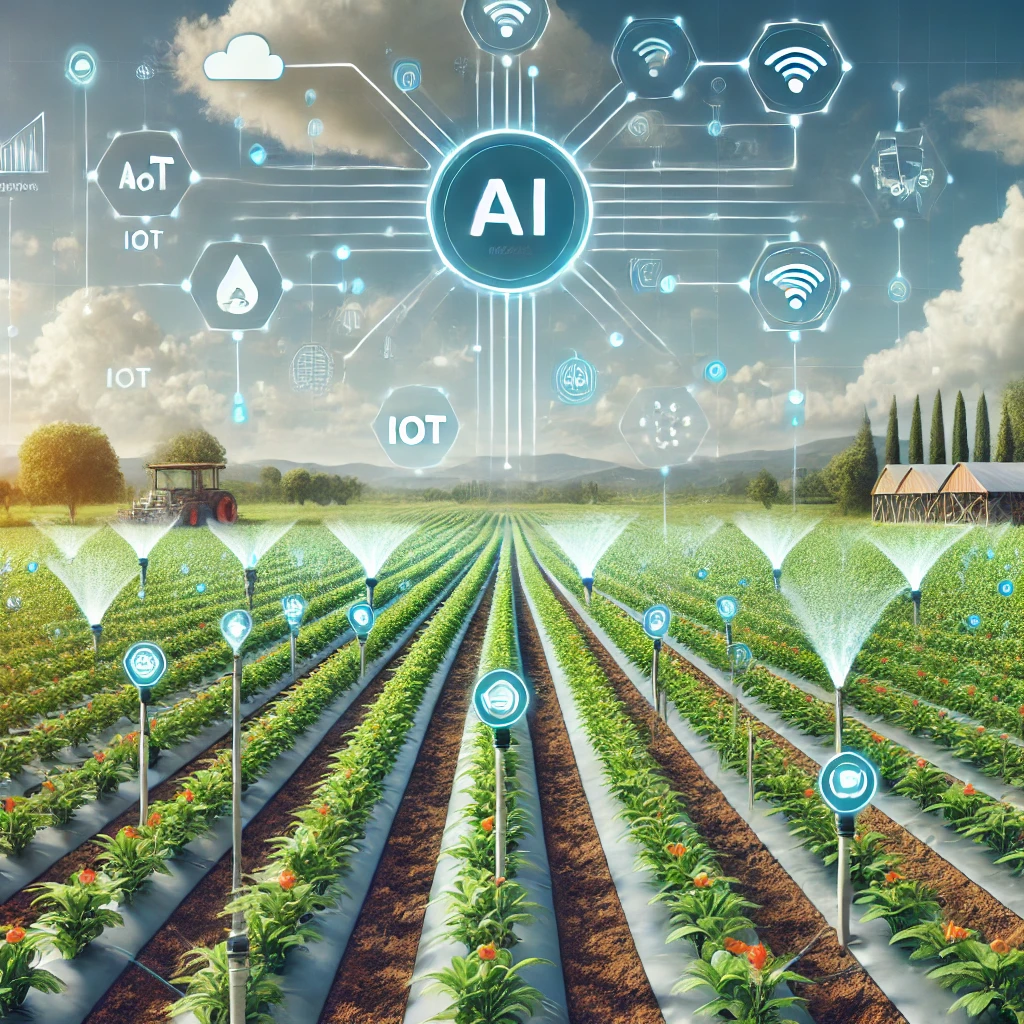
A study by Shashank Karna, Radhika Kotechaa, and Ritesh Kumar Pandey from K. J. Somaiya Institute of Technology, University of Mumbai, explores how Artificial Intelligence (AI) and the Internet of Things (IoT) can revolutionize agriculture by enhancing water management and crop yields. It focuses on addressing the limitations of conventional irrigation systems, which are labor-intensive and prone to inefficiencies. This approach aligns with the United Nations Sustainable Development Goals (SDGs), particularly the goals of Zero Hunger and Responsible Consumption and Production.
Revolutionizing Traditional Irrigation
Traditional irrigation systems often rely heavily on manual labor, which can lead to inaccuracies and inefficiencies in water usage. The paper argues that precise calculation of water requirements can significantly improve crop yields, reduce human involvement, and minimize decision-making errors. The integration of AI and IoT, referred to as AIoT, offers a solution by automating irrigation processes through real-time data analysis. This approach not only conserves water resources but also supports sustainable agriculture.
The AIoT System in Action
The proposed AIoT system operates in three phases. First, data is collected from various sensors deployed in the field, including those measuring soil moisture, temperature, and humidity. This real-time data acquisition ensures that the system has up-to-date information about the agricultural environment. Second, the collected data undergoes preprocessing to ensure its quality and consistency. This involves cleaning the data, handling missing values, and normalizing it to a standardized scale. The preprocessed data is then transmitted to an IoT cloud platform, which serves as a centralized repository for further analysis. In the final phase, the preprocessed data is analyzed by AI algorithms to predict the water requirements for crops. The AI considers factors such as soil moisture levels, weather conditions, crop type, and growth stage to determine the optimal amount of water needed for irrigation. The predictions are then used to control irrigation systems, ensuring that each crop receives the appropriate amount of water. This automated process minimizes water wastage and supports efficient water resource management.
Empirical Success and Broader Implications
The empirical evaluation of the AIoT system shows that it significantly improves crop yields while reducing human intervention. The system's ability to analyze diverse parameters and make accurate predictions helps address water conservation challenges and fosters sustainable agriculture practices. The paper highlights the potential of AIoT to revolutionize agriculture by providing real-time insights and automating critical processes. In reviewing the history and applications of AI in agriculture, the paper notes that AI has been used for tasks such as image recognition for plant disease detection, optimizing irrigation schedules, and controlling autonomous farm equipment. The transformative impact of AI-powered technologies on agriculture is evident, with AI enabling data-driven decision-making and improving efficiency and productivity. Similarly, IoT has emerged as a transformative technology in agriculture, providing real-time data and insights that help farmers make informed decisions. IoT optimizes resource management by efficiently harnessing data from sensors, enabling precise control over irrigation, fertilization, and crop health. The integration of AI and IoT, or AIoT, further amplifies the potential for data-driven decision-making and real-time monitoring in agriculture.
Future Prospects and Innovations
The implementation of the AIoT system involves several components, including sensors, IoT devices, and AI algorithms. The sensors collect data on various physical parameters, which is then processed and analyzed by AI algorithms to predict water requirements. The system continuously learns and adapts to changing environmental conditions, leading to more precise predictions over time. The evaluation of different AI algorithms shows that the Decision Tree Regressor performs the best in predicting water requirements. This model excels in both fitting the data accurately and minimizing prediction errors. The paper concludes that the AIoT system represents a significant step towards sustainable agriculture by optimizing crop yields, automating agricultural practices, and ensuring efficient water resource utilization. Looking ahead, the paper suggests that future research could explore the integration of other precision agriculture technologies, such as remote sensing and satellite imagery, to gain more detailed insights into crop conditions. Additionally, establishing platforms for data sharing and collaboration among farmers, researchers, and agricultural experts could further enhance the accuracy and efficiency of AI models in agriculture. Overall, the integration of AI and IoT in agriculture holds great promise for advancing sustainable farming practices. By leveraging cutting-edge technologies and tailoring solutions to specific crops, the AIoT system can help farmers optimize resource use, improve crop yields, and contribute to the achievement of global sustainability goals.
- FIRST PUBLISHED IN:
- Devdiscourse