Improving AI accuracy for critical healthcare decisions
As LLMs become increasingly integrated into medical practice, this study provides a crucial framework for evaluating and improving their reliability.
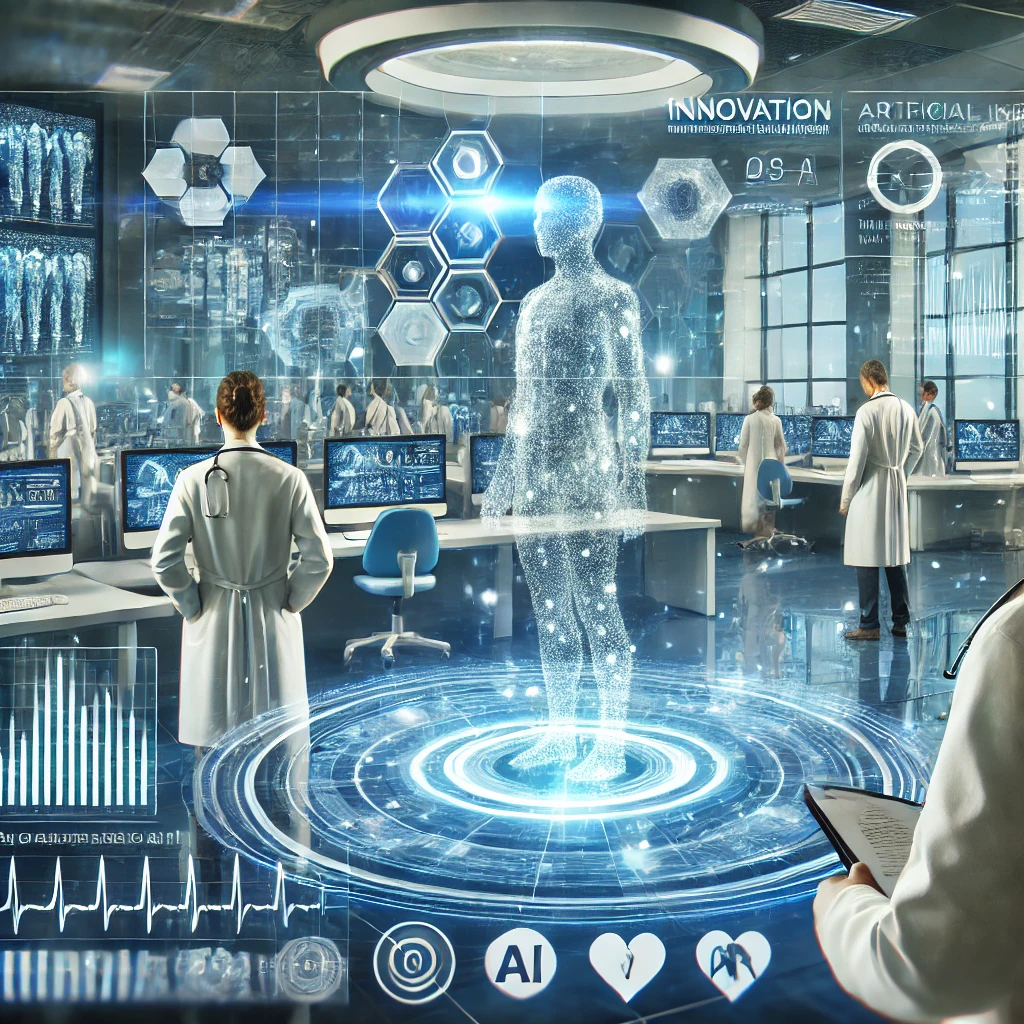
The integration of Artificial Intelligence (AI) into healthcare has sparked a paradigm shift, offering solutions that range from predictive diagnostics to personalized treatment plans. Among these advancements, Large Language Models (LLMs) have emerged as transformative tools. With their ability to process and analyze complex datasets, these models promise to revolutionize clinical decision-making. However, despite their potential, questions around reliability and accuracy in probabilistic predictions have remained a barrier to their full adoption in medical settings.
In a groundbreaking study titled "Probabilistic Medical Predictions of Large Language Models," published in npj Digital Medicine, researchers explore the promise and pitfalls of using large language models (LLMs) in medical applications. The study, conducted by experts from Harvard Medical School and affiliated institutions, evaluates the reliability of probabilistic predictions made by LLMs, a critical component for integrating AI into healthcare decision-making.
The dual challenge of probabilities
The authors focused on two types of probability outputs generated by LLMs: explicit probabilities and implicit probabilities. Explicit probabilities are user-friendly, generated directly through text-based prompts like, “Please provide the probability along with your prediction.” However, their reliability often falters due to the inherent numerical reasoning limitations of LLMs. Implicit probabilities, derived from the likelihood of specific token predictions, offer a more statistically sound alternative but remain challenging to extract and apply across diverse scenarios.
The study’s extensive evaluation spanned six advanced open-source LLMs and five medical datasets, comparing these probability types under various conditions. Findings revealed that implicit probabilities consistently outperformed explicit ones in metrics like discrimination, precision, and recall. This discrepancy was more pronounced in smaller models and datasets with imbalanced labels, raising concerns about the widespread use of explicit probabilities in clinical settings.
Performance
The study’s evaluation included advanced LLMs like Meta-Llama-3.1-70B, Mistral-Large, and Qwen2-72B. Among these, Meta-Llama-3.1-70B achieved the highest accuracy across datasets, including the United States Medical Licensing Examination (USMLE) and the MGB-SDoH, a dataset based on electronic health records.
Interestingly, smaller models demonstrated a greater disparity between explicit and implicit probabilities, highlighting the limitations of less sophisticated architectures. This discrepancy was amplified in datasets with imbalanced labels, where explicit probabilities were more prone to errors. For instance, in scenarios with rare outcomes, the explicit probabilities often failed to reflect the true likelihood, potentially skewing clinical decisions.
The researchers also found that even large models tended to overconfidently polarize their predictions, regardless of their correctness. While implicit probabilities offered a more nuanced distribution, explicit probabilities often hovered around extremes like 90% or 10%, undermining their reliability.
Implications for healthcare
The findings underscore the need for cautious interpretation of AI predictions in clinical settings. Explicit probabilities, while easy to implement, can amplify biases and mislead users about the confidence of a model's predictions. On the other hand, implicit probabilities, though more accurate, require further refinement and better integration into user workflows.
To bridge this gap, the study advocates for developing hybrid approaches that combine the flexibility of explicit probabilities with the statistical rigor of implicit ones. Fine-tuning LLMs to improve numerical reasoning and probabilistic estimation could pave the way for safer and more effective AI applications in healthcare.
A path forward
As LLMs become increasingly integrated into medical practice, this study provides a crucial framework for evaluating and improving their reliability. By highlighting the limitations of current probabilistic approaches, the authors encourage ongoing research and innovation to make AI a trustworthy ally in clinical decision-making.
This research not only advances the field of medical AI but also sets a benchmark for other domains requiring high-quality probabilistic predictions. As healthcare systems worldwide grapple with the dual challenges of innovation and accountability, studies like this illuminate the path toward ethical and effective AI deployment.
- FIRST PUBLISHED IN:
- Devdiscourse