AI takes the lead: A breakthrough in ovarian cancer diagnosis
The study represents a monumental advancement in the use of AI for ovarian cancer diagnosis. By significantly outperforming human examiners and demonstrating robust generalization across diverse settings, AI models have the potential to revolutionize medical diagnostics.
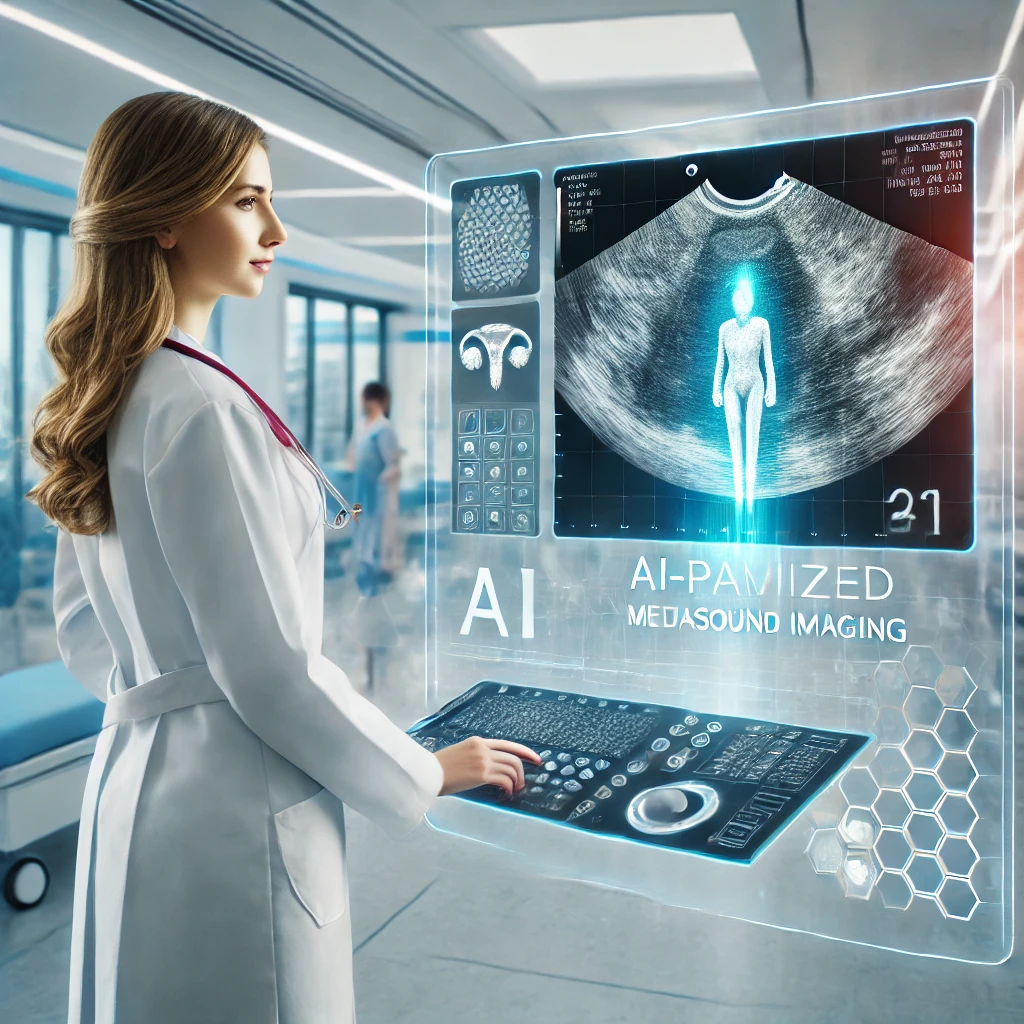
The field of medical diagnostics is undergoing a transformative shift, with artificial intelligence (AI) at its forefront. One particularly promising development is the use of AI in diagnosing ovarian cancer, a condition where early and accurate detection is crucial for effective treatment. Published in Nature Medicine, the study titled "International Multicenter Validation of AI-Driven Ultrasound Detection of Ovarian Cancer" provides a detailed look into how AI can outperform human expertise in identifying ovarian lesions, marking a significant step forward in medical imaging.
