A Closer Look at Artificial Intelligence: The Testing Process
In this detailed exploration, we delve into the intricate world of Artificial Intelligence (AI) testing. Join us as we unravel the various methods and challenges involved in assessing AI's human-like capabilities and understanding its role in shaping the future of technology.
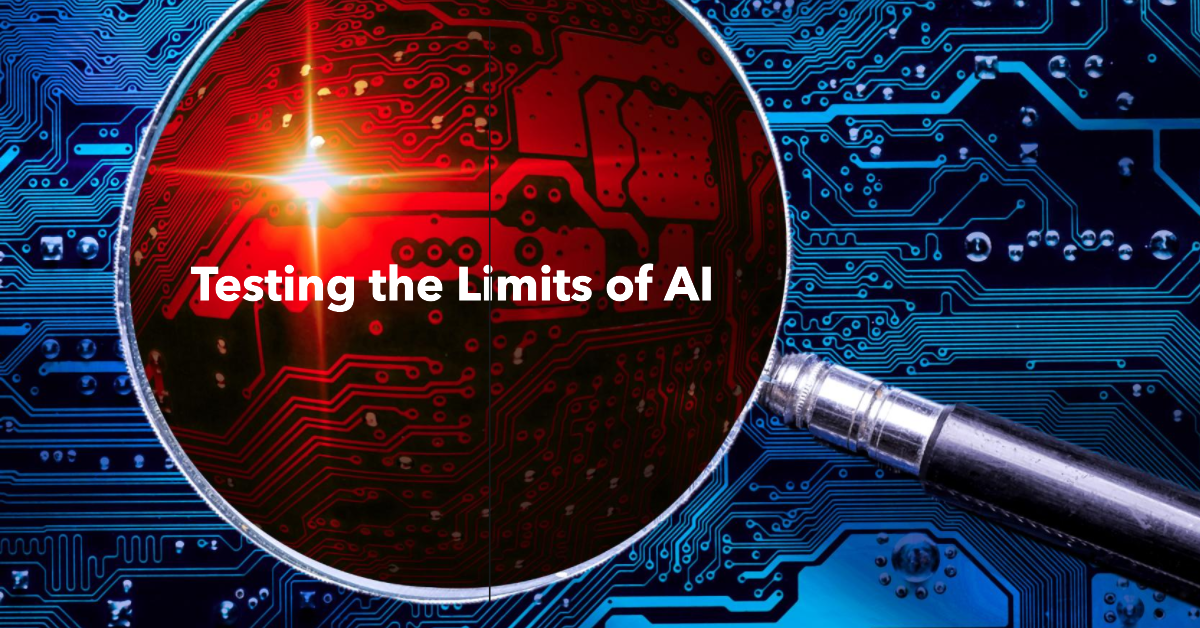
Artificial Intelligence (AI) has transcended the realm of science fiction and firmly entrenched itself in our daily lives. From virtual assistants like Siri and Alexa to self-driving cars and recommendation algorithms, AI technologies are ubiquitous. However, as AI evolves, so does the need to scrutinize and evaluate its abilities. In this blog post, we will take a deep dive into the testing process of Artificial Intelligence, shedding light on the methods, challenges, and implications of assessing AI's human-like intelligence.
Understanding the Need for AI Testing
Before we delve into the nitty-gritty of AI testing, it's crucial to understand why it's necessary. The primary goal of AI testing is to determine whether a machine can exhibit human-like intelligence in specific tasks or scenarios. This evaluation helps ensure that AI systems meet the desired standards of performance, reliability, and safety.
The rapid advancements in AI and machine learning technologies have raised several critical questions, including:
- Reliability: Can AI systems consistently perform tasks accurately, without unexpected errors or biases?
- Ethical Considerations: How do AI systems make ethical decisions in situations involving human lives or sensitive information?
- Bias and Fairness: Are AI algorithms fair and free from discrimination?
- Scalability: Can AI systems adapt and scale efficiently in various environments?
- Safety: How do we ensure that AI technologies do not pose risks to humans or the environment?
