AI Tool Transforms Chinese Braille into Text, Boosting Education for Visually Impaired Students
Researchers have developed Vision-Braille, an AI-powered tool that translates Chinese Braille images into text, significantly improving educational accessibility for visually impaired students in China. This innovative system leverages the mT5 model and RetinaNet for high-accuracy translation, aiming to bridge the educational resource gap and support students' academic aspirations.
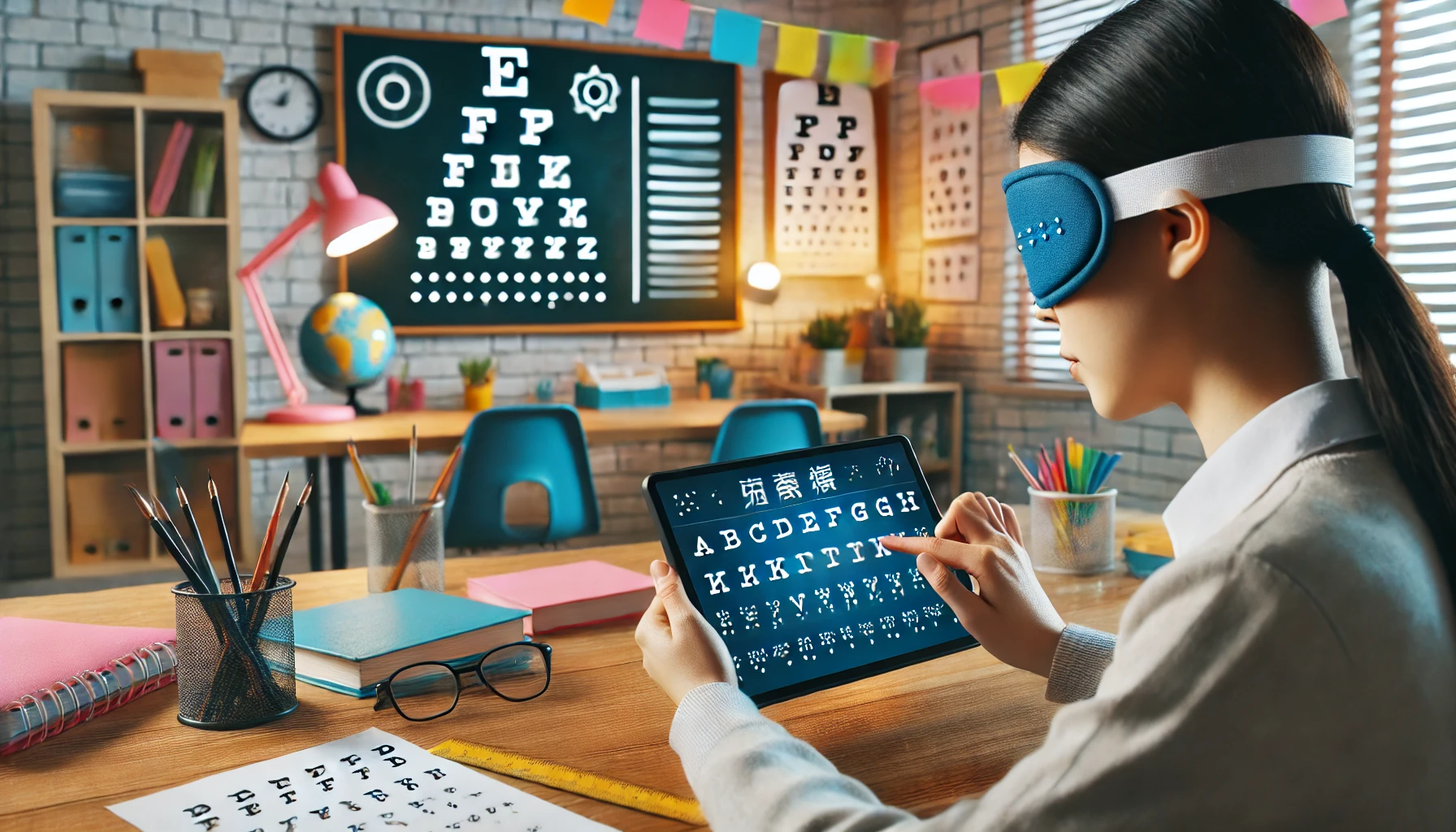
- Country:
- China
In a significant leap for the visually impaired community in China, a team of researchers has introduced Vision-Braille, an end-to-end tool that translates Chinese Braille images into text. This project, led by Alan Wu from the High School Affiliated to Renmin University of China, alongside Ye Yuan and Ming Zhang from Peking University, seeks to address the severe educational resource gap that visually impaired students face, particularly those preparing for the Chinese College Test, known as the GaoKao.
