The Future of E-commerce: Leveraging Big Data for Efficient Consumer Analysis
The paper explores how integrating Big Data with business intelligence systems enhances e-commerce data analysis, offering deeper insights into consumer behavior through the Days-Times-Money (DTM) model. This approach helps businesses tailor marketing strategies, improving decision-making and competitiveness.
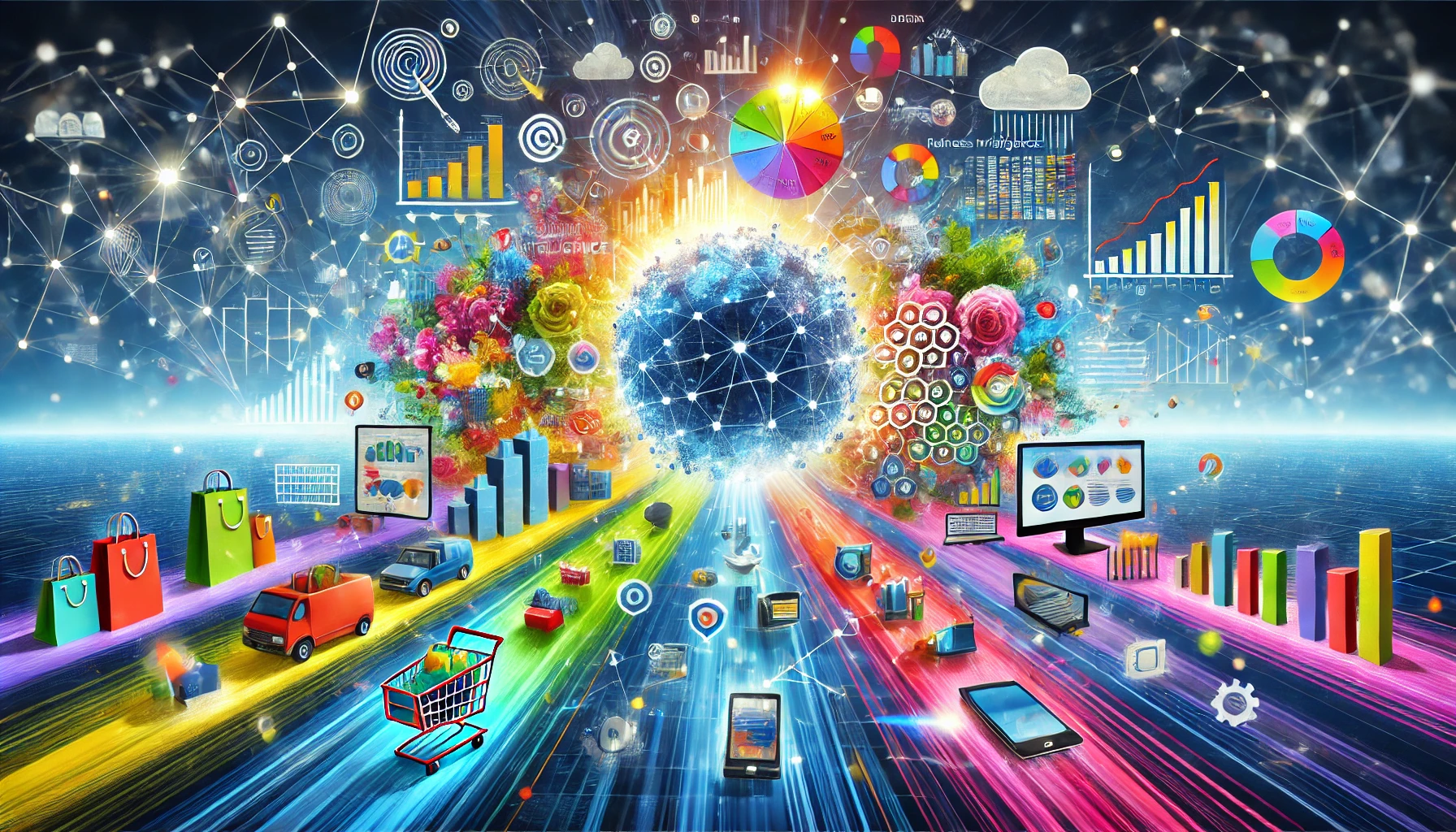
Researchers from Yunnan Technology and Business University, SEGi University, and Dazhou Vocational and Technical College, examine the application of Big Data-driven business intelligence in e-commerce. With the rapid expansion of e-commerce, the sheer volume and complexity of consumer data have outgrown the capabilities of traditional business intelligence systems, necessitating more advanced models for data analysis. The research explores how the integration of Big Data with business intelligence systems can improve data processing efficiency and provide deeper insights into consumer behavior, which is essential for businesses to stay competitive in the evolving digital marketplace. The researchers propose the Days-Times-Money (DTM) model as a tool to better understand consumer habits and preferences, allowing businesses to make informed decisions based on comprehensive data analysis.
