AI-powered breakthroughs in Alzheimer’s detection: Advancing early diagnosis with LLMs
As the global burden of dementia grows, innovations like these promise a future where early and accurate detection is not only possible but accessible to all, bridging gaps in healthcare equity. Beyond Alzheimer’s, this research underscores the profound potential of AI in revolutionizing the diagnosis and management of neurodegenerative diseases, laying the groundwork for a smarter, more inclusive healthcare paradigm.
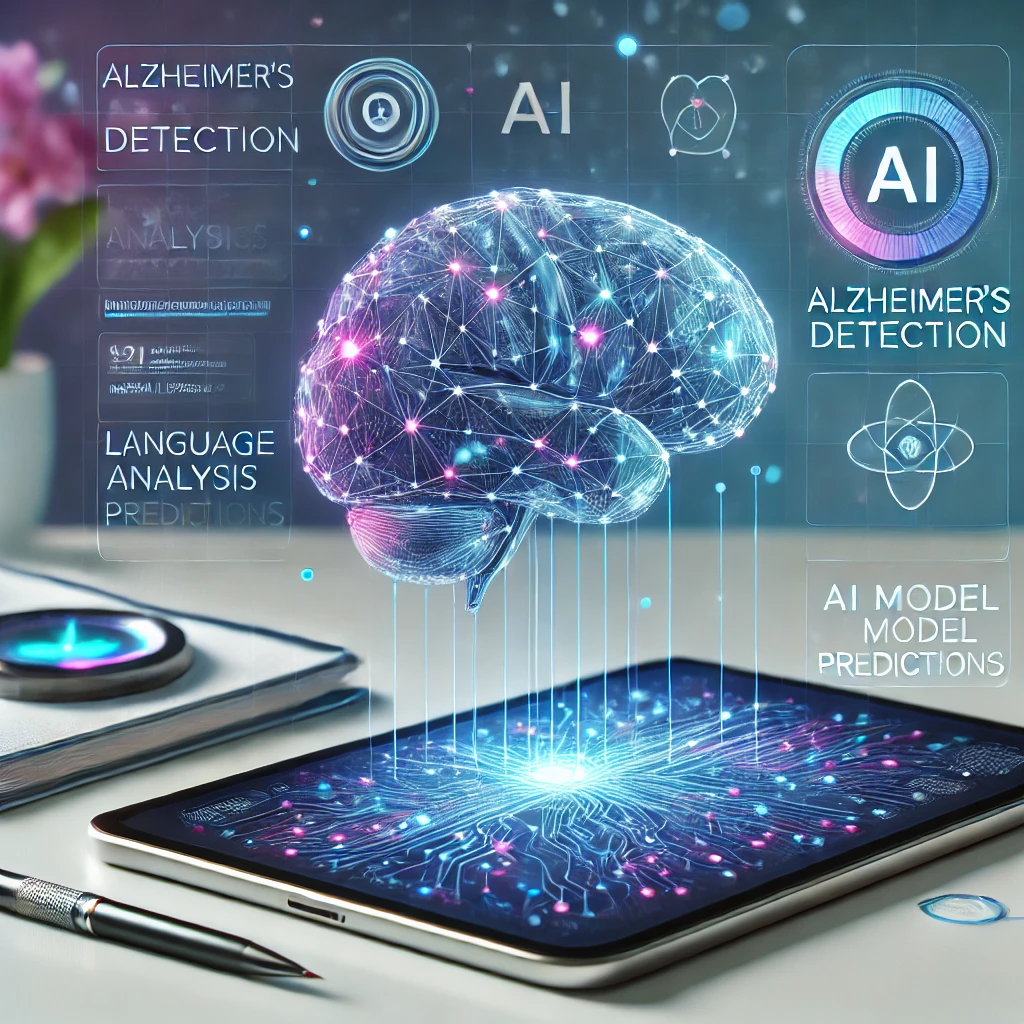
As the world faces a growing aging population, the prevalence of age-related neurodegenerative diseases like Alzheimer’s Disease (AD) is on the rise, posing a critical public health challenge. Globally, millions of people live with cognitive impairments, with Alzheimer’s being the most common form of dementia. Early and accurate detection of AD is vital for timely intervention and care. However, traditional diagnostic methods, which often involve clinical visits, neuropsychological testing, and invasive procedures, are resource-intensive and inaccessible to many, especially in low-resource settings.
