AIOps Framework: Streamlining IT Operations with AI and Machine Learning
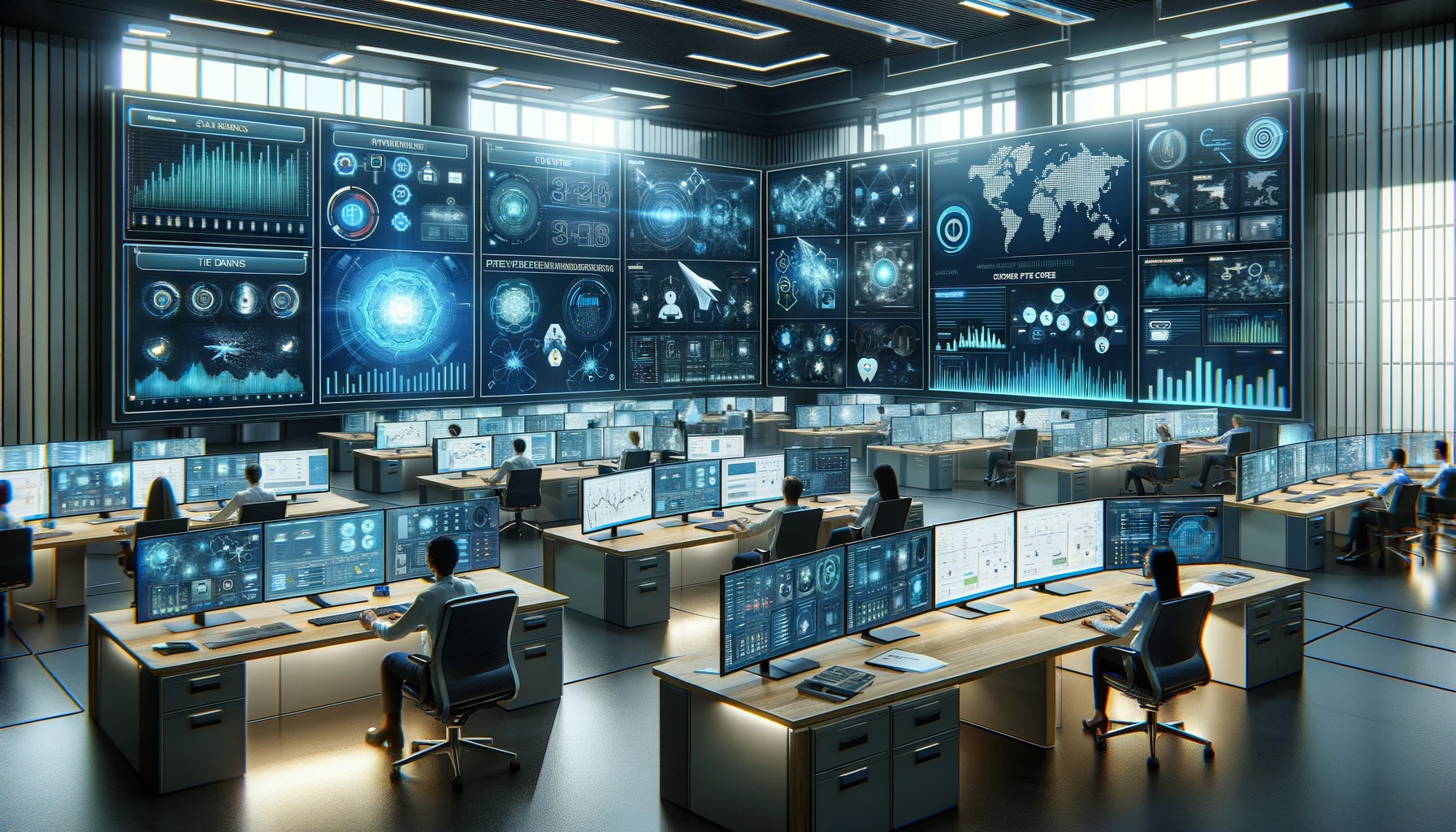
Managing modern IT systems is a complex and challenging task, especially with the massive amounts of data they generate. Researchers from the University of Lyon, INSA Lyon, and Infologic in France, including Youcef Remil, Anes Bendimerad, Romain Mathonat, and Mehdi Kaytoue, are addressing these challenges. Traditional methods that rely on manual tasks and predefined rules struggle to keep up with the demands. This is where AIOps, or Artificial Intelligence for IT Operations, comes into play. AIOps uses advanced analytics, machine learning, and big data to manage incidents more effectively.
