A smarter way to learn: Harnessing machine learning for personalized programming education
Personalized approaches informed by physiological data could revolutionize areas like workforce training, mental health interventions, and human-computer interaction. By tailoring experiences to individual profiles, this technology has the potential to improve outcomes in numerous fields.
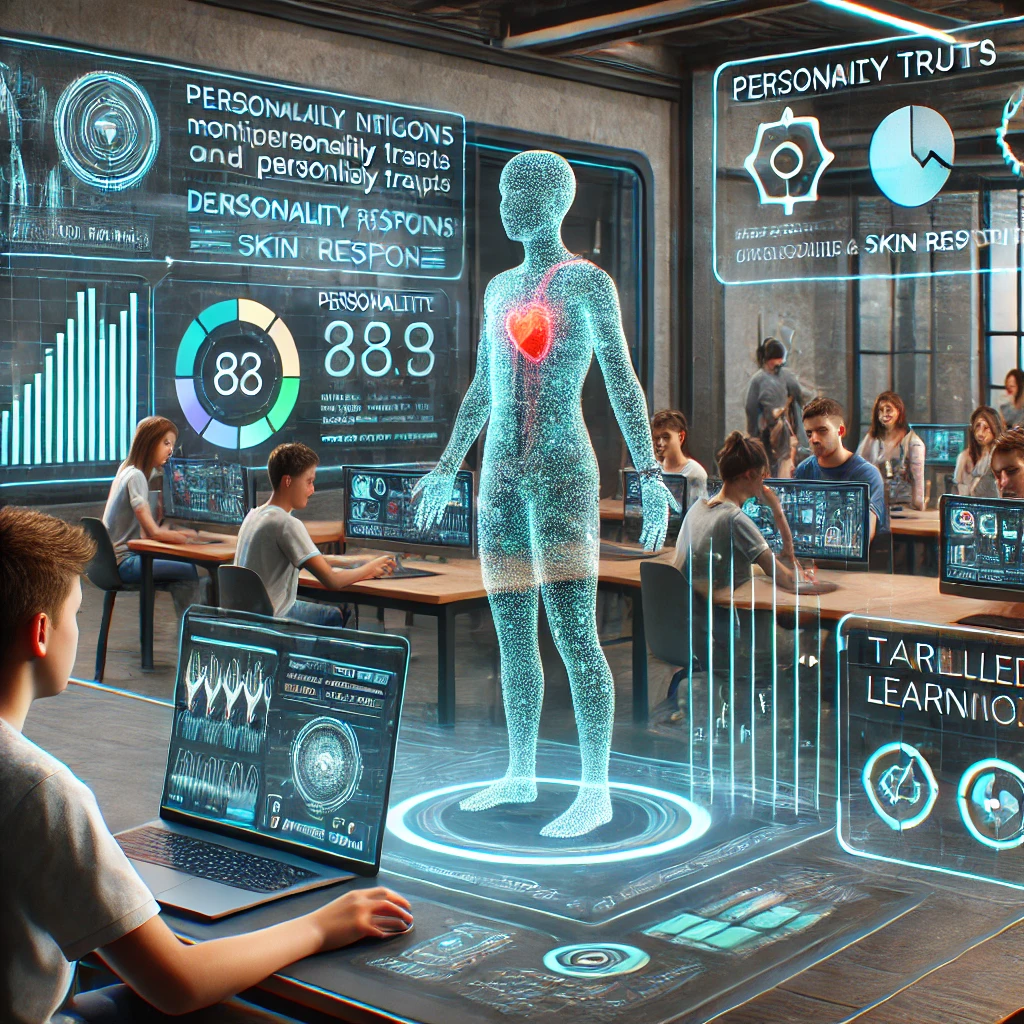
The integration of machine learning into education is transforming traditional approaches to teaching and learning. In the study “Personalized Programming Education: Using Machine Learning to Boost Learning Performance Based on Students' Personality Traits” by Chun-Hsiung Tseng and colleagues, a groundbreaking framework is introduced that leverages physiological data and machine learning to enhance learning outcomes. Available on arXiv, the research focuses on how personality traits, assessed through physiological signals such as galvanic skin response (GSR) and heart rate variance, can inform personalized learning strategies, significantly improving educational performance in programming courses.
