Building inclusive AI for healthcare: Transparency and trust at the core
The STANDING Together initiative employed a rigorous, multi-phase approach to formulate its recommendations. Over a two-year period, the researchers engaged in systematic reviews of existing literature, Delphi consensus processes, stakeholder consultations, and public forums. The initiative drew on the expertise of more th
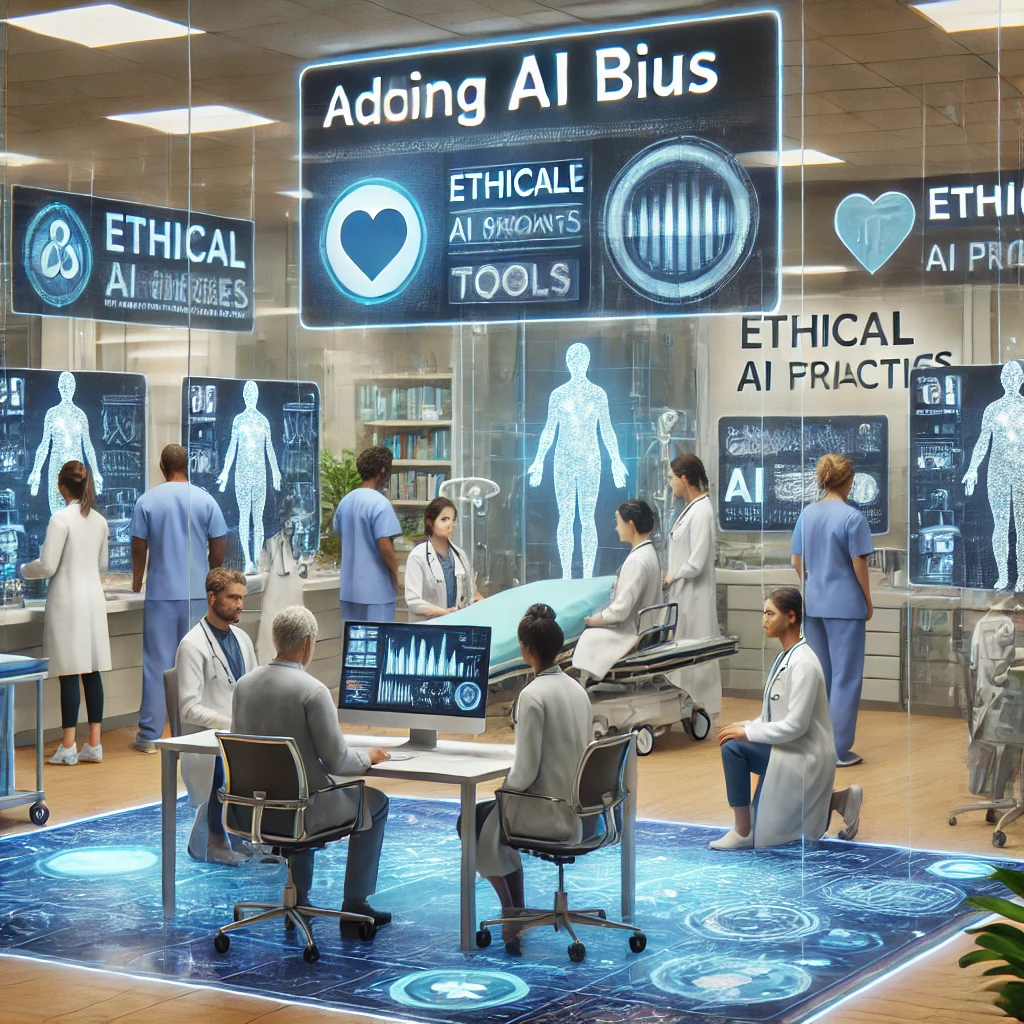
Artificial Intelligence (AI) holds transformative potential for healthcare, offering unprecedented capabilities in diagnostics, treatment planning, and operational efficiencies. Yet, as this technology integrates into healthcare systems, it risks perpetuating and amplifying existing inequities due to inherent biases in the datasets on which AI models are trained. These biases often arise from systemic disparities, incomplete data representation, and a lack of transparency in data usage. Addressing these challenges is imperative to harness AI’s full potential while ensuring equity and inclusivity in healthcare outcomes.
The paper “Tackling Algorithmic Bias and Promoting Transparency in Health Datasets: The STANDING Together Consensus Recommendations,” published in The Lancet Digital Health, Volume 7, Issue 1, e64 - e88, provides a comprehensive roadmap to confront these challenges. Authored by an international consortium of researchers, the study outlines a set of recommendations to bridge gaps in dataset documentation and promote fairness, transparency, and ethical AI use in healthcare.
