Transforming healthcare: Real-time patient monitoring with 5G and AI integration
Remote patient monitoring has emerged as a cornerstone of modern healthcare, particularly with the growing need for continuous and reliable health assessments outside traditional clinical settings. However, existing RPM systems face significant challenges, such as high latency, limited bandwidth, and inadequate real-time processing capabilities. These shortcomings often result in delayed detection of critical health changes, which can have dire consequences for patient outcomes.
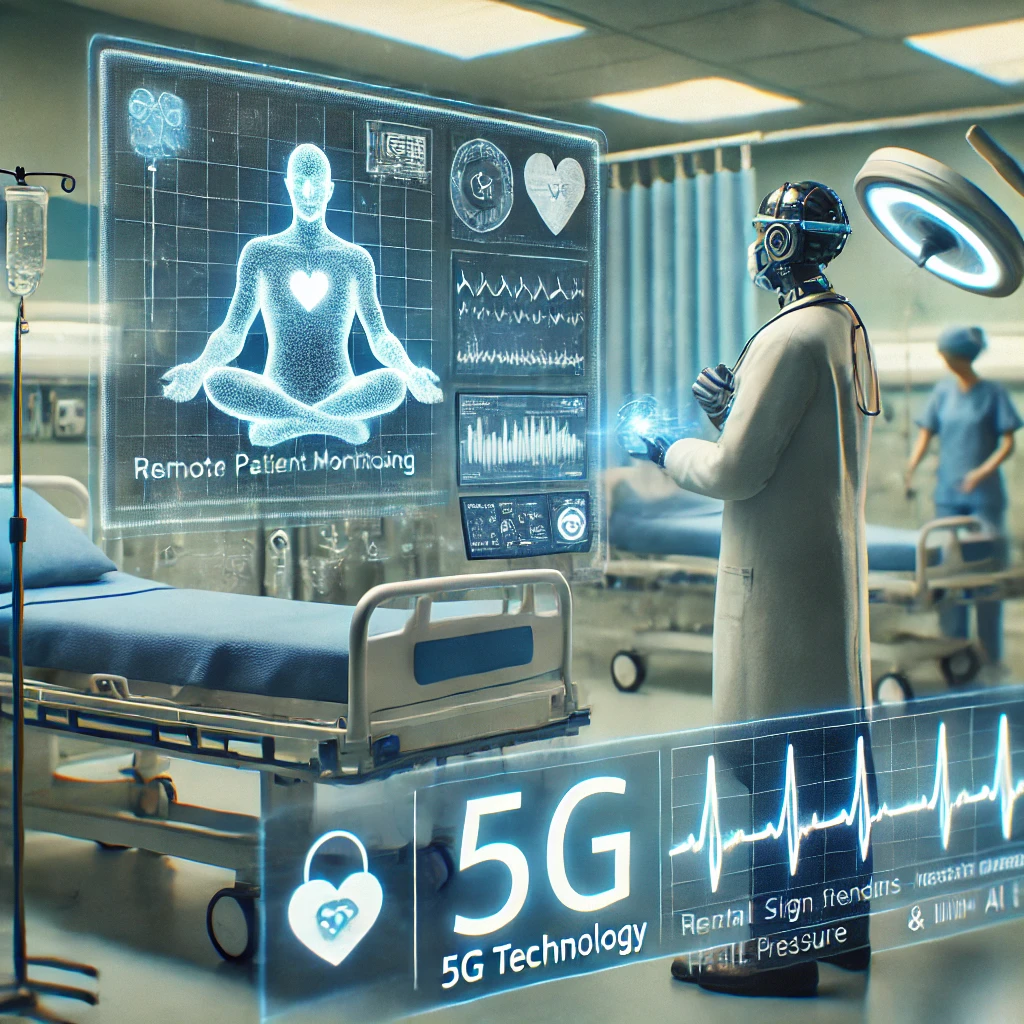
The fusion of cutting-edge technologies with healthcare is ushering in a transformative era, reshaping the way care is delivered and monitored. Among these innovations, remote patient monitoring (RPM) has emerged as a critical pillar of modern healthcare, enabling continuous, real-time assessment of patient health beyond the confines of traditional clinical settings. In the study “Real-Time Health Monitoring Using 5G Networks: A Deep Learning-Based Architecture for Remote Patient Care” by Iqra Batool, a novel framework is presented that leverages the power of 5G networks and deep learning to redefine how patient health is monitored in real-time. Available on arXiv, this research addresses the critical limitations of existing RPM systems, such as latency, bandwidth constraints, and lack of predictive accuracy, by introducing a hybrid CNN-LSTM architecture optimized for edge computing.