Rethinking personality assessment in education
Traditional personality assessment methods, often based on questionnaires, suffer from limitations such as unreliable responses and subjective biases. This study proposes a more objective and data-driven alternative by utilizing physiological signals to predict personality traits. Rooted in the Big Five personality model, the research highlights how specific physiological markers can predict traits such as extraversion, agreeableness, and conscientiousness. For instance, galvanic skin response and heart rate variance were identified as critical indicators of extraversion, while heart rate variance also correlated with agreeableness and conscientiousness.
The ability to assess personality traits through physiological data represents a significant leap forward. This approach offers an automated mechanism to evaluate personality in real-time, reducing reliance on self-reported data. The findings also point to the potential of such assessments to go beyond static personality measures, providing dynamic insights into how personality traits manifest in different contexts, such as during active learning tasks.
Enhancing learning through personalization
To validate their approach, the researchers conducted an experiment with programming students enrolled in a game development course. Students were divided into two groups: an experimental group that received learning materials tailored to their personality traits and a control group with randomly assigned materials. The learning materials were aligned with three established strategies—deep learning, surface learning, and strategic learning - each designed to match different personality profiles.
The study revealed a marked difference in performance between the two groups. Students in the experimental group demonstrated a statistically significant improvement in programming skills, showcasing the effectiveness of personalized learning materials. The tailored approach fostered deeper engagement, allowing students to navigate complex programming concepts more effectively than their peers in the control group. The results provide strong evidence for the value of personalization in educational settings, especially in fields requiring high cognitive engagement like programming.
Building predictive models
The core of the study’s innovation lies in its use of machine learning to develop predictive models for personality traits. The researchers employed the Random Forest algorithm, leveraging physiological variables such as GSR and heart rate variance. These variables were processed and normalized to enhance the model’s prediction accuracy. The system successfully predicted traits like extraversion, conscientiousness, and agreeableness, demonstrating the viability of combining physiological data with advanced analytics.
Interestingly, while the model excelled in predicting some traits, it faced challenges with others, such as emotional stability and openness. This discrepancy underscores the complexity of personality as a construct and suggests avenues for future refinement. The integration of additional data sources, such as EEG signals, could improve the model’s ability to predict a broader range of traits, enhancing its utility across diverse educational scenarios.
Implications and future directions
The findings of this research hold profound implications for the future of education. By leveraging physiological data and machine learning, educators can create personalized learning environments that cater to the unique needs of each student. This approach has the potential to improve learning outcomes, increase engagement, and foster a more inclusive educational experience.
While this study focused on programming education, its methodology can be extended to other domains, offering a versatile framework for enhancing learning performance. Fields such as language learning, mathematics, and even professional training could benefit from similar personalized approaches. Additionally, integrating real-time feedback systems into these frameworks could enable continuous adaptation, making learning even more dynamic and responsive to individual needs.
However, the study also acknowledges its limitations. The relatively small sample size restricts the generalizability of the findings, and the reliance on a specific set of physiological signals may overlook other valuable indicators. Future research should focus on scaling the model to larger and more diverse populations to validate its efficacy across different educational contexts. Moreover, the inclusion of multimodal data, such as EEG or voice analytics, could enhance the model’s predictive capabilities, providing a richer understanding of the interplay between personality traits and learning behaviors.
The broader implications of this research extend beyond education. Personalized approaches informed by physiological data could revolutionize areas like workforce training, mental health interventions, and human-computer interaction. By tailoring experiences to individual profiles, this technology has the potential to improve outcomes in numerous fields.
Ultimately, this study demonstrates that combining machine learning with physiological data offers a promising path toward more effective and inclusive education. As technology continues to advance, educators and researchers alike must explore innovative ways to harness these tools, creating learning environments that are not only personalized but also adaptive, empowering, and transformative. This approach represents a significant step forward in addressing the diverse needs of learners in an increasingly complex world.
- FIRST PUBLISHED IN:
- Devdiscourse