AI meets consumer trust: A novel approach to handling negative online reviews
As consumer expectations continue to evolve, embracing explainable AI will be essential for fostering trust, enhancing customer satisfaction, and maintaining a competitive edge in the digital economy.
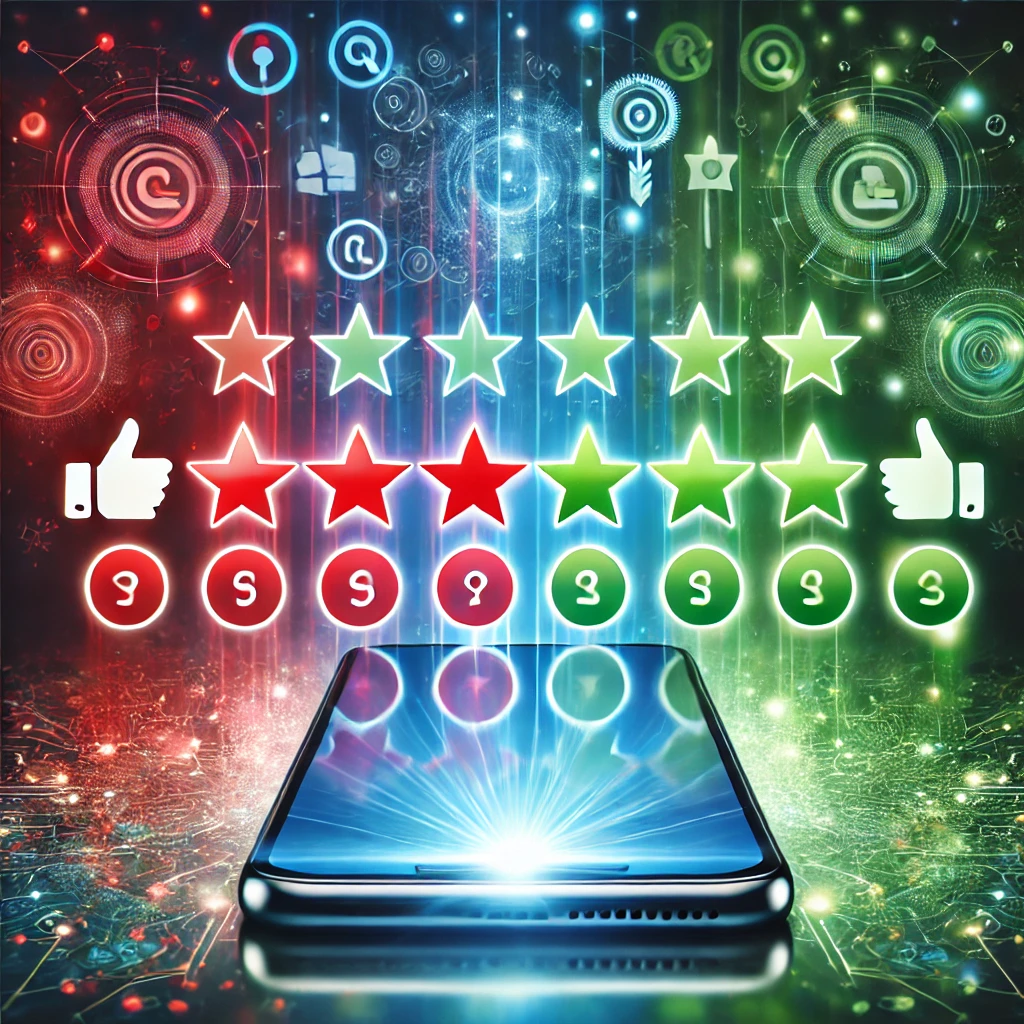
The influence of online reviews on consumer choices has grown exponentially, especially within the service sector, where product quality often relies on subjective experiences. Negative reviews, often perceived as more helpful and influential than their positive counterparts, can significantly shape a business’s reputation and bottom line. However, with the sheer volume of reviews generated daily, businesses face a daunting challenge: identifying and addressing the most critical concerns among thousands of reviews.
The study “From Prediction to Explanation: Managing Influential Negative Reviews Through Explainable AI”, available on arXiv, introduces a groundbreaking approach to tackle this issue using Explainable AI (XAI). By leveraging state-of-the-art AI techniques, the study provides actionable insights for businesses to predict and manage influential negative reviews effectively.