Diagnosing ovarian cancer has long been a challenge due to the reliance on subjective assessments by expert ultrasound examiners, who are often in short supply. Inconsistent diagnostic accuracy among less experienced examiners further exacerbates this issue, leading to delayed diagnoses or unnecessary surgical interventions. Compounding these challenges, biopsies are contraindicated for suspected ovarian malignancies due to the risk of spreading cancerous cells. Against this backdrop, AI emerges as a powerful tool capable of enhancing diagnostic accuracy while optimizing clinical workflows.
A comprehensive approach to validation
The study, led by Filip Christiansen and a global team of researchers, represents the first comprehensive validation of AI models in this domain across diverse international settings. The researchers used transformer-based neural networks, trained on a vast dataset of 17,119 ultrasound images from 3,652 patients across 20 centers in eight countries. This dataset included images from 21 different ultrasound systems, reflecting real-world variability in clinical environments. The team employed a leave-one-center-out cross-validation approach, rigorously testing the AI models on unseen data to ensure their generalizability.
The results were remarkable. The AI models achieved an F1 score of 83.50%, significantly outperforming expert human examiners (79.50%) and non-experts (74.10%). Unlike human assessments, which often vary due to examiner experience and subjective interpretation, the AI models consistently demonstrated robust diagnostic performance across different patient populations, ultrasound systems, and histological diagnoses. This reliability was evident in the models’ ability to generalize effectively across centers, even those not included in the training data.
Reducing diagnostic errors and enhancing efficiency
One of the most striking outcomes of the study was the significant reduction in diagnostic errors achieved by the AI models. Compared to expert examiners, the AI models lowered the false negative rate by 39.27% and the false positive rate by 35.53%. These improvements are critical in reducing missed cancer diagnoses and preventing unnecessary surgeries, both of which can have profound implications for patient outcomes and healthcare costs.
The study also highlighted the potential of AI to alleviate the shortage of expert ultrasound examiners. In a simulated clinical triage setting, the integration of AI as a diagnostic assistant reduced referrals to experts by 63%. By serving as a second reader in the diagnostic process, AI not only enhanced accuracy but also optimized resource allocation, enabling healthcare systems to manage patient loads more effectively.
Moreover, the AI models demonstrated resilience in handling challenging cases. While human examiners often showed a sharp decline in accuracy when uncertain, the AI maintained a high level of performance, showcasing its potential to support clinical decisions in complex scenarios. The models were also well-calibrated, ensuring that their predictions aligned closely with the actual risk of malignancy, which is crucial for building trust among clinicians and facilitating their integration into practice.
Limitations, broader implications, and the path forward
Despite these promising results, the study acknowledged certain limitations. Being retrospective in nature, it relied on pre-existing ultrasound images and lacked the prospective validation necessary for clinical implementation. The researchers emphasized the need for further studies to assess the performance of AI in less controlled environments, such as settings with lower cancer prevalence or images obtained by less experienced examiners. Nevertheless, the findings lay a strong foundation for future research and the eventual adoption of AI-driven diagnostics in clinical practice.
The implications of this research extend beyond ovarian cancer. By demonstrating the effectiveness of transformer-based neural networks in medical imaging, the study paves the way for similar applications in diagnosing other conditions. As healthcare systems worldwide grapple with resource constraints and rising patient demands, the integration of AI offers a scalable solution to enhance diagnostic accuracy and accessibility. The versatility of these AI models highlights their potential to address diagnostic challenges across diverse medical fields, transforming the landscape of healthcare delivery.
In conclusion, the study represents a monumental advancement in the use of AI for ovarian cancer diagnosis. By significantly outperforming human examiners and demonstrating robust generalization across diverse settings, AI models have the potential to revolutionize medical diagnostics. As we move closer to integrating AI into routine clinical practice, this research underscores the transformative power of technology in improving patient outcomes and addressing global healthcare challenges. This marks a pivotal moment in the journey toward AI-assisted medicine, offering hope for more equitable and effective diagnostic solutions worldwide.
- FIRST PUBLISHED IN:
- Devdiscourse