High-Accuracy Malaria Detection System: Deep Learning Model Accessible via Web Interface
Researchers from Addis Ababa University, Marquette University, and KU Leuven have developed a highly accurate automated web-based malaria detection system using deep learning models, achieving up to 97% accuracy and offering real-time diagnostic capabilities via a web interface. This innovative approach aims to improve malaria diagnosis, particularly in resource-limited settings, by providing a scalable and reliable solution.
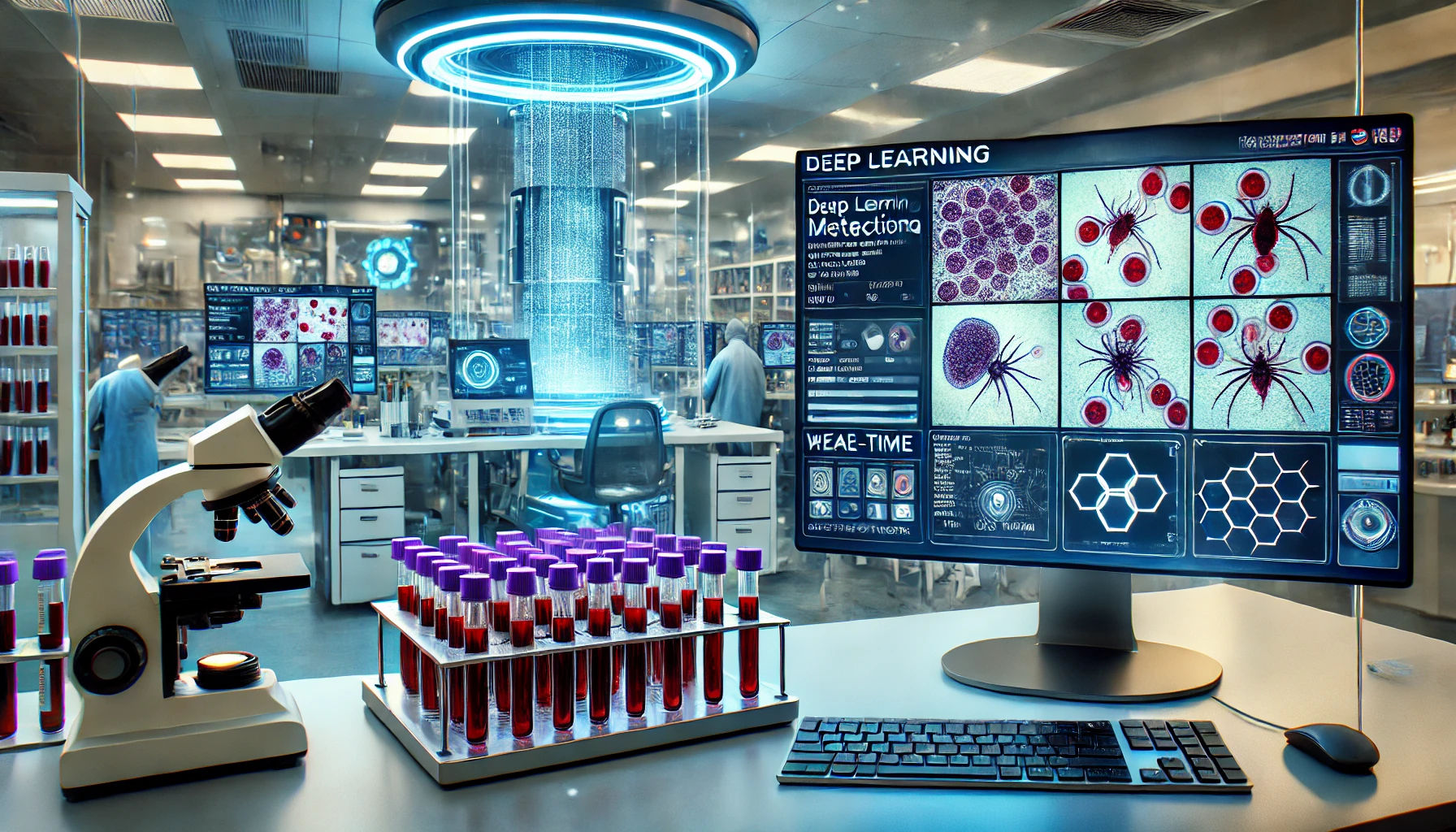
A groundbreaking study led by Abraham G. Taye and his team from the Center of Biomedical Engineering at Addis Ababa University, the Opus College of Engineering at Marquette University, and the Leuven Center for Affordable Healthcare Technology at KU Leuven, focuses on developing an automated web-based malaria detection system leveraging machine learning and deep learning techniques. Malaria remains a significant global health challenge, with millions at risk and hundreds of thousands succumbing to the disease annually, particularly in Africa. The conventional diagnostic methods, such as microscopic examination of blood smears, are often labor-intensive, error-prone, and require significant expertise.
