Direct Tax Engagement: How Indonesia Increased Compliance with Face-to-Face Strategies
The World Bank's study on door-to-door tax enforcement in Gorontalo, Indonesia, showed significant improvements in tax compliance, particularly among lower-value properties. Using machine learning, the program demonstrated the potential of direct engagement strategies but raised concerns about the regressive effects of data-driven enforcement.
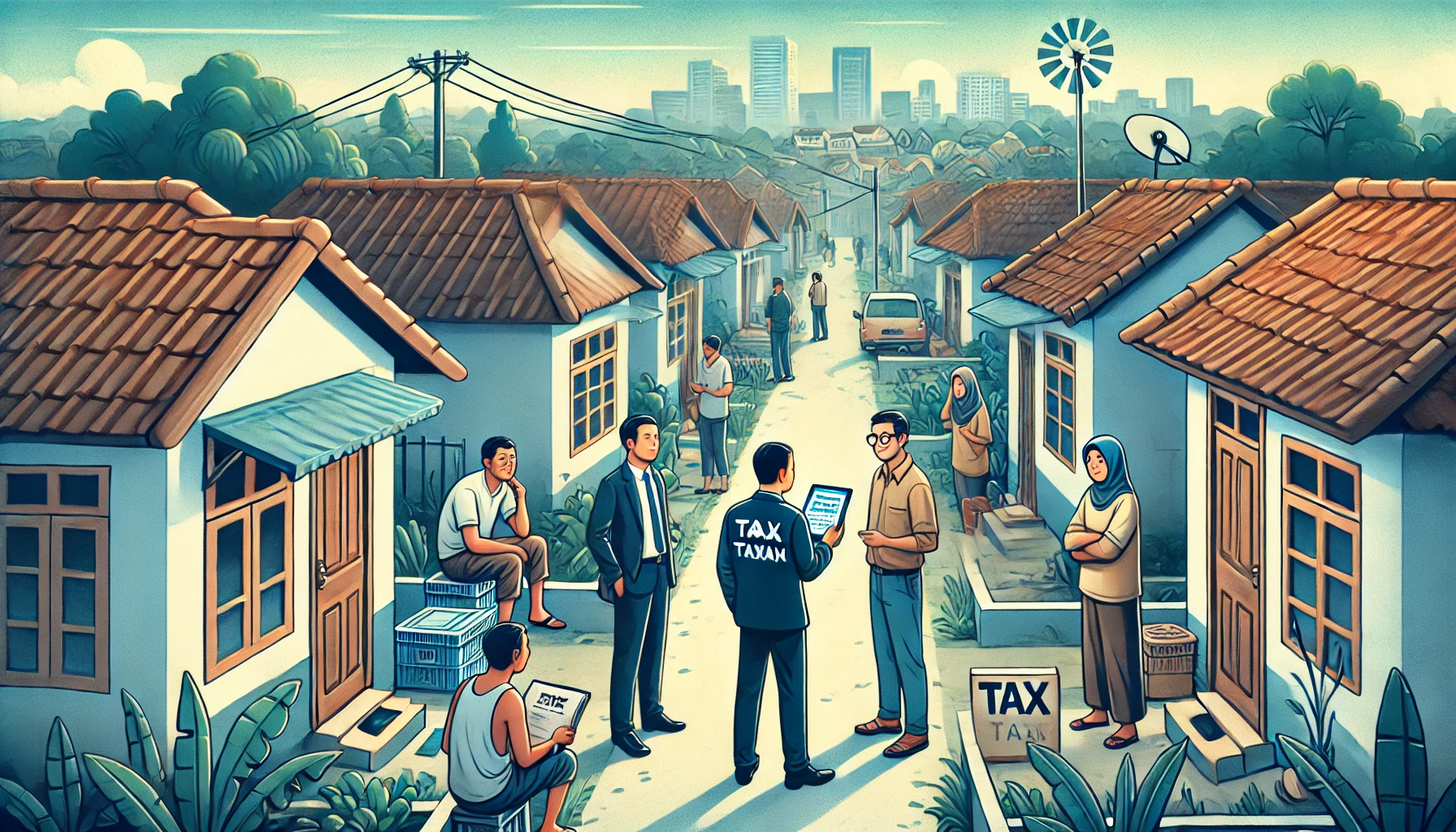
A recent Policy Research Working Paper published by the World Bank, authored by Paulo Antonacci from Duke University and Muhammad Khudadad Chattha from the World Bank, evaluates the effectiveness of a door-to-door tax enforcement strategy in Gorontalo, Indonesia. The study focuses on an initiative where tax officials visited properties to engage directly with property owners about their tax obligations in an attempt to improve tax compliance and revenue collection. The program ran from November 2020 to December 2021, with the tax officials explaining outstanding debts and payment processes to property owners, aiming to increase compliance. The authors used machine learning techniques to analyze administrative data and measure the program's impact, revealing insights about how such direct interventions could shape tax compliance in developing countries like Indonesia.