Streamlining IT Management for Modern Challenges
Imagine your school's computer system crashing every day, causing chaos and frustration. Traditional IT methods would involve someone manually checking logs and trying to figure out what's wrong, which is time-consuming and often inefficient. AIOps, however, can analyze huge amounts of data in real time, predict potential issues before they happen, and even fix them automatically.
Despite its promise, AIOps is still a young field. Researchers and industries are working on it, but their efforts are scattered across different sectors without a consistent approach. This study aims to bring order to this chaos by proposing clear terminology and a structured way to manage incidents using AIOps.
Enhancing Operational Efficiency with AI
First, let's understand the basics of AIOps. IT environments today are more complex than ever. They include a mix of on-premises systems, cloud services, and mobile devices, all interacting in real time. Traditional IT management solutions can't keep up because they're not adaptive or scalable enough. AIOps, introduced by Gartner in 2017, combines big data and machine learning to make IT operations smarter and more efficient.
AIOps has six key abilities that enhance IT operations. First, perception involves gathering data from various sources such as logs, performance metrics, and network traffic. Second, prevention focuses on predicting potential failures and preventing high-severity outages. Third, detection is about identifying errors or anomalies in the system. Fourth, location involves analyzing data to find the root causes of problems. Fifth, action entails prioritizing incidents and implementing corrective measures. Lastly, interaction facilitates communication between the system and human operators, ensuring smooth coordination and resolution of issues.
Building a Comprehensive AIOps Framework
These abilities help AIOps detect and fix issues faster and more accurately than traditional methods. Companies are beginning to adopt AIOps, but there's still a long way to go to make it a standard practice.
One of the main challenges in AIOps is data management. IT systems generate vast amounts of data, and this data needs to be collected, stored, and analyzed efficiently. Different data sources have different formats, which makes data normalization and cleaning crucial. Another challenge is human interaction with AIOps. IT professionals are used to manual processes and might be skeptical about relying on AI. Building trust in AIOps solutions and making them user-friendly is essential.
The study proposes a comprehensive AIOps framework for incident management. This framework includes modules for data collection and ingestion, data storage and organization, data visualization, and the incident management procedure. The goal is to create a reliable, scalable, and secure system that can handle large volumes of data from diverse sources.
The Future of IT Management with AIOps
The incident management procedure involves several key phases. It starts with detection, which is the process of identifying incidents or potential issues. Once detected, the next step is prioritization, where incidents are ranked based on their urgency and impact. Following this, the assignment takes place, allocating the incidents to the appropriate people or teams for resolution. Finally, the resolution phase involves fixing the incidents and documenting the process to ensure that the issue is fully addressed and recorded for future reference.
Each phase has subcategories like incident classification, deduplication (removing duplicate incidents), root cause analysis, correlation (finding relationships between incidents), and mitigation (minimizing the impact of incidents).
To make AIOps effective, solutions need to meet certain criteria: they should be interpretable, scalable, maintainable, adaptable, robust, and evaluated in context. This means that the AI models should be transparent and easy to understand, able to handle large-scale data, require minimal maintenance, adapt to changing conditions, be resilient to variations in data, and be tested in real-world scenarios.
AIOps hold great potential for revolutionizing IT operations by making them more efficient and reliable. By establishing a clear framework and addressing the challenges in data management and human interaction, AIOps can become a powerful tool in managing modern IT systems. This study provides a roadmap for future developments, aiming to make AIOps a standard practice in the industry.
- FIRST PUBLISHED IN:
- Devdiscourse