Blending Data and Machine Learning: A New Era for Early Disease Outbreak Detection
The review explores how combining conventional health data with real-time social media and machine learning methods enhances early disease outbreak detection, providing faster, more accurate public health responses. Integrating diverse data sources offers critical improvements in monitoring and forecasting epidemic trends.
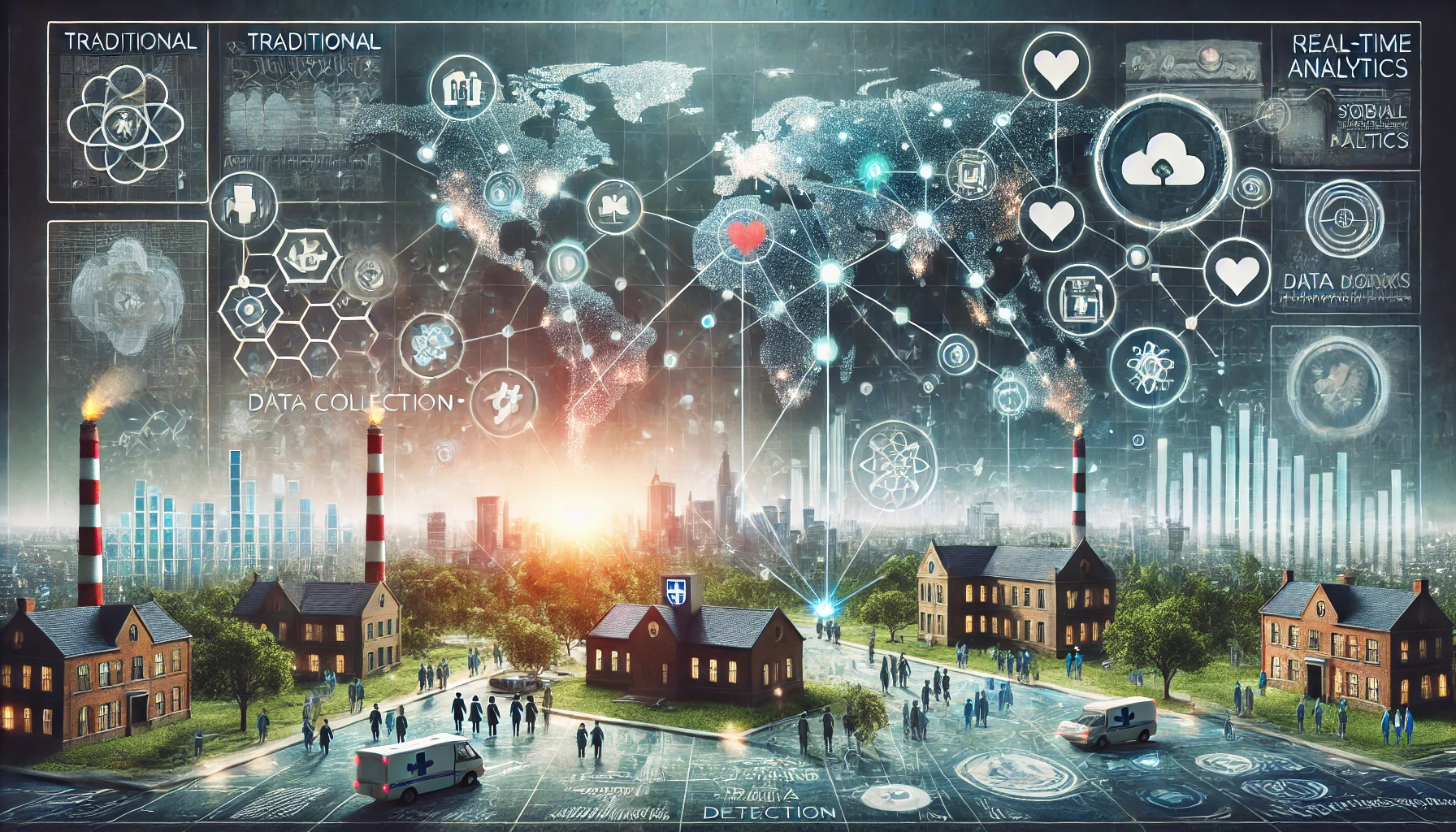
Research by Ghazaleh Babanejaddehaki, Aijun An, and Manos Papagelis from York University’s Department of Electrical Engineering and Computer Science explores modern methods for detecting and forecasting disease outbreaks, emphasizing the potential impact of early detection systems in reducing public health risks. As infectious disease outbreaks pose severe threats to global health and stability, timely identification is crucial. To this end, health authorities worldwide have developed surveillance systems, with contributions spanning clinical institutions, local and federal agencies, and international entities. In recent years, social media and internet data have emerged as supplementary sources, aiding traditional systems in the real-time tracking of disease trends. This review primarily examines studies conducted between 2015 and 2022, analyzing a range of techniques, including time series methods and machine learning, that utilize data from conventional sources like hospital records and global health organizations, as well as informal sources such as social media and online search trends. The goal is to improve outbreak detection by integrating diverse data types, harnessing their combined potential to aid in early warning and epidemic management.
