Harnessing Real-Time Data for Effective Welfare Monitoring and Policy Decisions
The World Bank's report highlights the importance of real-time welfare monitoring using advanced methods like nowcasting and microsimulations to provide timely data for effective policymaking. By leveraging both traditional and high-frequency data, governments can make informed decisions to address poverty and vulnerability more efficiently.
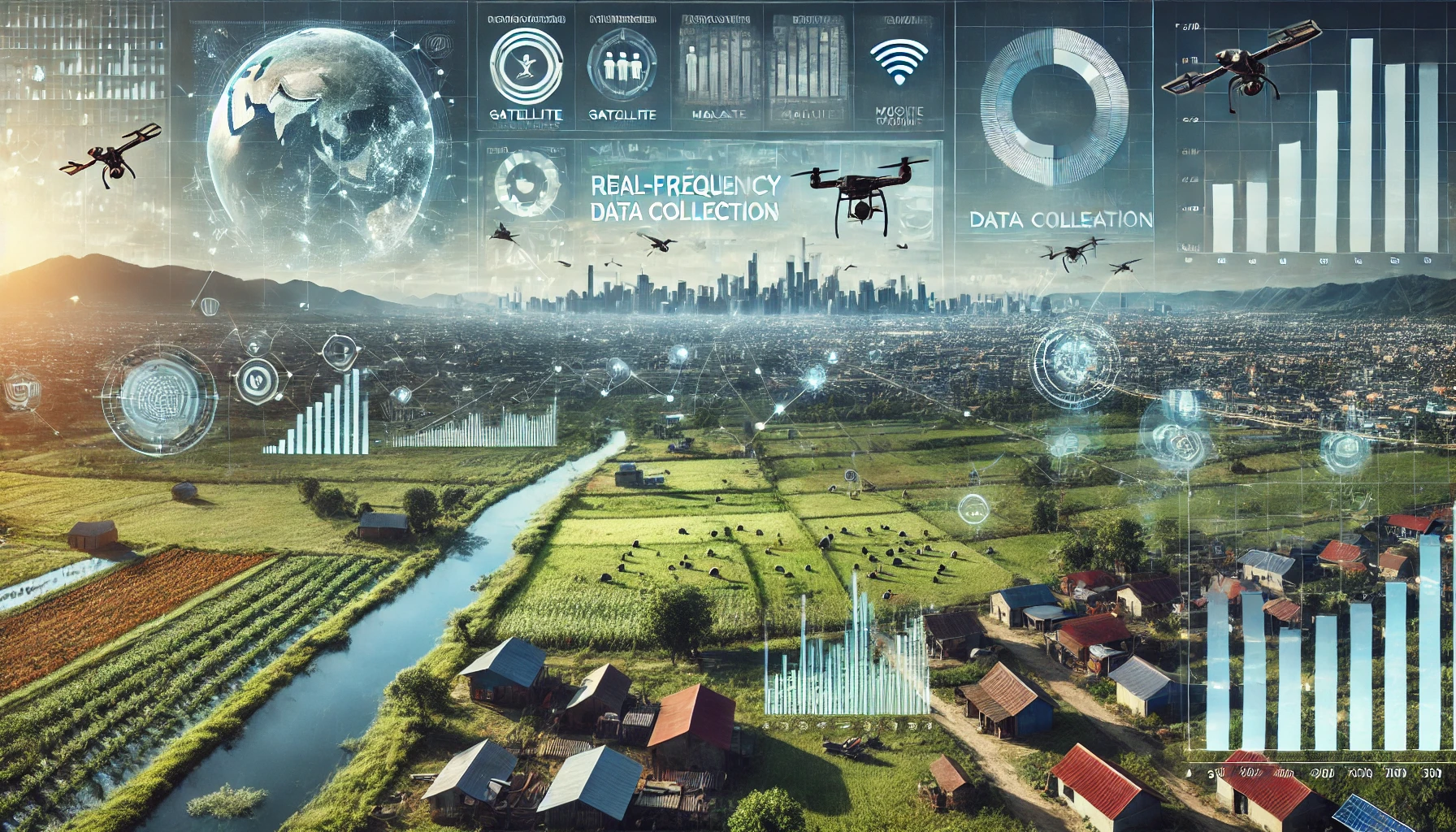
The World Bank's document explores the critical role that timely welfare data plays in shaping effective policymaking, particularly in addressing poverty and vulnerability in the context of global crises such as pandemics, climate change, and economic shocks. Policymakers traditionally rely on household surveys to assess welfare and poverty levels, but these methods are often outdated by the time they are used. Surveys are typically conducted only every few years due to high financial and administrative costs. In low-income and fragile countries, these gaps are even larger, making it difficult for governments to respond effectively to current challenges. This document highlights the importance of real-time welfare monitoring as a way to address this lag in data and improve policy decisions.