Challenges of Traditional Business Intelligence Systems
In this study, the authors argue that traditional business intelligence systems struggle to manage the complexity and volume of modern e-commerce data. These systems often produce complex data reports that are difficult to interpret, especially for decision-makers outside the technical domain. Moreover, they lack the ability to interactively analyze data across multiple dimensions, making it hard to extract meaningful insights. The paper highlights that business intelligence systems need to move beyond their conventional roles by incorporating advanced data mining technologies that can uncover hidden patterns and provide more actionable intelligence. To address this gap, the researchers combine Big Data and business intelligence to develop the DTM model, which was tested on the consumer data of a particular beverage brand.
The Days-Times-Money (DTM) Model for Consumer Insights
The DTM model categorizes consumers based on three main factors: the number of days they make purchases, the frequency of their purchases, and the total amount of money spent. The researchers used data mining techniques to process and analyze consumer data, which was then classified into four distinct consumer groups based on these consumption attributes. The results revealed that the highest consumption density for days, times, and money was 68.63%, 67.99%, and 69.72%, respectively, indicating significant room for growth in consumer engagement for the brand. By classifying consumers into groups such as high-frequency and high-amount buyers, or low-frequency and low-amount buyers, the researchers provided insights that could help the brand tailor its marketing strategies to different consumer segments. For example, high-frequency but low-amount buyers could be incentivized with discount coupons to increase their spending, while high-frequency and high-amount consumers could be targeted with personalized product recommendations to maintain their loyalty.
Big Data Enhancing Business Intelligence Capabilities
The study also underscores the importance of using Big Data to enhance the analytical power of business intelligence systems. By employing data mining techniques, businesses can go beyond superficial metrics like sales volume and profit margins to uncover more nuanced patterns in consumer behavior. This ability to dig deeper into consumer data allows businesses to personalize their marketing efforts and develop strategies that cater to the specific needs and preferences of different consumer groups. The authors point out that the DTM model can provide more accurate assessments of customer value by analyzing not just how much they spend, but how frequently they make purchases and how engaged they are with the brand over time. The comprehensive data analysis provided by this model can help businesses make more informed decisions, such as when to introduce new products, how to adjust pricing strategies, and how to optimize customer retention efforts.
Acknowledging the Limitations and Future Challenges
While the study demonstrates the potential benefits of integrating Big Data with business intelligence in e-commerce, it also acknowledges certain limitations. The research was based on data from a single beverage brand, which limits the generalizability of the findings. Furthermore, the authors highlight the ongoing challenges of data security and privacy in the Big Data era, issues that businesses must address as they continue to rely more heavily on data-driven decision-making. Despite these challenges, the paper argues that the adoption of Big Data analytics in business intelligence systems is an inevitable trend in the e-commerce sector. As businesses increasingly rely on digital platforms to interact with customers and drive sales, the ability to effectively analyze large datasets will be critical to maintaining competitiveness in the marketplace.
A Strong Case for Big Data in E-commerce
The research provides valuable insights into how the integration of Big Data and business intelligence systems can enhance e-commerce data analysis and improve decision-making processes. By developing the DTM model, the researchers offer a practical tool for classifying consumers and optimizing marketing strategies based on detailed consumption patterns. The findings suggest that businesses can significantly benefit from incorporating Big Data into their business intelligence systems to better understand customer behavior, increase engagement, and boost profitability. While challenges remain, particularly in terms of data security and the scalability of the research to other product categories, the study lays a strong foundation for further exploration of Big Data applications in e-commerce.
- FIRST PUBLISHED IN:
- Devdiscourse