Direct Engagement Boosts Compliance Rates
The program was designed to address growing levels of tax evasion, particularly in the property sector, by having tax officials directly interact with property owners. This face-to-face approach was expected to foster greater compliance by making the tax payment process clearer and more personal. The study shows that the program had a substantial impact, with tax compliance increasing by 4.3% on the extensive margin, which refers to the proportion of taxpayers who paid, and by 5.1% on the intensive margin, representing the total amount of taxes collected. These improvements were particularly strong in the year following the program, as compliance continued to rise in 2022.
Lower-Value Properties Respond More Positively
One of the most significant findings of the research is that the door-to-door enforcement strategy was more effective among lower-value properties. Properties with smaller land areas and those that had some form of construction on them were more likely to respond positively to the tax officials' visits. In contrast, higher-value properties showed less sensitivity to these enforcement efforts, suggesting that wealthier property owners were less affected by direct engagement from tax authorities. This raises important questions about the potential regressive nature of data-driven tax enforcement strategies. In a system where enforcement is focused more on lower-value properties, there is a risk that poorer segments of the population might bear a disproportionate burden of tax compliance efforts, while wealthier individuals may evade more easily.
Sustained Impact Beyond the Initial Program
The study also reveals that the positive effects of the program persisted over time. In 2022, tax compliance continued to improve, with an overall increase of 6.1% compared to the previous year. While the researchers do not provide a definitive explanation for this sustained improvement, they speculate that the COVID-19 pandemic may have played a role in influencing taxpayer behavior. It's possible that the economic disruption caused by the pandemic led to changes in how people approached their tax obligations, and the effects of the door-to-door visits may have been more pronounced because of these unusual circumstances. The authors suggest that the program's impact could have been even greater in non-pandemic years, as the economic effects of the pandemic likely influenced the results.
Selection Bias in Visited Properties
The analysis of the program reveals that the properties visited by tax officials were generally more compliant even before the program began. These properties had better historical compliance records, lower total values, and smaller land areas compared to the properties that were not visited. This selection bias indicates that the program's implementation was not evenly distributed, with tax officials more likely to visit properties in neighborhoods with higher historical compliance rates. As a result, areas with lower compliance rates were less likely to be targeted by the program, which limited the overall effectiveness of the initiative. The researchers suggest that future programs could improve their results by focusing on properties with a higher likelihood of non-compliance, potentially increasing tax revenues even further.
The Role of Machine Learning in Tax Compliance
The use of machine learning techniques in this study is particularly notable. The authors employed advanced causal inference methods to control for differences between the visited and non-visited properties, which allowed them to isolate the effect of the door-to-door visits on tax compliance. This approach highlights the potential for machine learning to be used in evaluating tax enforcement programs, especially in settings where traditional methods may be less effective. The findings of the study suggest that data-driven strategies could be useful for tax authorities looking to improve compliance, but they also emphasize the need to carefully consider the distributional consequences of such approaches.
- FIRST PUBLISHED IN:
- Devdiscourse
ALSO READ
India economy expected to grow at 7 per cent in FY25: World Bank.
World Bank Revises India's Growth Forecast to 7%
World Bank Upgrades India's Growth Forecast to 7% for FY25, Highlights Resilience
World Bank Increases India's Growth Forecast Amid Agri Recovery and Rural Demand
GTRI Criticizes World Bank's Call for India to Join RCEP