New Approaches to Welfare Monitoring for Better Decision-Making
To meet the need for more timely data, the World Bank's Poverty and Equity Global Practice has been at the forefront of developing and implementing new approaches to welfare monitoring. These methods leverage both traditional surveys, referred to as baseline data, and alternative, high-frequency data sources, such as geospatial data, digital trace data, and administrative records. These new data sources allow for real-time monitoring of welfare trends, offering policymakers insights into household well-being on a much more frequent basis than traditional surveys. The document outlines various methods, including nowcasting, GDP-based welfare monitoring, and microsimulations, which help update welfare estimates by using auxiliary data. Nowcasting involves using models that combine baseline data with other frequent data points, such as GDP growth or satellite imagery, to estimate current welfare conditions. This method has grown in prominence as policymakers recognize that they need to respond faster and more effectively to economic shocks or environmental disasters.
The Role of Microsimulations in Real-Time Welfare Analysis
The report also explores the use of microsimulations and general equilibrium models as another approach to real-time welfare monitoring. These methods draw on richer data sources, allowing for a more detailed analysis of how macroeconomic changes impact different segments of the population. Microsimulations can model the behavior of households and individuals and how these are affected by factors like changes in prices, wages, or employment. Such methods are particularly useful in scenarios where the effects of shocks are not evenly distributed across populations, making it necessary to account for indirect effects and distributional changes. For instance, the impacts of an economic downturn might be felt more acutely in rural areas than in urban centers, or among unskilled laborers as opposed to professionals. Microsimulations provide a more nuanced understanding of these dynamics, which can lead to more targeted and effective policy interventions.
GDP-Based Models for Welfare Nowcasting
GDP-based models also play a significant role in nowcasting welfare. These models assume that economic growth, reflected in GDP, correlates strongly with poverty reduction. By updating poverty estimates based on changes in GDP, these methods offer a simpler way to monitor welfare. However, the document points out that this approach may not capture the complexity of how economic growth impacts different parts of the population. For example, growth in unskilled labor-intensive sectors like agriculture or construction tends to have a greater effect on poverty reduction than growth in capital-intensive sectors. Therefore, while GDP-based models are useful for providing a broad overview, they may need to be supplemented with more detailed methods, such as microsimulations, to account for the distributional impacts of growth.
Challenges in Real-Time Welfare Monitoring
One of the key challenges in real-time welfare monitoring is the availability and quality of data. In many low-income countries, both survey and auxiliary data are either unavailable or unreliable, which limits the effectiveness of these new methods. The document emphasizes the importance of continued investment in baseline data collection, such as household surveys and censuses, as these provide the foundation for all real-time monitoring efforts. Even with advanced nowcasting and microsimulation models, without a strong baseline, the estimates produced may not be reliable. The World Bank has been working closely with national governments to modernize their statistical systems, but there is still a significant gap in the availability of up-to-date welfare data in many parts of the world.
Balancing Simplicity and Complexity in Welfare Monitoring
The report also acknowledges that all of these methods come with trade-offs. The most appropriate method for monitoring welfare in real time depends on the specific context of a country, its data ecosystem, and the objectives of the analysis. In some cases, simpler methods like GDP-based nowcasting might be sufficient, while in others, more complex models that account for distributional shifts might be necessary. The typology of approaches presented in the document provides a structured way for policymakers to evaluate different methods and select the one that best fits their needs. Ultimately, improving real-time welfare monitoring is essential for governments to respond more effectively to crises and improve the resilience of their economies and populations. By using these advanced monitoring tools, policymakers can ensure that their interventions are data-driven and responsive to current conditions, making them more likely to achieve meaningful reductions in poverty and inequality.
- FIRST PUBLISHED IN:
- Devdiscourse