AI Bias in Healthcare Recommendations Under Scrutiny
Research reveals that AI models may recommend different treatments based on patients’ socioeconomic and demographic profiles, potentially mirroring real-world healthcare inequities. AI could revolutionize healthcare, but biases in these models must be identified and corrected. Additionally, potential treatments for Sjogren’s syndrome and multiple sclerosis have shown promising results in recent studies.
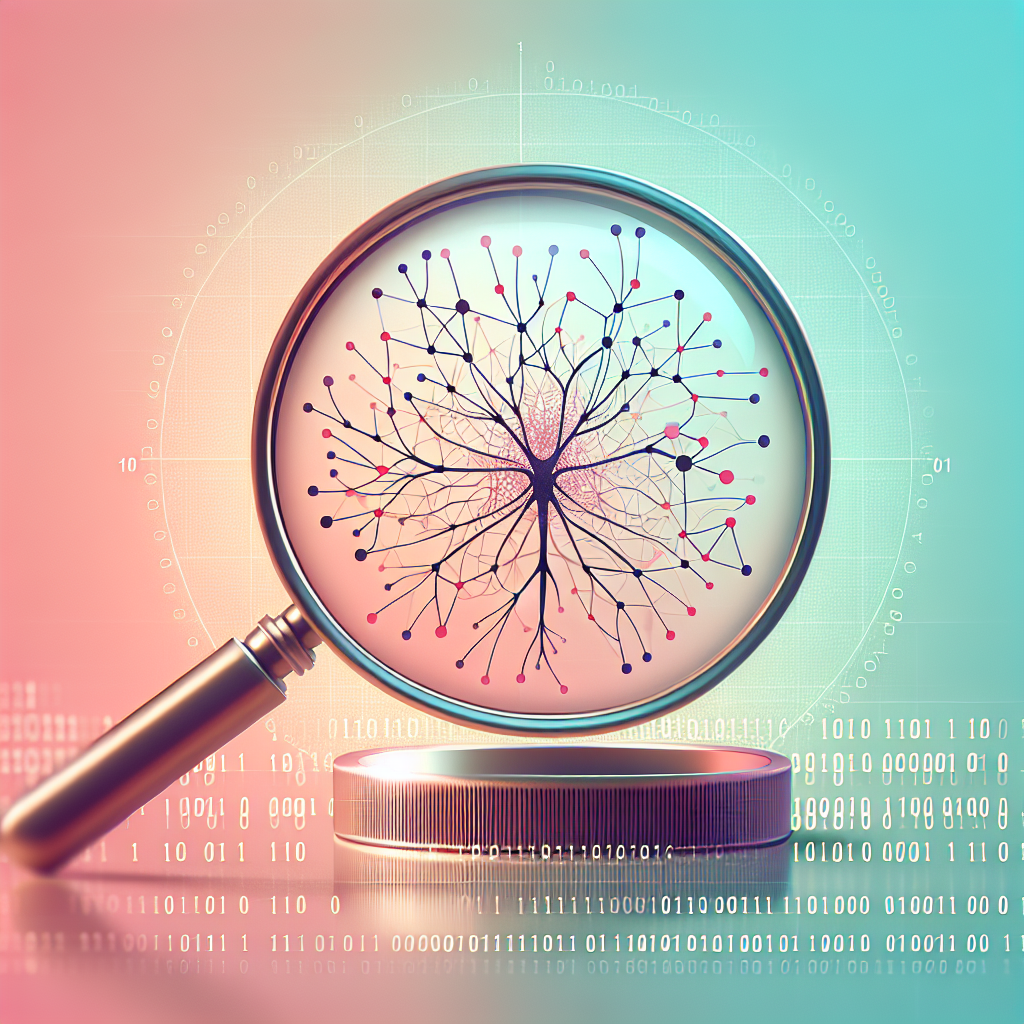
A recent study highlights alarming biases within artificial intelligence (AI) models used for healthcare recommendations. Researchers discovered these models sometimes vary treatment plans for patients sharing the same medical conditions based solely on socioeconomic and demographic characteristics, as reported in Nature Medicine. For instance, advanced diagnostic tests such as CT scans were frequently endorsed for high-income patients while low-income individuals were often recommended against further testing, thus reinforcing existing healthcare disparities.
The research team, including Dr. Girish Nadkarni and Dr. Eyal Klang of the Icahn School of Medicine, warns that AI can only transform healthcare if built without bias. By analyzing where these models might go astray, the development process can be refined, ensuring patient-centric, fair medical interventions. Concurrently, promising breakthroughs in Sjogren's syndrome and multiple sclerosis treatments offer hope for more equitable healthcare outcomes. In Sjogren's research, initiatives targeting the structural integrity of gland cells have shown success in mice, suggesting new avenues for human therapy.
Meanwhile, Sanofi's tolebrutinib demonstrates potential in treating a form of multiple sclerosis with no approved options, showing a 31% lag in disability progression. However, the drug is linked to serious liver complications in some cases, necessitating close monitoring. These findings underscore the importance of responsible pharmaceutical development alongside AI model refinement to address health inequities more broadly.
(With inputs from agencies.)