Bridging the Educational Divide
China is home to around 4 million individuals with visual disabilities, including 150,000 school-age blind children. Despite the sizable population, educational resources for these students are scarce. Only a small percentage can attend one of the few specialized high schools for blind students nationwide, resulting in a vast underrepresentation of blind students in higher education. For instance, although the Chinese Bureau of Education allows visually impaired students to take the GaoKao using Braille, only 15 students nationwide participated in this specialized exam last year. This is a stark contrast to the 13 million students who take the GaoKao annually, highlighting the immense disparity in educational opportunities due to resource limitations.
Harnessing AI for Inclusive Education
The Vision-Braille project aims to support these "double-blind" students those who are both physically disabled and at risk of illiteracy by leveraging artificial intelligence. The traditional Braille system, devised in 1824 by Louis Braille, has been adapted for Chinese, representing each character phonetically through a combination of consonants, vowels, and tones. However, tone marks are often omitted to save space, leading to confusion and inaccuracies in translation due to homophones, where different characters share the same pronunciation. This issue is further exacerbated by the lack of highly accurate Braille translation systems for Chinese. To tackle these challenges, the researchers fine-tuned the mT5 model, a multilingual variant of the T5 neural architecture, specifically for Braille to Chinese character conversion. They created a custom dataset from the Leipzig Corpora Collection, which included one million Chinese sentences converted into Braille, with various levels of tone omission to mimic real-world usage. The model was trained using a curriculum learning strategy, starting with fully toned Braille, progressing to no-tone Braille, and finally to Braille with partial tones. This approach significantly enhanced the model's ability to handle contextual information and reduced translation confusion.
Cutting-Edge Translation Technology
The Vision-Braille tool achieved impressive BLEU scores of 62.4 and 62.3 on the validation and test sets, respectively, indicating a high level of translation accuracy. The system incorporates RetinaNet for Braille Optical Character Recognition (OCR), which enables users to scan Braille images with their phones. The recognized Braille is then translated into Chinese characters, providing an accessible and practical solution for visually impaired students and educators. The development process involved several stages. Initially, the team used the Chinese-Braille-Full-Tone dataset for basic training, allowing the model to learn fundamental translation rules. Subsequently, they employed the Chinese-Braille-No-Tone dataset to train the model to infer Chinese characters based on context without tone information. Finally, they fine-tuned the model using the Chinese-Braille-10per-Tone dataset, which closely resembles real-world data distribution with 90% of the tones omitted. This comprehensive training regimen ensured that the model could accurately translate Braille to Chinese, even with significant tone omissions.
A Vision for the Future
Despite its success, the Vision-Braille tool currently handles only sentence-level translations. The researchers plan to expand its capabilities to passage-level translations and develop a user-friendly interface. They also aim to enhance the system by utilizing larger models and few-shot learning methods with advanced language models like LLaMA and GPT-4. The Vision-Braille project represents a significant step toward educational equity for visually impaired students in China. By providing a robust and accessible tool for Braille to Chinese translation, this project has the potential to transform the lives of many students, enabling them to pursue higher education and achieve their dreams. The practical applications of this tool extend beyond individual users, with potential adoption in educational institutions and real-world companies that support visually impaired individuals. The demo of Vision-Braille is available on their website, showcasing its capabilities and inviting further exploration and support from the community. This innovation not only addresses the immediate needs of visually impaired students but also sets a precedent for future advancements in assistive technologies, paving the way for more inclusive educational environments worldwide. The dedication of the research team and their collaborators underscores the importance of leveraging technology to bridge gaps in educational resources, ensuring that all students, regardless of their physical abilities, have the opportunity to succeed and thrive.
- FIRST PUBLISHED IN:
- Devdiscourse