The growing influence of negative reviews
In an era dominated by online platforms, reviews have become pivotal in shaping consumer trust and purchasing decisions. Negative reviews, in particular, hold a unique sway as they are more frequently viewed, shared, and remembered than positive ones. They offer valuable feedback on service failures and product flaws, often resonating with other consumers who have faced similar issues. However, managing these reviews is challenging due to their emotional complexity. Multiple emotions - such as frustration, disappointment, and anger - are often intertwined, making it difficult for businesses to craft effective responses.
Furthermore, the increasing volume of reviews makes manual analysis impractical. As a result, businesses either ignore negative reviews or use generic, template-based responses that fail to address specific concerns, further exacerbating customer dissatisfaction.
Artificial intelligence has emerged as a promising tool for review analysis, excelling in tasks like sentiment analysis, spam detection, and topic extraction. However, traditional AI models, often described as "black-box systems," lack transparency in their decision-making processes. Managers using these models are left wondering why certain reviews are classified as influential and how to address the issues they highlight effectively.
The study addresses this gap by introducing an Explainable AI framework that not only predicts influential negative reviews but also provides detailed explanations at both the feature and word levels. This dual approach empowers managers to understand the underlying factors driving the classification and tailor their responses accordingly.
Methodology: From prediction to explanation
The proposed framework employs a comprehensive methodology that combines prediction and explanation to analyze and manage negative reviews effectively. It consists of three core components: a BERT-based model for review embedding, an interpretable feature attention mechanism, and a post-hoc explanation module.
The foundation of the framework is the Bidirectional Encoder Representations from Transformers (BERT) model, fine-tuned on a dataset of over 101,000 negative reviews from Dianping.com. This pre-trained model captures the nuanced semantics of review texts, enabling highly accurate classification of influential reviews.
To enhance the model’s transparency, the study integrates an interpretable feature attention mechanism. This component evaluates features such as review length, engagement level, image count, and membership status, dynamically highlighting their contributions to the classification process.
Finally, the framework incorporates post-hoc explanation techniques, specifically LIME and SHAP, to deconstruct the model’s decisions. These tools provide granular insights into the influence of individual features and words, offering a deeper understanding of the factors driving the classification and equipping managers with actionable information to address critical reviews effectively.
Key findings and predictive performance
The study compares the proposed framework against several state-of-the-art text classification algorithms, including SVM, TextCNN, LSTM, and BiLSTM. The BERT-based model consistently outperformed other methods, achieving the highest F1 score, which is particularly important for imbalanced datasets like reviews where influential reviews are a minority.
-
Feature-Level Explanations: The analysis revealed that factors like review length, engagement, anonymity, and image count significantly contribute to the classification of influential reviews. Interestingly, features such as positivity and consumption verification showed mixed impacts, suggesting complex interactions within the model.
-
Word-Level Explanations: By highlighting specific words and phrases that drive the classification, the model allows managers to pinpoint key issues. For instance, terms like "poor service," "waiter," and "disappointing" were identified as critical indicators of influential reviews.
-
Explanation-Guided Responses: Integrating the model’s predictions and explanations into response generation significantly improved the quality of managerial replies. Responses tailored to address specific issues—such as poor service or misleading promotions—were more effective in mitigating customer dissatisfaction.
Managerial Implications: Actionable Insights for Businesses
The study provides several actionable insights for businesses looking to manage negative reviews more effectively. By identifying influential reviews, managers can prioritize their efforts and focus on addressing the most critical concerns, ensuring a more efficient allocation of resources. Feature-level explanations further enhance customer engagement by providing managers with a deeper understanding of the underlying issues in customer feedback. This enables the creation of personalized and empathetic responses that resonate with dissatisfied customers, improving the overall customer experience.
Additionally, word-level explanations offer businesses the ability to pinpoint recurring issues, such as delays in service or pricing concerns, allowing for proactive measures to prevent similar complaints in the future. The transparency afforded by the Explainable AI (XAI) framework also plays a key role in building trust between businesses and their customers. When customers see their concerns being thoughtfully addressed with tailored solutions, their confidence in the brand strengthens, fostering loyalty and long-term relationships.
Limitations and future directions
While the study makes significant contributions, it is not without limitations. The dataset, focused on the restaurant industry in a single geographic region, may limit the generalizability of the findings. Future research could explore the application of the XAI framework across diverse industries and cultural contexts. Additionally, incorporating more advanced features and refining the definition of influential reviews could further enhance the model’s accuracy and applicability.
As consumer expectations continue to evolve, embracing explainable AI will be essential for fostering trust, enhancing customer satisfaction, and maintaining a competitive edge in the digital economy.
- FIRST PUBLISHED IN:
- Devdiscourse