Traditional Surveillance Systems and Their Limitations
In the traditional approach to epidemic detection, healthcare systems rely heavily on structured datasets from institutions like the World Health Organization (WHO), national health departments, hospital records, and pharmacy logs. Such conventional data sources allow a foundational view of disease patterns, albeit with some limitations. For instance, public health data can suffer from delays due to the validation processes and bureaucratic hurdles, which can limit timeliness. Thus, in situations where early intervention could save lives, these methods may fall short. Recognizing the need for faster systems, researchers have increasingly turned to online platforms as supplementary data sources. Social media platforms, such as Twitter, along with internet search engines, have proven particularly valuable for real-time tracking of health trends. These platforms allow public health authorities to monitor user behaviors, such as search queries related to symptoms and treatments, which can act as early indicators of outbreaks. For instance, projects like Google Flu Trends and Twitter Disease Surveillance utilize search and social media data to identify disease spread in near real-time, thereby providing additional layers of information to aid rapid response. The review explains that while WHO states that informal sources like social media can provide early indicators for over 60% of epidemics, these sources are typically more useful when combined with conventional data, as they may lack specificity or sensitivity when used alone.
Combining Classic and Modern Approaches for Forecasting
The analytical methods applied to both conventional and internet-based data span traditional statistical techniques and advanced machine learning models. Classical statistical methods, such as ARIMA (Auto-Regressive Integrated Moving Average), Holt-Winters, SARIMA (Seasonal ARIMA), and CUSUM (Cumulative Sum Control Chart) have long been used for forecasting time series data like stock prices or disease counts. These models are suitable for capturing general patterns, such as seasonality, and can be effective in tracking diseases with well-known periodic patterns. However, they often lack the flexibility required to address the non-linear patterns seen in more complex outbreak data. To enhance prediction accuracy, researchers are increasingly adopting machine learning techniques, especially in analyzing non-traditional data sources. Social media data, for example, can exhibit rapid, dynamic shifts that require adaptive models to handle their complexity. Machine learning algorithms like regression trees, support vector machines (SVM), and deep learning models such as LSTM (Long Short-Term Memory) networks, have shown promising results in identifying outbreaks from internet data by capturing intricate temporal relationships within the data. LSTM, in particular, is valued for its ability to process sequences, making it highly suitable for time series analysis in predicting case counts and outbreak growth rates.
Using Social Media and Internet Data to Track Outbreaks
The review highlights several recent studies in which social media data were successfully used in outbreak detection models. For instance, one model applied search and social media data to forecast H7N9 cases in China, showing high predictive accuracy by correlating search index data with confirmed cases. In another example, researchers analyzed Twitter data on influenza, utilizing machine learning classifiers like SVM, Naïve Bayes, and Random Forest to categorize disease-related tweets. Such models demonstrated a significant correlation between Twitter mentions and real-world case counts, proving the feasibility of using social media as a data source for real-time epidemic intelligence. Moreover, hybrid approaches, which combine traditional statistical methods with machine learning, are proving to be particularly effective. These approaches leverage spatiotemporal models that account for both time and location, thus enhancing the predictive power of outbreak models. For example, hybrid models using Markov switching and Bayesian frameworks have been applied to seasonal flu data, helping to capture complex spatial-temporal patterns. The results indicate that combining social media data with conventional health sources yields better accuracy, aiding in timely public health interventions.
Challenges in Data Accuracy and Integration
While the review identifies significant advancements, it also highlights challenges such as data privacy, the handling of massive unstructured data, and ensuring data relevance. Social media data is often noisy and can contain biases, making it difficult to extract actionable insights. Additionally, interpreting internet search trends remains complex, as people may search for symptoms or diseases for various reasons, which may not always indicate an outbreak. Thus, future research may focus on refining hybrid models that incorporate both social and conventional data, especially those that can adapt to varying epidemic patterns. The authors emphasize the need for sophisticated algorithms capable of integrating vast and disparate data sources, which could enhance the responsiveness and accuracy of early warning systems.
Conclusion: The Path Forward in Outbreak Detection
The review concludes by underscoring the promise of machine learning and hybrid models in revolutionizing public health surveillance and contributing to faster, more effective outbreak responses. As public health threats evolve, the integration of diverse data sources and analytical methods will be crucial for developing reliable early warning systems. Machine learning and hybrid models, by capturing both established trends and emerging signals, offer the potential to address the unique challenges posed by each outbreak and to empower health authorities with faster, data-driven insights.
- READ MORE ON:
- public health
- global health
- World Health Organization
- ARIMA
- SARIMA
- CUSUM
- LSTM
- H7N9
- FIRST PUBLISHED IN:
- Devdiscourse
ALSO READ
Justice Nariman Labels Ramjanmabhoomi Verdict a 'Travesty'
Justice Nariman Critiques Ramjanmabhoomi-Babri Masjid Verdict: A Call for Secularism
Sabarimala Temple's Record Sale of Offerings Amidst Pilgrim Surge
Safe Zone Project Enhances Pilgrimage Safety on Sabarimala Route
Tragedy Strikes: Car Hits Sabarimala Pilgrims in Kanamala