Urban vs Rural: Real-Time Indicators in Forecasting Household Welfare During the Pandemic
A World Bank study found that real-time indicators like Google search and mobility data effectively predicted early-stage economic disruptions during COVID-19, especially in urban areas. However, their predictive power declined over time, with limited accuracy in rural regions and for certain welfare indicators like food insecurity and school dropouts.
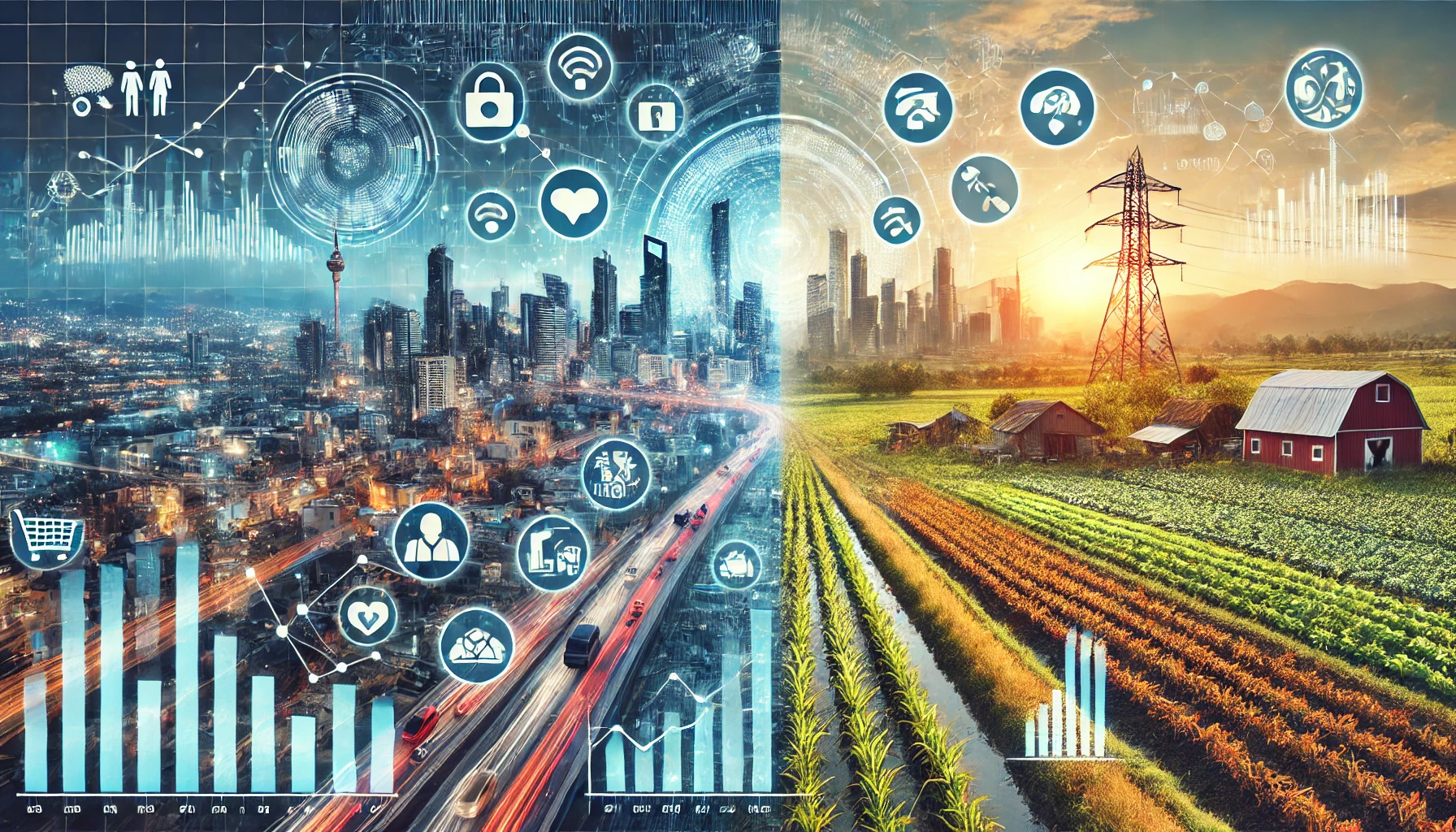
A World Bank study, conducted by researchers from the Development Economics, Poverty and Equity Global Practice, and the Human Capital Project, explores the extent to which real-time indicators, such as internet search trends, mobility data, and satellite imagery, tracked changes in household welfare during the COVID-19 crisis in developing countries. The research involved analyzing data from high-frequency phone surveys conducted in around 300 regions across 20 countries between April 2020 and February 2021. These phone surveys provided essential information on household income changes, employment, food security, and educational disruptions, offering a basis to evaluate how real-time data could serve as fast, cost-effective proxies for socioeconomic conditions during the pandemic.
