Enhancing Ocean Monitoring: AI-Driven Solutions for Real-Time Buoy Trajectory Predictions
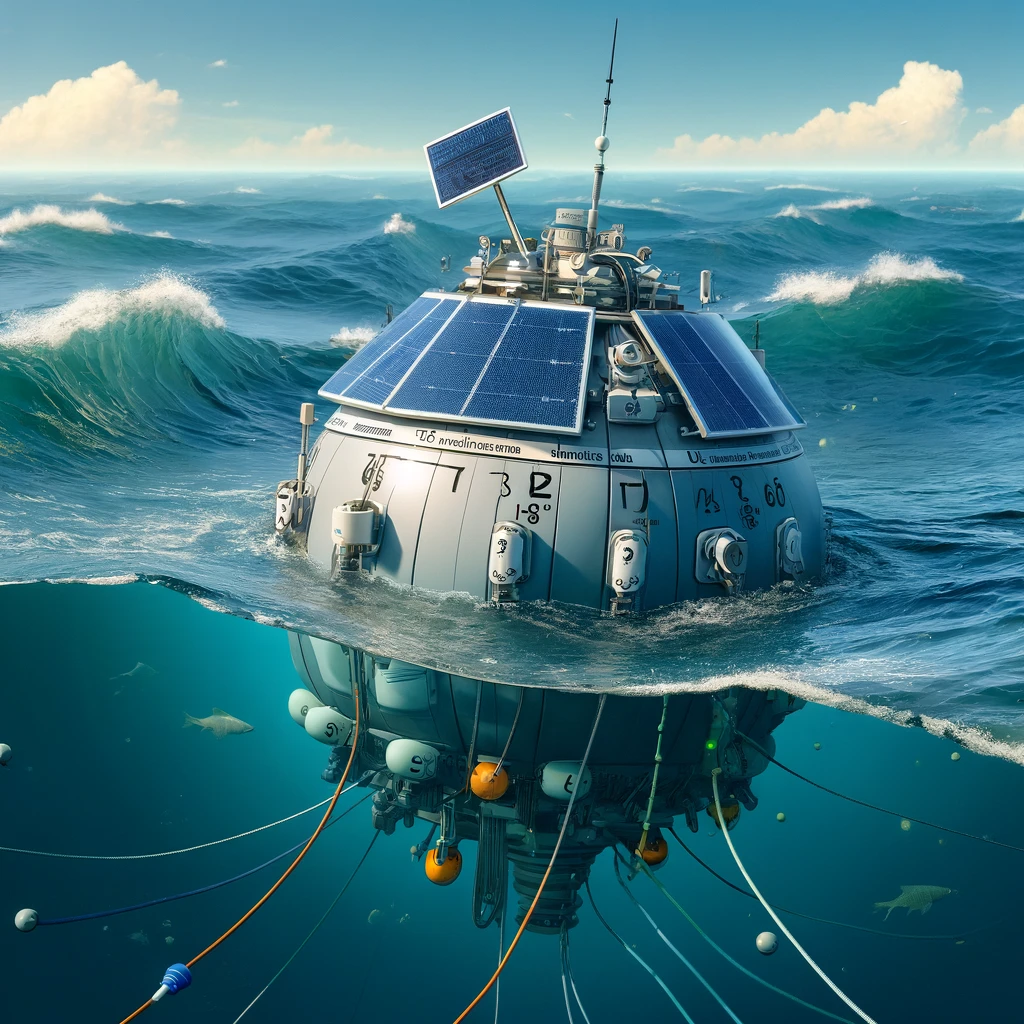
A study by Miaomiao Song, Wei Hu, Shixuan Liu, Shizhe Chen, Xiao Fu, Jiming Zhang, Wenqing Li, and Yuzhe Xu from the Institute of Oceanographic Instrumentation, Qilu University of Technology, China presents a solution for predicting the trajectories of Surface Drifting Buoys (SDBs) using an AI-driven approach. This study involves the creation of a scalable mathematical model for trajectory prediction, which simplifies the challenge by focusing on predicting velocities in the eastward and northward directions. A four-layer trajectory prediction calculation framework (FLTPCF) is developed, which outlines a workflow for real-time online training of marine environment data and SDB trajectories.
Surface Drifting Buoys (SDBs) are specialized floating devices used to collect and transmit oceanographic data. Equipped with sensors, they measure sea surface temperature, salinity, currents, wave height, barometric pressure, and wind speed and direction. These buoys drift with ocean currents and surface winds, providing valuable real-time data for marine environmental monitoring, weather forecasting, climate research, marine navigation, and fisheries management.
Hybrid Neural Network Model: CNN–BiGRU–Attention
The core of the proposed method is a hybrid neural network model named CNN–BiGRU–Attention. This model integrates a Convolutional Neural Network (CNN) for local and global feature extraction, a Bidirectional Gated Recurrent Unit (BiGRU) for handling long-term dependencies, and an Attention mechanism (AM) for focusing on crucial features. The model's effectiveness is demonstrated through extensive experiments, including ablation studies, comparative analyses with state-of-the-art models such as BiLSTM and Transformer, evaluations against numerical methods, and adaptability tests under varying sea conditions. The results show that the CNN–BiGRU–Attention model outperforms other models in terms of convergence, accuracy, and generalization capabilities in predicting 24, 48, and 72-hour trajectories of SDBs.
The study begins with an introduction to the importance and applications of SDBs in global marine monitoring and the challenges in predicting their trajectories due to complex marine environmental factors. It then reviews the advancements in AI technologies, particularly artificial neural networks (ANNs), for addressing time-series prediction problems in various fields, including marine environmental monitoring.
Mathematical Model and Computational Framework
The researchers detail the mathematical model for SDB trajectory prediction, transforming the trajectory point prediction problem into a velocity prediction problem. They discuss the mechanical structure of SDBs and the critical marine environmental factors affecting their motion. The mathematical model is defined through a series of equations that describe the relationship between the trajectory points, marine environmental data, and SDB velocities.
A computational framework comprising four layers of data acquisition, data preprocessing, intelligent prediction computation, and data postprocessing is established to implement the AI-driven trajectory prediction model. The data acquisition layer involves the collection of real-time marine environmental data and SDB trajectory data from various sources. The data preprocessing layer includes tasks such as handling missing data, spatial interpolation, spatiotemporal alignment, and normalization. The intelligent prediction computation layer integrates CNN, BiGRU, and AM to predict the SDB drifting velocities. The data postprocessing layer converts the predicted velocities back into trajectory points for visualization and further applications.
The hybrid neural network model, CNN–BiGRU–Attention, is elaborated, explaining the role of each layer in capturing spatiotemporal features and enhancing prediction accuracy. The model is trained using a dataset of marine environmental and SDB trajectory data, and its performance is evaluated using metrics such as root mean square error (RMSE), mean absolute error (MAE), and coefficient of determination.
AI-Driven Model Redefines Buoy Path Accuracy
Experimental results validate the superiority of the CNN–BiGRU–Attention model over other models in predicting SDB trajectories. The ablation study highlights the importance of integrating CNN, BiGRU, and AM, showing that each component contributes to the model's performance. The visualization of predicted trajectories further demonstrates the model's accuracy and consistency in different sea conditions.
The paper presents a significant advancement in predicting SDB trajectories using an AI-based approach, with potential applications in marine environmental monitoring, SDB deployment planning, and rescue operations. The integration of CNN, BiGRU, and AM in the trajectory prediction model proves to be effective in handling the complexities of marine environmental data and improving prediction accuracy.
- FIRST PUBLISHED IN:
- Devdiscourse