ChatGPT and Stock Forecasts: Examining AI’s Behavioral Biases in Financial Markets
The study examines how ChatGPT-4 interprets historical stock returns and finds that, like humans, it over-extrapolates recent trends and exhibits optimism about future performance. While its risk assessments are more calibrated, it still displays biases in stock return forecasting.
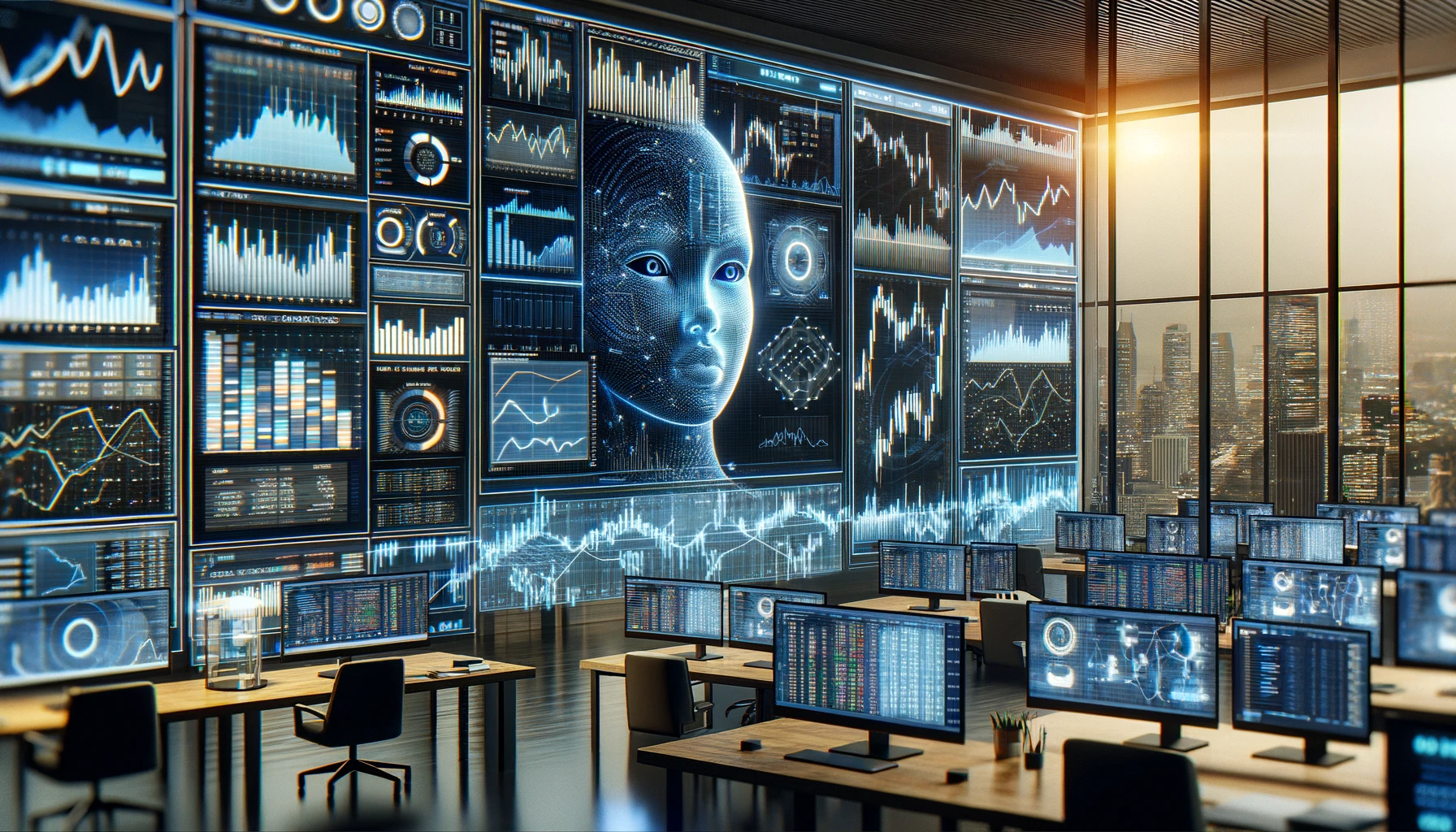
A comprehensive study by Shuaiyu Chen, T. Clifton Green, Huseyin Gulen, and Dexin Zhou from Purdue University, Emory University, and Baruch College, examines how large language models (LLMs) like ChatGPT-4 handle stock return data and whether they exhibit human-like biases in their forecasts. The researchers explore how ChatGPT interprets historical stock returns and compares its predictions with crowd-sourced forecasts from the Forcerank platform, a system where participants rank stocks based on expected performance. Their findings indicate that LLMs, like human forecasters, tend to over-extrapolate recent trends and exhibit optimism about future returns, though they are somewhat better calibrated in assessing risks than humans.