In this context, digital health technologies have emerged as a promising avenue, offering innovative solutions to democratize healthcare. Speech analysis, in particular, has shown potential as a non-invasive method to detect cognitive impairments. Building on this foundation, a groundbreaking study titled "Alzheimer’s Disease Detection Based on Large Language Model Prompt Engineering" by Tian Zheng and colleagues introduces a novel approach that leverages the power of large language models (LLMs) to revolutionize Alzheimer’s diagnosis.
Harnessing the power of LLMs
This study, published by researchers from the Institute of Software, Chinese Academy of Sciences, and the University of Chinese Academy of Sciences, presents a state-of-the-art methodology that combines advanced artificial intelligence techniques with speech-based data to detect cognitive impairments. By employing the LLAMA2 language model and innovative techniques such as prompt engineering and LoRA (Low-Rank Adaptation), the researchers have not only enhanced diagnostic accuracy but also addressed the high computational demands associated with LLMs.
The research utilized the ADReSS2020 dataset, a widely recognized benchmark in Alzheimer’s research, consisting of transcribed speech samples. These samples include verbal descriptions of images by participants, categorized as either healthy or exhibiting Alzheimer’s-related impairments. The study implemented meticulous preprocessing to ensure data consistency and quality, creating a strong foundation for model training.
The researchers explored three primary methodologies to optimize model performance:
-
Prompt Learning with LLAMA2: Prompt engineering techniques were applied to tailor the LLM for Alzheimer’s detection. The model was fine-tuned using prompts that aligned with the task, such as phrases designed to assess speech disorders or dementia. LLAMA2’s autoregressive architecture, capable of generating coherent sequences, proved instrumental in analyzing nuanced linguistic patterns.
-
LoRA Fine-Tuning: To mitigate the computational burden of large models, the study employed LoRA, a technique that inserts low-rank matrices into the model’s architecture. This reduced the number of parameters requiring updates, making the approach resource-efficient without compromising accuracy.
-
Conditional Learning: This approach involved calculating the probability of text belonging to specific categories based on labels. However, the model’s performance in this area was limited by the small dataset size and lack of label diversity, underscoring the need for further refinement.
The LLAMA2-based prompt engineering model achieved a remarkable classification accuracy of 81.31%, outperforming the BERT-based baseline model, which achieved 76.85%. This improvement underscores the superior capabilities of generative models like LLAMA2 in handling complex text sequences and extracting intricate linguistic features. The flexibility of LLAMA2 in adapting to new tasks was particularly advantageous in capturing the subtle patterns characteristic of Alzheimer’s-related speech.
However, the study also highlighted challenges. While LLAMA2 excelled in prompt learning, its performance in conditional learning was suboptimal, achieving an accuracy of only 57.41%. This discrepancy was attributed to the limited dataset and the computational complexity of generative tasks. The findings emphasize the importance of dataset diversity and the need for advanced optimization techniques to unlock the full potential of LLMs.
Implications for healthcare
This research marks a significant leap forward in leveraging artificial intelligence for healthcare. By demonstrating the efficacy of combining large language models with innovative fine-tuning techniques, the study paves the way for more accessible and scalable solutions for Alzheimer’s detection. The non-invasive nature of speech-based analysis, coupled with the efficiency of LoRA, makes this approach particularly well-suited for deployment in resource-constrained environments.
Moreover, the study opens avenues for the application of LLMs in diagnosing other neurodegenerative diseases. The ability to analyze linguistic and acoustic features with high precision has implications beyond Alzheimer’s, potentially transforming how conditions like Parkinson’s disease and aphasia are diagnosed.
Expanding horizons: Future directions
The study marks a pivotal milestone in the application of artificial intelligence to healthcare, yet the researchers acknowledge opportunities for further advancement. The limited size and linguistic uniformity of the ADReSS2020 dataset impose constraints on the model’s generalizability. Future efforts will prioritize expanding datasets to encompass diverse languages and dialects, enabling broader applicability and inclusivity. Additionally, refining techniques such as prompt tuning and conditional learning remains a crucial focus, aiming to overcome current limitations and elevate diagnostic precision.
The methodologies explored in this research are envisioned to extend to other datasets and languages, including Chinese, further broadening the scope of application. By addressing these challenges, the researchers aim to enhance the model's robustness and utility, ensuring its effectiveness in varied real-world healthcare contexts.
As the global burden of dementia grows, innovations like these promise a future where early and accurate detection is not only possible but accessible to all, bridging gaps in healthcare equity. Beyond Alzheimer’s, this research underscores the profound potential of AI in revolutionizing the diagnosis and management of neurodegenerative diseases, laying the groundwork for a smarter, more inclusive healthcare paradigm.
- FIRST PUBLISHED IN:
- Devdiscourse