The research aims to enhance the accuracy and efficiency of malaria detection through the application of deep learning models. The team formulated a deep learning technique using traditional Convolutional Neural Networks (CNNs) and transfer learning models, including VGG19, InceptionV3, and Xception. These models were trained on datasets from the National Institutes of Health (NIH), consisting of over 27,000 images categorized into parasitized and healthy samples. During testing, the deep CNN models demonstrated remarkable accuracy, with the highest reaching 97%. The Xception model followed closely with an accuracy of 95%, while the Support Vector Machine (SVM) model achieved 83%. The implementation of transfer learning, which utilizes pre-trained models and fine-tunes them for specific tasks, proved highly effective in improving detection accuracy.
Automated System for Real-Time Detection
The automated system is accessible via a web interface, allowing users to upload blood smear images for real-time malaria detection. This approach aims to mitigate the limitations of manual diagnostics by providing a faster, more reliable, and scalable solution. The study underscores the critical need for early and accurate detection of malaria to facilitate prompt treatment, particularly in vulnerable populations such as children and pregnant women. It highlights the potential of deep learning technologies to revolutionize malaria diagnosis, especially in resource-limited settings. The web-based platform, powered by TensorFlow.js, offers an innovative tool that can be deployed widely, enhancing the reach of malaria diagnostics.
Enhancing Diagnostic Accuracy and Efficiency
The system's architecture includes data preprocessing steps such as image resizing, normalization, and augmentation to optimize model performance. The models were evaluated using various performance metrics, including accuracy, precision, recall, and F1-score, ensuring their robustness and reliability. The researchers also emphasize the significance of integrating user feedback into the system's development, ensuring it meets the practical needs of healthcare professionals. The system's deployment and testing in real-world settings revealed potential benefits, including increased diagnostic accuracy and reduced burden on medical personnel. However, challenges such as the need for reliable internet connectivity and potential biases in the system were also identified.
Training and Validating Deep Learning Models
In the development of this system, several key aspects were addressed. The deep learning models were meticulously trained and validated using a comprehensive dataset from the NIH. The preprocessing steps included data augmentation to increase the diversity of the training data and normalization to ensure consistency in the input images. The CNN architecture was designed to learn intricate patterns in the images, which are crucial for accurately distinguishing between parasitized and healthy blood cells. Transfer learning models, particularly those like VGG19, InceptionV3, and Xception, were fine-tuned for the specific task of malaria detection, leveraging their pre-trained weights from large-scale image datasets to enhance performance.
Revolutionizing Malaria Diagnosis
The significance of this study lies in its potential impact on malaria diagnosis and treatment. By developing an automated system, the researchers aim to improve the speed and accuracy of detecting malaria parasites, which can lead to faster diagnoses and timely treatments. This is particularly important in malaria-endemic regions where access to experienced medical professionals and high-quality diagnostic tools may be limited. The automated system also aims to alleviate the workload of healthcare professionals, allowing them to focus on other critical tasks. One of the major contributions of this research is the integration of the automated detection system into a web-based platform. This platform enables users to upload blood smear images and receive instant diagnostic results, making it a valuable tool for remote and resource-limited settings.
Innovative Web-Based Platform
The use of TensorFlow.js in the web application allows for real-time processing and analysis of images directly in the browser, eliminating the need for specialized software or hardware. This accessibility and ease of use make the system particularly suitable for widespread adoption. Overall, the study presents a significant advancement in the field of automated malaria detection, showcasing the power of machine learning and deep learning in enhancing healthcare outcomes. The research paves the way for further developments in automated diagnostic systems, with the potential to save lives and improve healthcare delivery in malaria-endemic regions. The findings highlight the effectiveness of deep learning models and transfer learning techniques in medical image analysis and underscore the importance of continued innovation and research in this area. By addressing the limitations of traditional diagnostic methods and providing a scalable, reliable, and accessible solution, this study represents a crucial step forward in the global fight against malaria.
- FIRST PUBLISHED IN:
- Devdiscourse