The Extrapolation Bias in Stock Forecasts
The study’s core investigation centers on whether ChatGPT displays cognitive biases similar to those well-documented in human investors, such as over-extrapolation of recent returns. Over-extrapolation occurs when investors place too much weight on recent performance, assuming that trends will continue, even when historical data show short-term reversals are common. In financial markets, returns tend to reverse in the short term, meaning assets that have recently performed well often perform worse in the near future and vice versa. The researchers sought to understand if ChatGPT would follow this trend or, like humans, rely heavily on recent data, thus overestimating the likelihood of continued positive returns.
ChatGPT’s Predictions in Stock Ranking Contests
To explore this, the team prompted ChatGPT-4 to participate in stock-ranking contests similar to those held on the Forcerank platform. Participants in these contests rank ten stocks each week based on their perceived future performance, with the rankings influenced by twelve weeks of historical return data. The research team provided ChatGPT with the same historical data to see how its predictions aligned with human forecasts and real-world returns. The results showed that, much like human participants, ChatGPT placed significant emphasis on recent stock performance, especially the previous week's returns. However, while humans reacted more strongly to negative returns, ChatGPT's forecasts were influenced more by positive returns in the recent past. This tendency suggests that, like humans, ChatGPT over-extrapolates but does so with a greater focus on positive performance.
Optimistic Forecasting and Risk Calibration
One notable finding is that despite LLMs being trained to handle large datasets objectively, ChatGPT’s forecasts displayed optimism relative to both historical averages and realized future returns. On average, the forecasts generated by ChatGPT were higher than the historical returns it was given, and the model predicted next-period returns to be significantly higher than what materialized. For example, while the average realized return in the dataset was around 1.15%, ChatGPT’s average forecast was closer to 2.2%. This suggests that the model may have been influenced by its training data, which likely embedded an assumption that future returns should generally be positive, leading to an optimistic bias.
Improved Confidence Intervals but Pessimistic Extremes
The researchers also assessed how well ChatGPT handled risk estimation by comparing its performance in predicting confidence intervals with human forecasts. When prompted to provide 80% confidence intervals (the range within which the model predicts returns will fall 80% of the time), ChatGPT performed better than human CFOs surveyed in earlier studies, particularly in its calibration. However, even though the model’s confidence intervals were more accurate than human forecasts, ChatGPT still demonstrated a bias toward conservatism at the extremes. Its forecasts for the 10th and 90th percentiles were lower than the actual historical data, indicating that the model was more pessimistic about both extreme positive and negative outcomes than it should have been based on the data.
Visual Data and Cross-Model Comparison
In another aspect of the study, the researchers provided ChatGPT with visual data in the form of price charts to see if the model’s forecasting behavior would change when analyzing visual rather than numerical data. ChatGPT performed similarly in this task, extrapolating from recent performance and continuing to overemphasize short-term trends. This suggests that its tendency to over-extrapolate is not limited to numerical data but also extends to visual financial information.
Furthermore, the study compared ChatGPT’s forecasts to those of another large language model, Claude, to see if these biases were specific to ChatGPT or more broadly embedded in LLMs. The results showed that Claude exhibited similar behavior, with a high correlation between the two models' forecasts. This indicates that the observed biases, such as over-extrapolation of recent returns and optimism about future performance, are not unique to ChatGPT but may be common across different LLMs.
In summary, while ChatGPT’s forecasts were more calibrated than human predictions, especially in terms of risk assessment, the model still demonstrated significant cognitive biases. The findings suggest that even though LLMs offer enhanced numeracy and risk assessment capabilities compared to humans, they remain vulnerable to the same biases that affect human decision-making in financial contexts. As LLMs continue to be integrated into financial decision-making processes, it will be crucial to critically evaluate their forecasts and account for these potential biases to avoid over-reliance on their predictions.
- READ MORE ON:
- large language models
- ChatGPT-4
- ChatGPT
- LLMs
- FIRST PUBLISHED IN:
- Devdiscourse