Google Search Data Predicts Early-Stage Economic Shocks
The study found that real-time indicators, particularly Google search data, were effective in predicting early-stage economic disruptions, such as income declines and work stoppages. In the first wave of data collection, conducted between April and August 2020, search terms related to “money,” “jobs,” “free food,” and “religion” proved to be powerful predictors. These search trends explained up to 37% of the variation in the share of households reporting total income declines and 34% of the variation in work stoppages across regions. Google mobility data, which tracked movement patterns in response to lockdown measures, also demonstrated strong predictive capacity. For instance, regions with lower workplace mobility showed higher levels of wage income declines, reflecting the widespread impact of lockdowns on employment opportunities. The combination of Google search and mobility data provided a meaningful early signal of economic distress.
Real-Time Indicators Lose Predictive Power Over Time
Despite this promising start, the study highlighted the declining predictive power of these indicators over time. While real-time data continued to capture some variations in income loss and employment during later waves of the survey, they were less effective at predicting household welfare changes as the pandemic evolved. By February 2021, Google mobility measures explained only 15% of the changes in current employment levels. Moreover, indicators like Google search trends related to food and religion, which had been strong predictors early on, lost some of their explanatory power as the crisis dragged on. The ability of real-time data to predict other welfare indicators, such as food insecurity and school dropouts, was also limited. For example, searches related to food, which were expected to indicate rising food insecurity, did not strongly correlate with actual survey responses on households skipping meals or going a whole day without food. Similarly, educational disruptions, particularly children leaving school, proved difficult to predict using the real-time data available.
Urban Areas Show Stronger Predictive Accuracy
One of the key findings of the study was the contrast in predictive accuracy between urban and rural regions. Real-time indicators, especially Google search and mobility data, were significantly more effective in urban areas than in rural ones. In urban regions, these indicators explained up to 55% of the variation in income declines and 33% of the variation in employment, highlighting their potential as a tool for urban policymakers responding to sudden economic shocks. In contrast, in rural areas, where internet access and smartphone penetration are lower, the predictive power of these indicators was much weaker. For example, real-time data were poor at predicting non-farm and wage income declines in rural regions, partly because fewer people in these areas use Google services or mobile internet frequently. This disparity suggests that real-time data are best suited for monitoring economic conditions in urban settings and more developed regions, while alternative methods may be needed to assess welfare changes in rural communities.
Satellite Data Proves Less Reliable for Economic Tracking
Satellite data, including nighttime light emissions, vegetation indices, and air pollution levels, were also evaluated as potential predictors of welfare changes, but they generally performed worse than Google’s search and mobility data. Nighttime lights, often used as a proxy for economic activity, were not selected in any of the models as significant predictors of household income or employment changes. Vegetation indices, while relevant in some rural contexts, had limited predictive power overall, except for food insecurity in certain areas where agricultural disruptions were severe. Air pollution data, specifically nitrogen dioxide levels, showed some correlation with work stoppages and non-farm income changes, particularly in urban regions, where economic activity tends to drive pollution levels.
The Future of Real-Time Indicators in Crisis Management
The study concludes that while real-time indicators, particularly from Google, provide useful insights during the early phases of an economic crisis like COVID-19, they are not a complete substitute for traditional survey-based methods of tracking household welfare. These indicators worked best in urban areas during the initial shock of the pandemic but became less reliable over time and in rural contexts. The findings underscore the need for a balanced approach, where real-time data can complement traditional data collection efforts, providing rapid assessments in moments of acute crisis. However, the limitations of real-time data particularly in rural areas with low internet access mean that policymakers must be cautious when using these indicators to design interventions. The study recommends further research into improving predictive models by incorporating more diverse real-time data sources and applying advanced machine learning techniques to better capture the nuances of household welfare changes in developing countries.
- READ MORE ON:
- World Bank
- COVID-19
- Real-time indicators
- FIRST PUBLISHED IN:
- Devdiscourse
ALSO READ
World Bank Urges Bhutan to Diversify Economy and Boost Job Creation for Sustainable Growth
World Bank Chief Calls for Swift Global Reforms
World Bank President Calls for Faster, Simplified Processes Amid Global Challenges
World Bank Approves $150M Loan to Upgrade Bahia’s Road Infrastructure, Benefiting 2.35 Million People
World Bank Report: Healthy Longevity Could Save 150 Million Lives by 2050, Transform Developing Countries