Algorithmic bias in healthcare AI
Healthcare AI systems depend on vast datasets to train algorithms capable of making accurate predictions. However, the quality and diversity of these datasets are pivotal in determining the equity of the outcomes they produce. Historically, many health datasets have reflected structural inequities, with overrepresentation of certain populations and underrepresentation of others, such as racial minorities, women, and rural communities. This lack of diversity not only skews algorithmic predictions but also risks exacerbating health disparities by disadvantaging already vulnerable groups. For instance, AI systems trained predominantly on data from urban, affluent populations may fail to accurately diagnose conditions in rural or socio-economically disadvantaged communities.
Biases also arise when datasets do not adequately capture the complexity of real-world healthcare environments. Inadequate documentation of how data was collected, processed, and utilized further compounds this issue, making it difficult to assess the suitability of datasets for specific AI applications. Past instances of racial and gender bias in healthcare AI systems, such as algorithms prioritizing resource allocation based on biased cost-effectiveness metrics, underscore the urgency of addressing these issues. The STANDING Together initiative seeks to provide actionable strategies to mitigate such risks and establish trust in AI-driven healthcare technologies.
Developing the STANDING Together recommendations
The STANDING Together initiative employed a rigorous, multi-phase approach to formulate its recommendations. Over a two-year period, the researchers engaged in systematic reviews of existing literature, Delphi consensus processes, stakeholder consultations, and public forums. The initiative drew on the expertise of more than 350 participants from 58 countries, representing a diverse array of fields, including healthcare, AI development, policy, and bioethics.
The methodology focused on identifying key gaps in dataset transparency and algorithmic fairness. Researchers analyzed how current practices in data collection and use contribute to biases and examined frameworks for documenting datasets comprehensively. The recommendations were then refined through iterative feedback from stakeholders, ensuring that they were both theoretically robust and practically implementable. By categorizing their findings into guidelines for dataset documentation and principles for the ethical use of datasets, the initiative established a clear structure for addressing algorithmic bias at multiple stages of the AI lifecycle.
Key findings and recommendations
The STANDING Together recommendations emphasize the importance of creating inclusive datasets and promoting accountability throughout the development and deployment of AI systems. Dataset curators are encouraged to adopt transparent practices, such as providing detailed documentation that outlines the demographic composition of datasets, data sources, and potential limitations. This transparency allows users to evaluate the relevance and suitability of datasets for specific applications, ensuring that algorithms are trained on data that accurately represents the populations they aim to serve.
Addressing bias in underrepresented groups is another cornerstone of the recommendations. AI systems often fail marginalized populations because their needs and characteristics are not adequately captured in training data. By advocating for the inclusion of diverse demographic data, the recommendations aim to rectify this imbalance. For example, in regions where healthcare access is limited, datasets must incorporate information from rural and low-income populations to ensure that AI systems can deliver equitable outcomes.
The recommendations also call for robust accountability mechanisms in AI development. This includes routine testing of model performance across different demographic groups to identify and address disparities. Moreover, the initiative stresses the importance of interpretability in AI systems, ensuring that both clinicians and patients understand how algorithms generate their outputs. This transparency is critical for building trust and enabling informed decision-making.
Implications for healthcare stakeholders
Implementing the STANDING Together recommendations has profound implications for various stakeholders in the healthcare ecosystem. Dataset curators can improve the quality and inclusivity of data by adopting standardized documentation practices, which provide a comprehensive overview of dataset characteristics and limitations. For AI developers, integrating fairness principles into model training and evaluation processes ensures that algorithms are not only accurate but also equitable in their outcomes.
For policymakers and regulators, the recommendations offer a framework for evaluating and certifying AI health technologies. By establishing clear guidelines for dataset transparency and ethical AI use, regulatory bodies can promote accountability and safeguard public trust in AI systems. Additionally, the recommendations highlight the need for cross-sector collaboration to address systemic biases, emphasizing the role of public-private partnerships in fostering innovation and inclusivity.
Navigating Challenges
While the STANDING Together recommendations provide a comprehensive framework for addressing algorithmic bias, their implementation faces significant challenges. Developing inclusive datasets requires substantial investment in infrastructure and data collection, particularly in low- and middle-income countries where resources are limited. Harmonizing standards across diverse healthcare systems also poses a challenge, as varying legal, cultural, and ethical norms influence data collection and AI deployment practices.
Overcoming these barriers will require sustained collaboration among stakeholders. Policymakers must incentivize the adoption of these recommendations through funding and regulatory support, while healthcare providers and AI developers must embed fairness and transparency principles into their workflows. Education and training programs for healthcare professionals and data scientists are also essential for building the expertise needed to implement these guidelines effectively.
Looking ahead, future research should focus on scalable solutions to address resource disparities and improve the representativeness of health datasets. Advances in federated learning and other privacy-preserving technologies offer promising avenues for enabling data sharing without compromising patient confidentiality. By leveraging these innovations, stakeholders can build AI systems that are not only technically advanced but also ethically sound and socially equitable.
- FIRST PUBLISHED IN:
- Devdiscourse