Methods of AI Testing
The methods used to test AI systems can be diverse, depending on the goals and complexity of the system in question. Here are some of the primary methods employed in AI testing:
-
Functional Testing: This involves testing the functionality of specific AI algorithms or components. Engineers assess how well the AI performs its intended tasks, such as natural language processing, image recognition, or data analysis. Functional testing helps identify bugs and errors that can hinder performance.
-
Performance Testing: Performance testing evaluates how an AI system handles varying workloads and conditions. It assesses factors like response time, throughput, and resource utilization. This type of testing helps ensure that the AI system can handle real-world demands effectively.
-
Security Testing: With the increasing use of AI in security-sensitive applications, security testing is vital. It involves evaluating the AI system's vulnerability to cyberattacks, data breaches, and malicious inputs.
-
Usability Testing: Usability testing focuses on the user experience (UX) of AI-driven interfaces or applications. Testers assess how easily users can interact with the AI system, providing valuable insights for improving the user interface.
-
Ethical Testing: This is an emerging field in AI testing, aiming to assess whether AI systems make ethical decisions. Test scenarios may involve sensitive topics, and the goal is to ensure AI systems adhere to ethical guidelines.
The Turing Test and Beyond
The Turing Test, proposed by Alan Turing in 1950, is one of the most famous benchmarks for assessing AI's human-like intelligence. In the Turing Test, a human judge engages in natural language conversations with both a human and a machine, without knowing which is which. If the judge can't tell for sure whether they're talking to a human or a machine because of how they respond, then we say the machine has successfully passed the Turing Test.
While the Turing Test is a landmark in AI testing, it has its limitations. Critics argue that passing the test does not necessarily indicate true human-like intelligence but rather a simulation of it. AI developers today strive for more comprehensive methods to evaluate AI's capabilities, considering factors like reasoning, ethics, and decision-making.
Challenges in AI Testing
AI testing is not without its challenges. Some of the notable hurdles include:
-
Data Quality: AI systems heavily rely on data, and the quality of data used for training can significantly impact their performance. Ensuring clean, unbiased data is a persistent challenge.
-
Interpretable AI: Many AI algorithms, especially in deep learning, are often referred to as "black boxes" because they lack transparency in their decision-making processes. Understanding and interpreting AI decisions remain a challenge.
-
Testing for Unforeseen Scenarios: AI systems are exposed to a wide range of real-world scenarios, some of which may not have been anticipated during development. Testing for these unforeseen scenarios is complex but essential.
-
Bias and Fairness: Detecting and mitigating bias in AI algorithms is an ongoing concern, as biased algorithms can perpetuate discrimination and inequality.
The Humanization of AI
As we continue to test and evaluate AI, the ultimate goal is to humanize it to some extent. AI is not just about performing tasks; it's about understanding context, making ethical decisions, and adapting to dynamic situations. Achieving human-like intelligence in AI has profound implications for various fields, including healthcare, finance, transportation, and education.
Humanizing AI means enabling it to:
-
Understand Context: AI should be capable of understanding the nuances of language, culture, and context in conversations and interactions.
-
Learn Continuously: AI systems should be able to learn from new information and adapt to changing circumstances without human intervention.
-
Make Ethical Decisions: Ensuring that AI systems make ethical choices in complex situations is vital for responsible AI deployment.
-
Interact Emotionally: Enabling AI to recognize and respond to human emotions can enhance its usability in various applications, such as mental health support or customer service.
Conclusion
Testing Artificial Intelligence is an ongoing and dynamic process that continues to evolve with the technology itself. As AI systems become more integrated into our lives, the need for comprehensive testing methods becomes even more critical. From functional testing to ethical assessments, AI testing helps us unlock the potential of AI while addressing its limitations and challenges.
In our quest to humanize AI, we must continue to refine our testing methodologies, push the boundaries of what AI can achieve, and ensure that it aligns with our values and ethical standards. As technology advancements continue, AI testing remains at the forefront of ensuring that these intelligent systems enhance our lives while maintaining the highest standards of performance and safety.