Addressing the challenges of remote patient monitoring
Remote patient monitoring has emerged as a cornerstone of modern healthcare, particularly with the growing need for continuous and reliable health assessments outside traditional clinical settings. However, existing RPM systems face significant challenges, such as high latency, limited bandwidth, and inadequate real-time processing capabilities. These shortcomings often result in delayed detection of critical health changes, which can have dire consequences for patient outcomes.
The study highlights how 5G technology, with its ultra-reliable low-latency communication (URLLC) capabilities, massive machine-type communication (mMTC), and enhanced mobile broadband (eMBB), can serve as a solution to these challenges. By integrating 5G networks with deep learning, the proposed system achieves unprecedented latency of 14.4 milliseconds and a prediction accuracy of 96.5% for various vital signs, including heart rate, blood pressure, and respiratory rate.
A deep learning framework for real-time analysis
The core of the proposed system is a hybrid deep learning model combining Convolutional Neural Networks (CNNs) and Long Short-Term Memory (LSTM) networks. This architecture is specifically designed to handle multivariate vital sign data while maintaining temporal accuracy and computational efficiency. The CNN component extracts meaningful features from raw data, while the LSTM layers capture long-term dependencies, ensuring accurate trend prediction and real-time analysis.
An attention mechanism further enhances the model’s performance by prioritizing the most critical temporal patterns in the data. This allows for precise forecasting of vital sign trends, enabling early detection of potential health deteriorations. The system also integrates a custom loss function that balances prediction accuracy with temporal consistency, ensuring reliable monitoring across diverse patient conditions.
The integration of 5G technology is a cornerstone of this research. The system employs network slicing to create dedicated communication channels for healthcare data transmission, ensuring consistent quality of service (QoS). This allows the system to maintain sub-millisecond latency, a critical requirement for real-time applications. Additionally, edge computing is utilized to preprocess data closer to the source, reducing the computational burden on central systems and further minimizing latency.
The research demonstrates how 5G’s capabilities enable the seamless transmission of high-frequency vital sign data without compromising reliability. By prioritizing healthcare data packets through advanced scheduling algorithms, the system ensures uninterrupted monitoring even during peak network usage.
Performance validation and implications
The proposed system was evaluated over three months using data from 1,000 patients in clinical settings. The results showcased its robustness, with an average mean absolute error (MAE) of less than 2.2% across all monitored vital signs. The system outperformed existing RPM solutions, achieving a 47% reduction in latency and a 4.2% improvement in prediction accuracy. These advancements underscore the feasibility of deploying the framework in real-world healthcare environments, where timely and accurate patient monitoring is critical.
The implications of this research are profound. By enabling real-time health monitoring with high precision, the system has the potential to revolutionize patient care, particularly in intensive care units, post-operative recovery, and chronic disease management. The predictive capabilities of the model allow for proactive medical intervention, reducing the risk of critical incidents and improving overall clinical outcomes.
Future directions and opportunities
While the study achieves significant milestones, it also highlights areas for further research. Expanding the framework to incorporate multimodal data sources, such as continuous glucose monitoring and oxygen saturation levels, could provide a more comprehensive view of patient health. Additionally, integrating privacy-preserving techniques and adaptive learning mechanisms would enhance the system’s applicability in diverse healthcare settings.
Another promising avenue is the exploration of federated learning to improve model performance across multiple facilities without compromising patient privacy. The integration of explainable AI techniques could further enhance clinical utility by providing interpretable insights into prediction decisions. These advancements would not only broaden the system’s scope but also strengthen its resilience and adaptability, ensuring it meets the dynamic needs of healthcare environments.
Furthermore, developing solutions for resource-constrained settings, such as rural or underserved areas, could extend the system’s reach to populations that often face barriers to advanced healthcare services. Addressing these challenges would make this technology a cornerstone of equitable and accessible global healthcare, empowering medical professionals and patients alike to achieve better health outcomes.
- FIRST PUBLISHED IN:
- Devdiscourse