AI outperforms human experts in predicting study outcomes
Rather than replacing human experts, the researchers envision LLMs as collaborators in the scientific process. By leveraging their predictive capabilities, scientists can focus on designing innovative experiments and interpreting results within broader theoretical frameworks. This synergy between human creativity and machine precision could lead to unprecedented breakthroughs across disciplines.
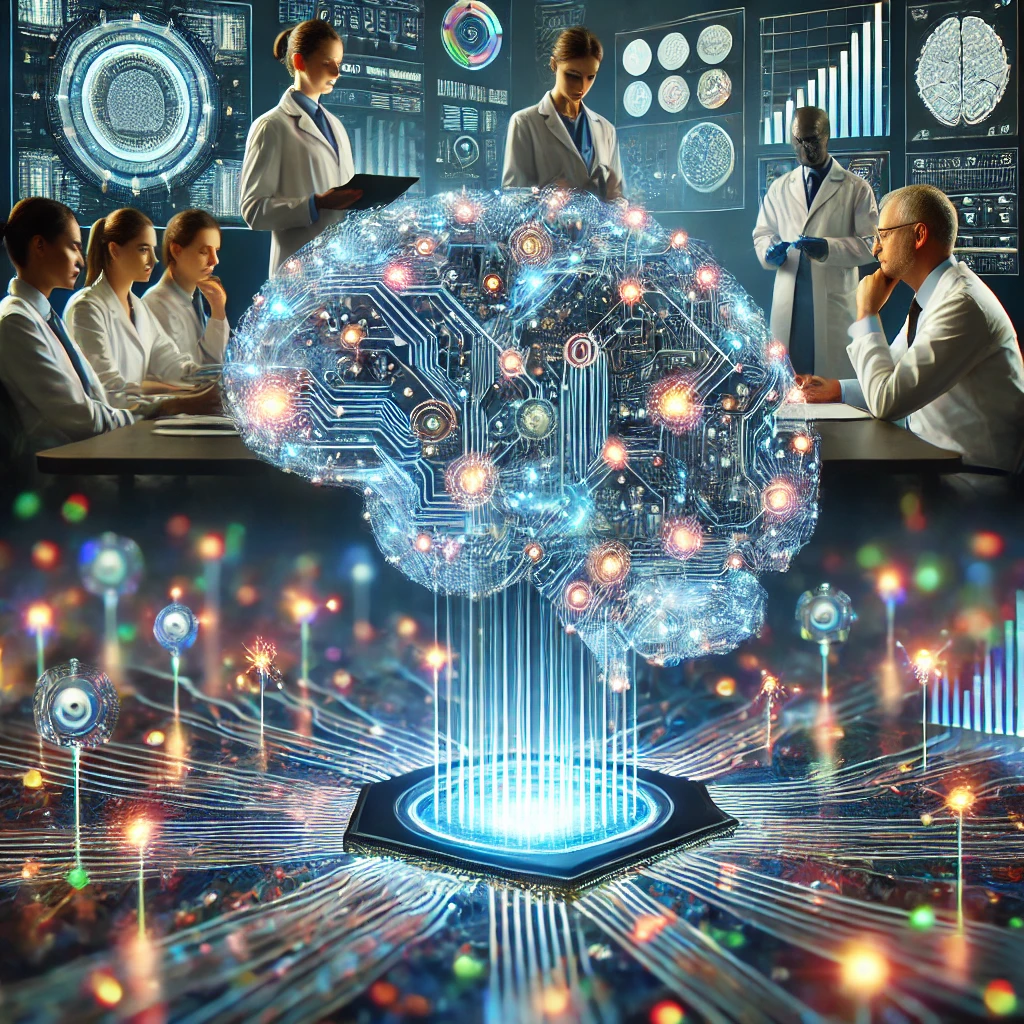
LLMs as game-changers
To test this hypothesis, the researchers developed BrainBench, a specialized benchmark for assessing the ability of LLMs to predict neuroscience results. Unlike traditional benchmarks that focus on retrieving or reasoning over established knowledge, BrainBench takes a forward-looking approach. It evaluates whether a model can discern which of two modified abstracts - the original or an altered version with different results—aligns with the actual study findings.
The challenge for LLMs is not merely one of regurgitating memorized information. Instead, BrainBench tests their ability to synthesize complex data patterns and infer outcomes from incomplete but interrelated signals. This ability to generalize and predict makes LLMs uniquely suited for tackling the complexities of neuroscience.
The results were striking. General-purpose LLMs achieved an average accuracy of 81.4% on BrainBench, significantly outperforming human experts, who managed a 63.4% accuracy rate. These human participants - comprising doctoral students, postdoctoral researchers, and academic faculty - represented diverse levels of expertise and experience in neuroscience. Even when restricted to participants with high self-reported expertise, humans fell short of matching the predictive prowess of LLMs.
To further enhance performance, the researchers fine-tuned an existing LLM using neuroscience-specific training data, creating BrainGPT. This model was trained on over 1.3 billion tokens sourced from neuroscience literature spanning two decades. With the use of Low-Rank Adaptation (LoRA) techniques, BrainGPT exhibited even greater accuracy, improving predictions by an additional 3% compared to its pre-trained state.
What makes LLMs like BrainGPT stand out is their ability to integrate contextual information from across an abstract. They do not merely focus on localized data points but draw insights from methods, study designs, and broader experimental contexts. This holistic approach enables them to uncover patterns and relationships that often elude human comprehension.
Future implications
The implications of this study extend far beyond neuroscience. The success of LLMs in predicting experimental outcomes suggests a future where artificial intelligence becomes an indispensable tool in scientific research.
Some potential applications and benefits include:
- Enhanced experimental design: By predicting likely outcomes, LLMs can help scientists prioritize experiments with the highest potential impact, saving time and resources.
- Bridging knowledge gaps: LLMs can identify overlooked patterns in existing literature, opening new avenues for research.
- Accelerated discovery: With the ability to process vast datasets in real time, LLMs can significantly reduce the time required to synthesize findings and propose new hypotheses.
- Collaborative research: As complementary tools, LLMs can work alongside human experts, providing data-driven insights while leaving the nuanced interpretation to human judgment.
Ethical and practical Considerations
Despite their advantages, the integration of LLMs into scientific workflows is not without challenges. Ethical concerns, such as data privacy, algorithmic bias, and the potential misuse of AI-generated predictions, must be carefully addressed. The researchers emphasize the importance of maintaining transparency in how these models are trained and evaluated.
Another key consideration is ensuring that LLMs remain updated with the latest scientific literature. The study highlights the potential of techniques like LoRA to fine-tune models efficiently, ensuring they stay relevant in rapidly evolving fields like neuroscience.
Human-AI Synergy
Rather than replacing human experts, the researchers envision LLMs as collaborators in the scientific process. By leveraging their predictive capabilities, scientists can focus on designing innovative experiments and interpreting results within broader theoretical frameworks. This synergy between human creativity and machine precision could lead to unprecedented breakthroughs across disciplines.
However, the study also cautions against over-reliance on AI. For instance, LLM predictions that contradict established theories might deter researchers from pursuing unconventional but potentially groundbreaking experiments. Balancing AI-driven insights with human intuition will be crucial in maximizing the benefits of this technology.
The researchers hope that this study serves as a blueprint for integrating AI into other knowledge-intensive domains, paving the way for a more efficient, equitable, and innovative scientific ecosystem.
- FIRST PUBLISHED IN:
- Devdiscourse